Improved YOLOv7 Based on Small Target Information Extraction for Road Crack Detection
2023 2nd International Conference on Machine Learning, Cloud Computing and Intelligent Mining (MLCCIM)(2023)
摘要
As one of the end-to-end detection algorithms in the domain of object detection, YOLO uses a separate convolution neural network (CNN) to achieve feature extraction and prediction. With higher efficiency and better performance, YOLOv7 is widely used to detect road damages, especially the cracks. But our experiments indicate that YOLOv7 is not good enough for small cracks detection, and we improve the detection performance of YOLOv7 by adding a layer for small targets information extraction to reduce the loss of feature information during the convolution process. Furthermore, in order to obtain richer feature information, we use RepConv and MPConv instead of simple convolution operations to ensure that small cracks can retain key feature information after fusing features from different scales and channels. Experiments are carried out on an open road damage sub-data set, and the results demonstrate that the detection performance of small road cracks and damages has been improved. At the same time, compared with the basic YOLOv7, the mAP@0.5 of our method has been increased by 2.5%. Moreover, as we can see from the visualization results, the detection performance of our improved YOLOv7 for small road cracks detection is also better than other YOLOv7-based models.
更多查看译文
关键词
road inspection,road crack detection,YOLOv7,small object detection
AI 理解论文
溯源树
样例
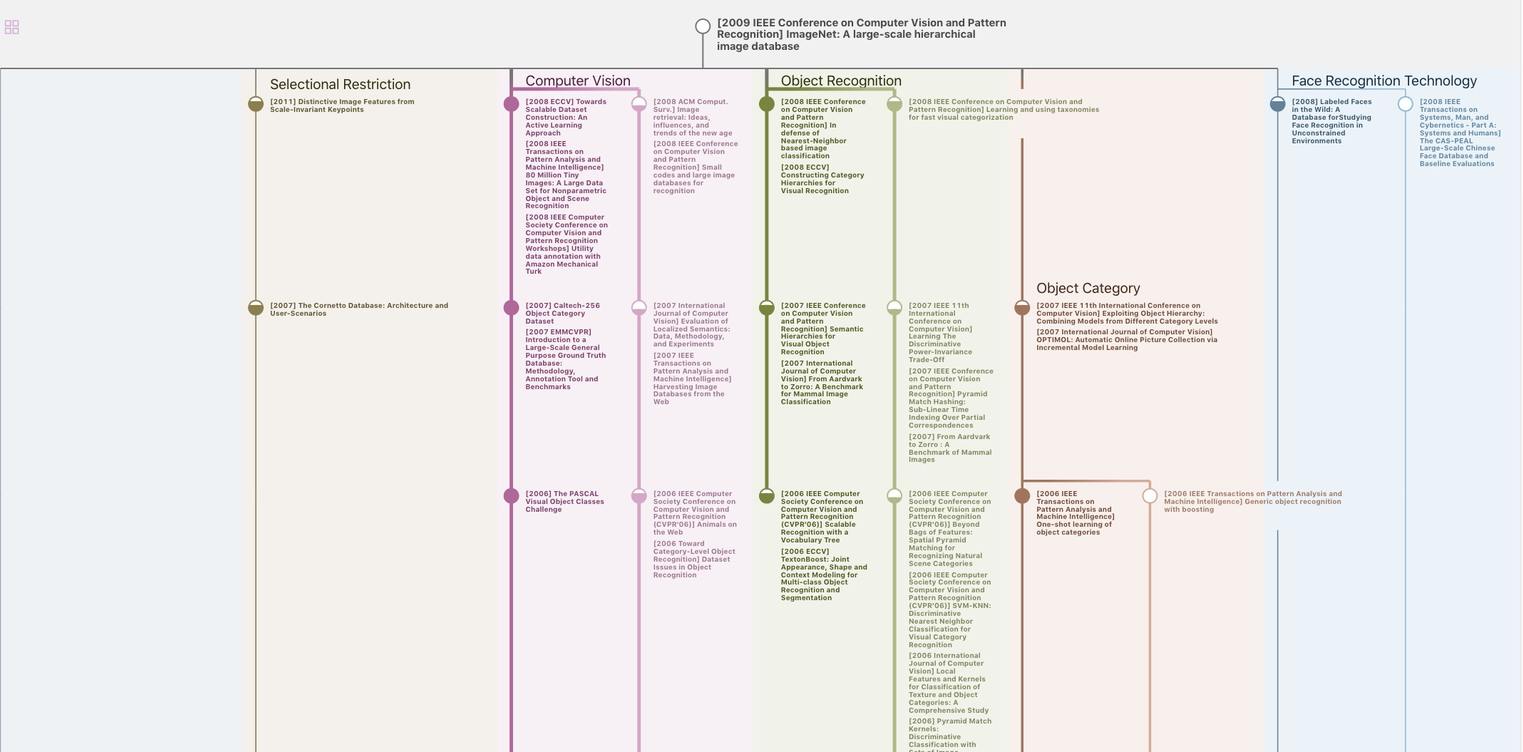
生成溯源树,研究论文发展脉络
Chat Paper
正在生成论文摘要