Causal Covariate Selection for the Imputation-based Regression Calibration Method for Exposure Measurement Error Bias Correction
arxiv(2023)
摘要
In this paper, we identify the criteria for the selection of the minimal and
most efficient covariate adjustment sets for the regression calibration method
developed by Carroll, Rupert and Stefanski (CRS, 1992), used to correct bias
due to continuous exposure measurement error. We utilize directed acyclic
graphs to illustrate how subject matter knowledge can aid in the selection of
such adjustment sets. Valid measurement error correction requires the
collection of data on any (1) common causes of true exposure and outcome and
(2) common causes of measurement error and outcome, in both the main study and
validation study. For the CRS regression calibration method to be valid,
researchers need to minimally adjust for covariate set (1) in both the
measurement error model (MEM) and the outcome model and adjust for covariate
set (2) at least in the MEM. In practice, we recommend including the minimal
covariate adjustment set in both the MEM and the outcome model. In contrast
with the regression calibration method developed by Rosner, Spiegelman and
Willet, it is valid and more efficient to adjust for correlates of the true
exposure or of measurement error that are not risk factors in the MEM only
under CRS method. We applied the proposed covariate selection approach to the
Health Professional Follow-up Study, examining the effect of fiber intake on
cardiovascular incidence. In this study, we demonstrated potential issues with
a data-driven approach to building the MEM that is agnostic to the structural
assumptions. We extend the originally proposed estimators to settings where
effect modification by a covariate is allowed. Finally, we caution against the
use of the regression calibration method to calibrate the true nutrition intake
using biomarkers.
更多查看译文
AI 理解论文
溯源树
样例
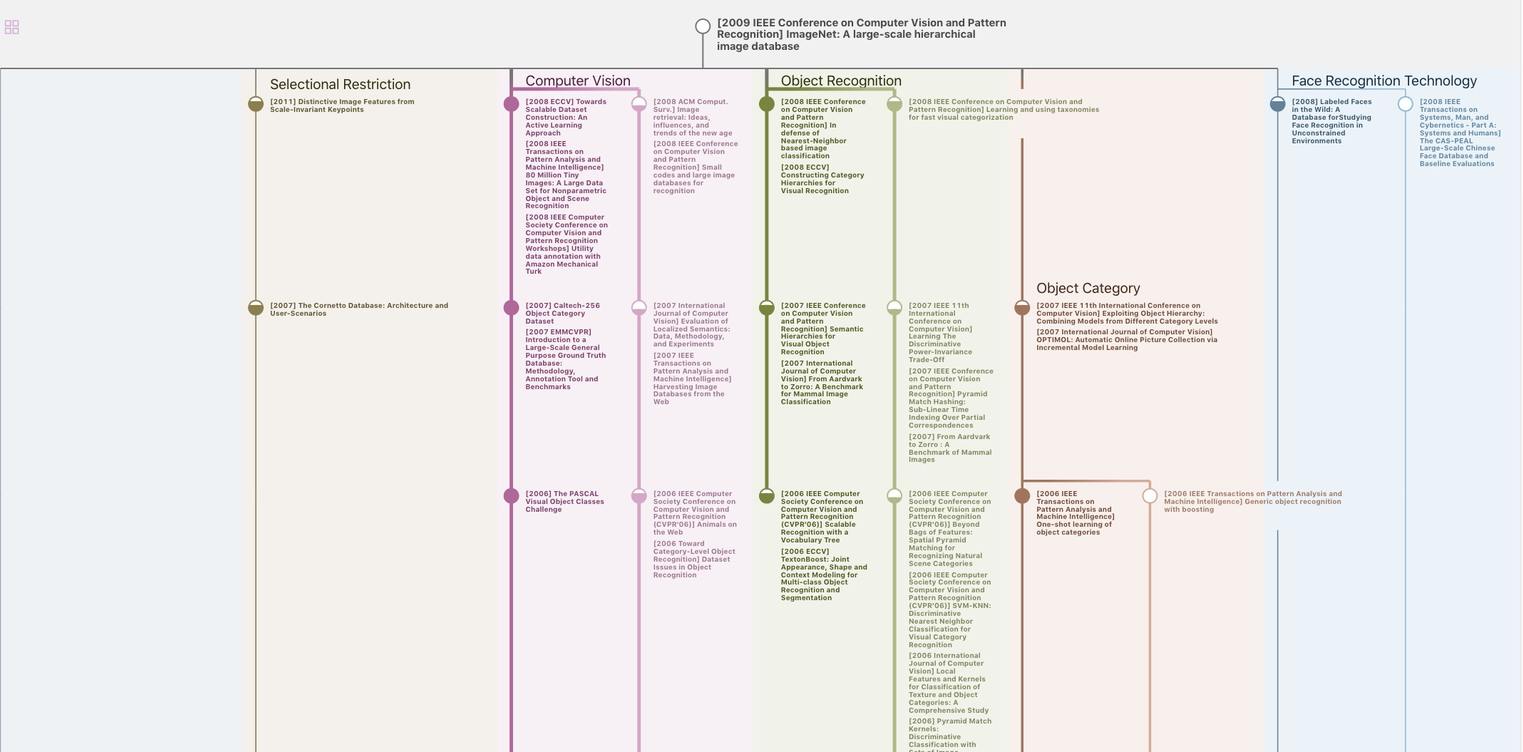
生成溯源树,研究论文发展脉络
Chat Paper
正在生成论文摘要