Differentially Private Gradient Flow based on the Sliced Wasserstein Distance for Non-Parametric Generative Modeling
CoRR(2023)
摘要
Safeguarding privacy in sensitive training data is paramount, particularly in
the context of generative modeling. This is done through either differentially
private stochastic gradient descent, or with a differentially private metric
for training models or generators. In this paper, we introduce a novel
differentially private generative modeling approach based on parameter-free
gradient flows in the space of probability measures. The proposed algorithm is
a new discretized flow which operates through a particle scheme, utilizing
drift derived from the sliced Wasserstein distance and computed in a private
manner. Our experiments show that compared to a generator-based model, our
proposed model can generate higher-fidelity data at a low privacy budget,
offering a viable alternative to generator-based approaches.
更多查看译文
AI 理解论文
溯源树
样例
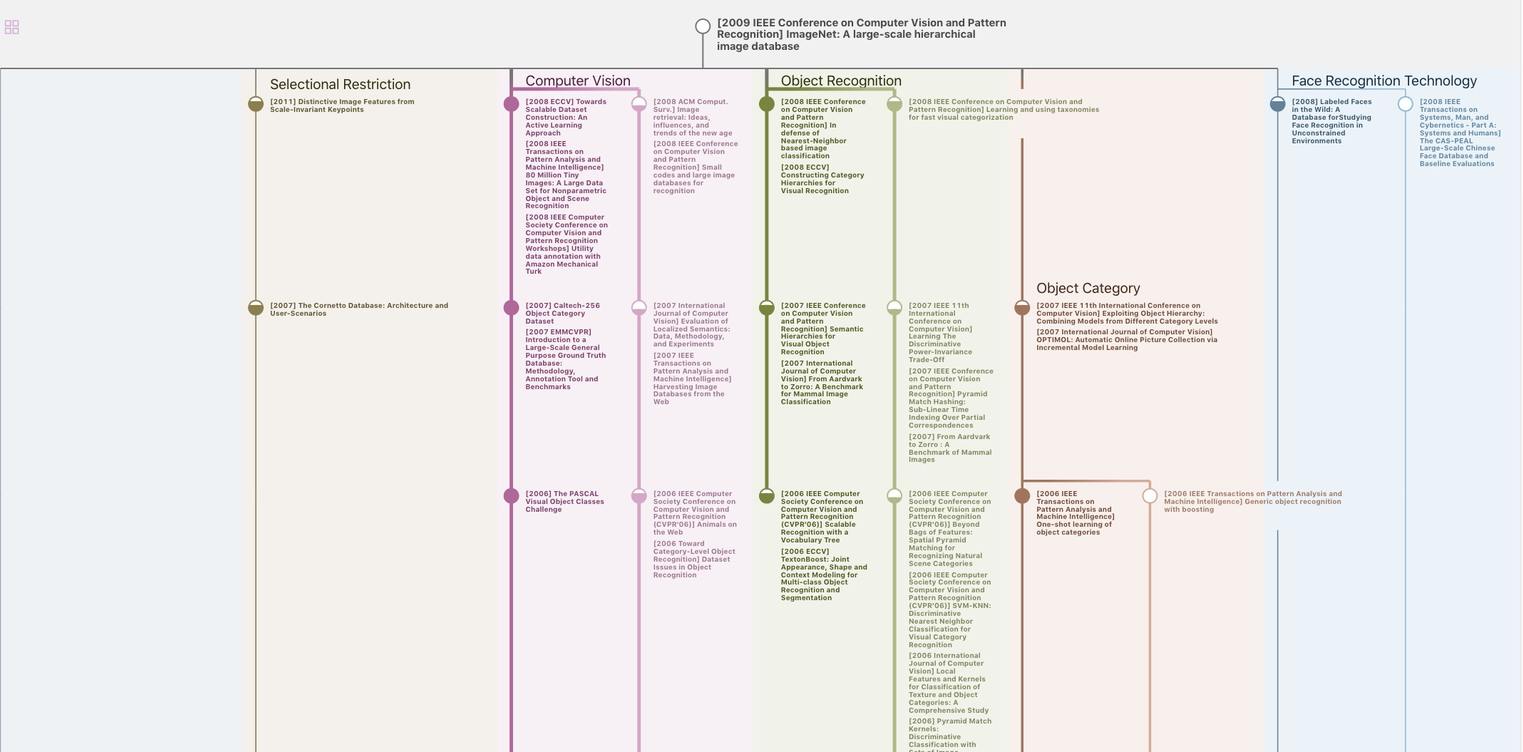
生成溯源树,研究论文发展脉络
Chat Paper
正在生成论文摘要