Evidential Pseudo-Label Ensemble for semi-supervised classification
PATTERN RECOGNITION LETTERS(2024)
摘要
Semi-supervised learning (SSL) focuses on effectively exploiting both a limited number of labeled data and relatively rich unlabeled data. Recent SSL researches have achieved great progress with pseudo-labeling. However, incorrect pseudo-label may cause severe error propagation, which has been widely recognized and termed as confirmation bias. Furthermore, vanilla ensemble strategies aiming at alleviating this issue will lead to serious conservative labeling due to the averaging property. Accordingly, they could not sufficiently explore the unlabeled data. In this paper, we proposed a novel SSL method termed Evidential Pseudo-Label Ensemble (EPLE) which aims to generate more accurate pseudo-labels with evidence support. Specifically, multiple networks with different augmented strategies are introduced to generate complementary evidence for unlabeled samples, and induce confident predictions with Dempster-Shafer theory (DST), which effectively addresses error propagation and confirmation bias. To enhance the consistency of predictions for the same samples with different noises, we employ multiple augmentation strategies for both weak and strong augmentations. We conduct experiments on standard semi-supervised learning datasets, including CIFAR-10/100, SVHN and STL10. Extensive experiments show the superior performance of our proposed EPLE compared to state-of-the-art SSL models.
更多查看译文
关键词
Semi-supervised learning,Pseudo labeling,Dempster-Shafer theory
AI 理解论文
溯源树
样例
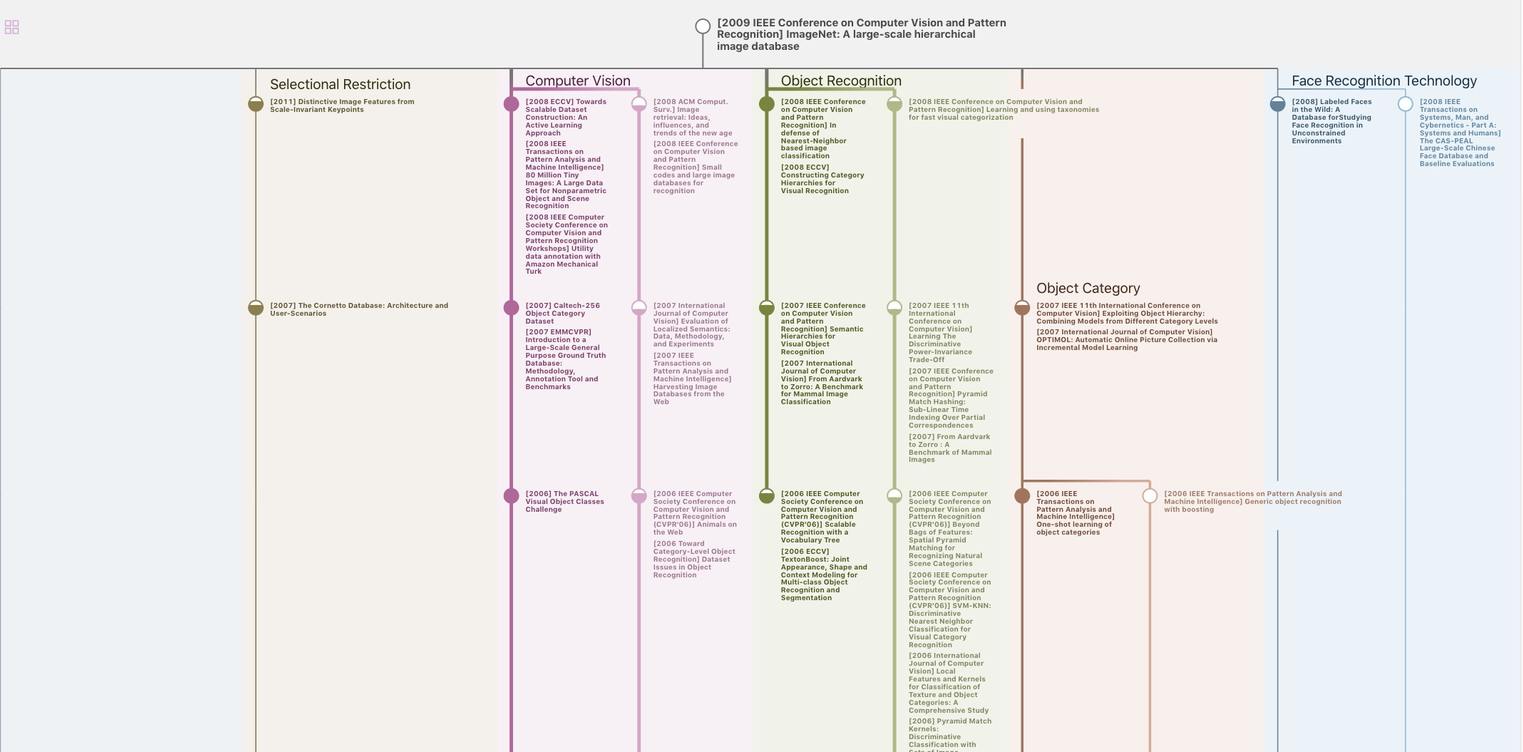
生成溯源树,研究论文发展脉络
Chat Paper
正在生成论文摘要