MA-TDMPC: Multi-Agent Temporal Difference for Model Predictive Control
2023 2nd International Conference on Machine Learning, Cloud Computing and Intelligent Mining (MLCCIM)(2023)
摘要
Model-based reinforcement learning has achieved substantial progress in recent years. However, it still faces challenges when applied in multi-agent systems. These challenges in multi-agent environments include the curse of dimensionality, partial observability, which pose difficulties in environment modeling. To address these problems, a novel model-based multi-agent reinforcement learning algorithm, named multi-agent temporal difference for model predictive control (MA-TDMPC), is proposed in this paper. In MA-TDMPC, each agent maintains its own multi-agent environment model: multi-agent communication-based local environment model (MACLM). MACLM is built on the local state-action space of each agent, thus reducing modeling complexity. To overcome the limitation of partial observability in multi-agent environment, MACLM makes prediction based on multi-agent communication. In terms of model utilization, MA-TDMPC uses the MACLM for action trajectory optimization within a finite horizon. Terminal value functions learned by temporal difference are used to estimate long term returns of trajectories, which is combined with the environment model for trajectory value estimation. Our method, MA-TDMPC, outperforms model-free multi-agent reinforcement learning and prior TDMPC in terms of superior sample efficiency and asymptotic performance on MPE tasks.
更多查看译文
关键词
model-based reinforcement learning,multi-agent reinforcement learning,temporal difference learning,model predictive control
AI 理解论文
溯源树
样例
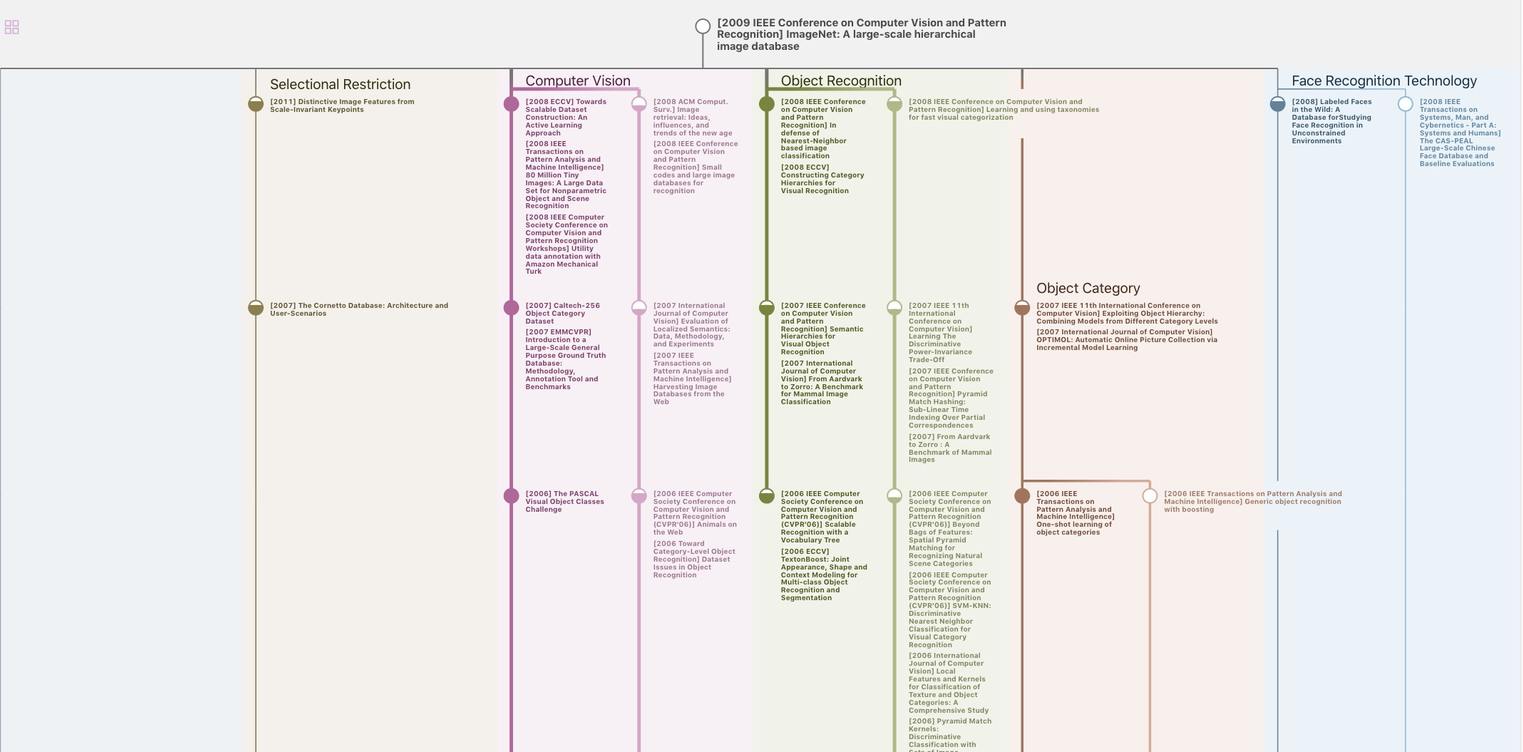
生成溯源树,研究论文发展脉络
Chat Paper
正在生成论文摘要