Hierarchical Classification of Financial Transactions Through Context-Fusion of Transformer-based Embeddings and Taxonomy-aware Attention Layer
CoRR(2023)
摘要
This work proposes the Two-headed DragoNet, a Transformer-based model for
hierarchical multi-label classification of financial transactions. Our model is
based on a stack of Transformers encoder layers that generate contextual
embeddings from two short textual descriptors (merchant name and business
activity), followed by a Context Fusion layer and two output heads that
classify transactions according to a hierarchical two-level taxonomy (macro and
micro categories). Finally, our proposed Taxonomy-aware Attention Layer
corrects predictions that break categorical hierarchy rules defined in the
given taxonomy. Our proposal outperforms classical machine learning methods in
experiments of macro-category classification by achieving an F1-score of 93\%
on a card dataset and 95% on a current account dataset.
更多查看译文
AI 理解论文
溯源树
样例
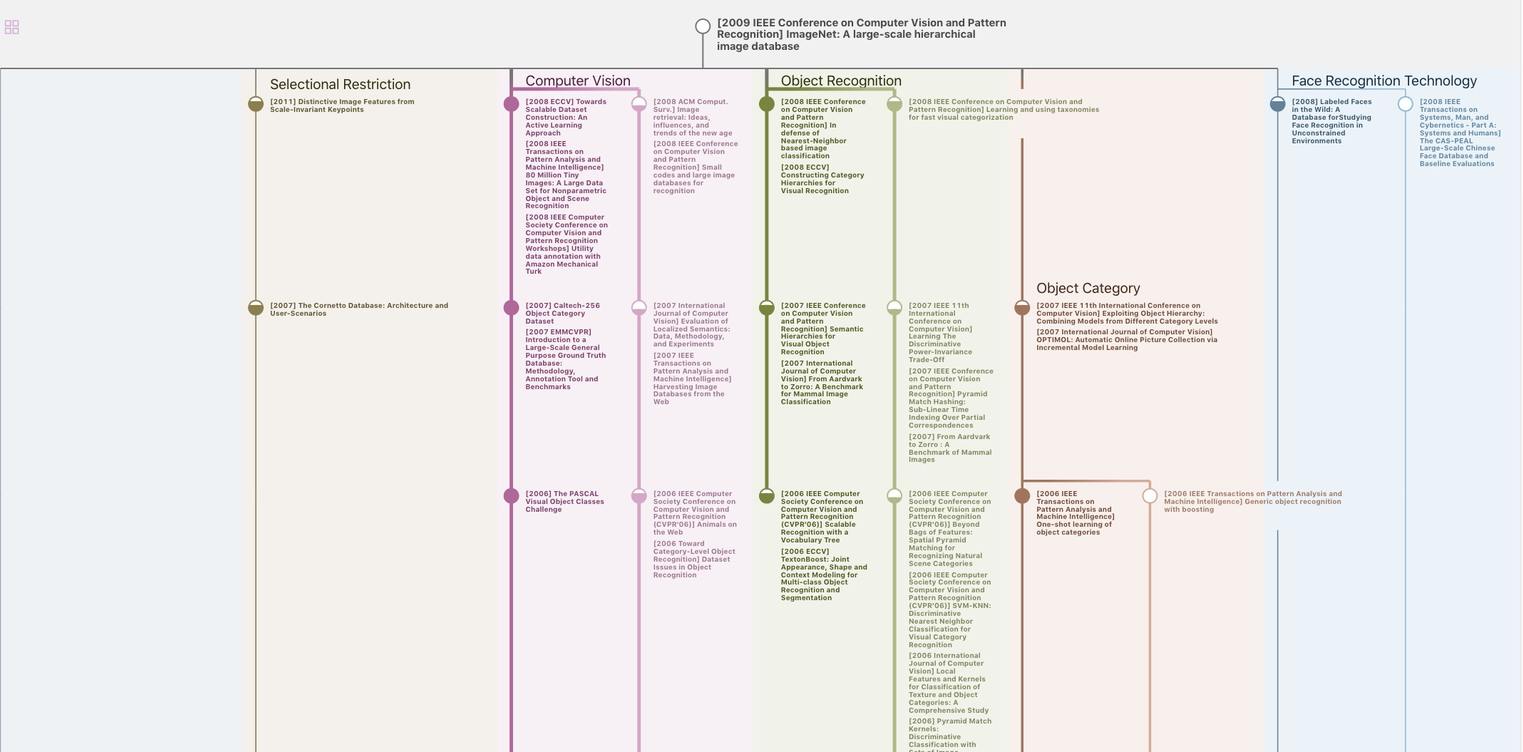
生成溯源树,研究论文发展脉络
Chat Paper
正在生成论文摘要