A Cooperative Silicon Content Dynamic Prediction Method With Variable Time Delay Estimation in the Blast Furnace Ironmaking Process
IEEE TRANSACTIONS ON INDUSTRIAL INFORMATICS(2024)
摘要
Online assessment of the molten iron quality of a blast furnace ironmaking process strongly depends on reliable measurement of silicon (Si) content. In this article, we propose a novel cooperative strategy to train the data-driven prediction model and estimate variable time delay (VTD) values. First, a dynamic deep network based on stacked denoising autoencoders (D-SDAE) with the ability to describe process nonlinearity and time-varying is designed for Si estimation. Then, time delay between the process variables and Si, which may disrupt the data distribution pattern (i.e., the real input-output relationship), is recovered in the modeling process. It is an overlooked data feature that occurs due to material transportation times, installation location, and the analysis period of sampling equipment. The VTD values are considered to be the parameters of the D-SDAE model and obtained in the training process by the proposed double-scale collaborative search particle swarm optimization algorithm. The effectiveness of the proposed VTD-based D-SDAE model is validated in a numerical simulation and an industrial ironmaking plant, and a marked improvement in the prediction performance is achieved when VTD information is considered.
更多查看译文
关键词
Blast furnace (BF),dynamic deep network,particle swarm optimization (PSO),silicon (Si) content prediction,variable time delay (VTD) estimation
AI 理解论文
溯源树
样例
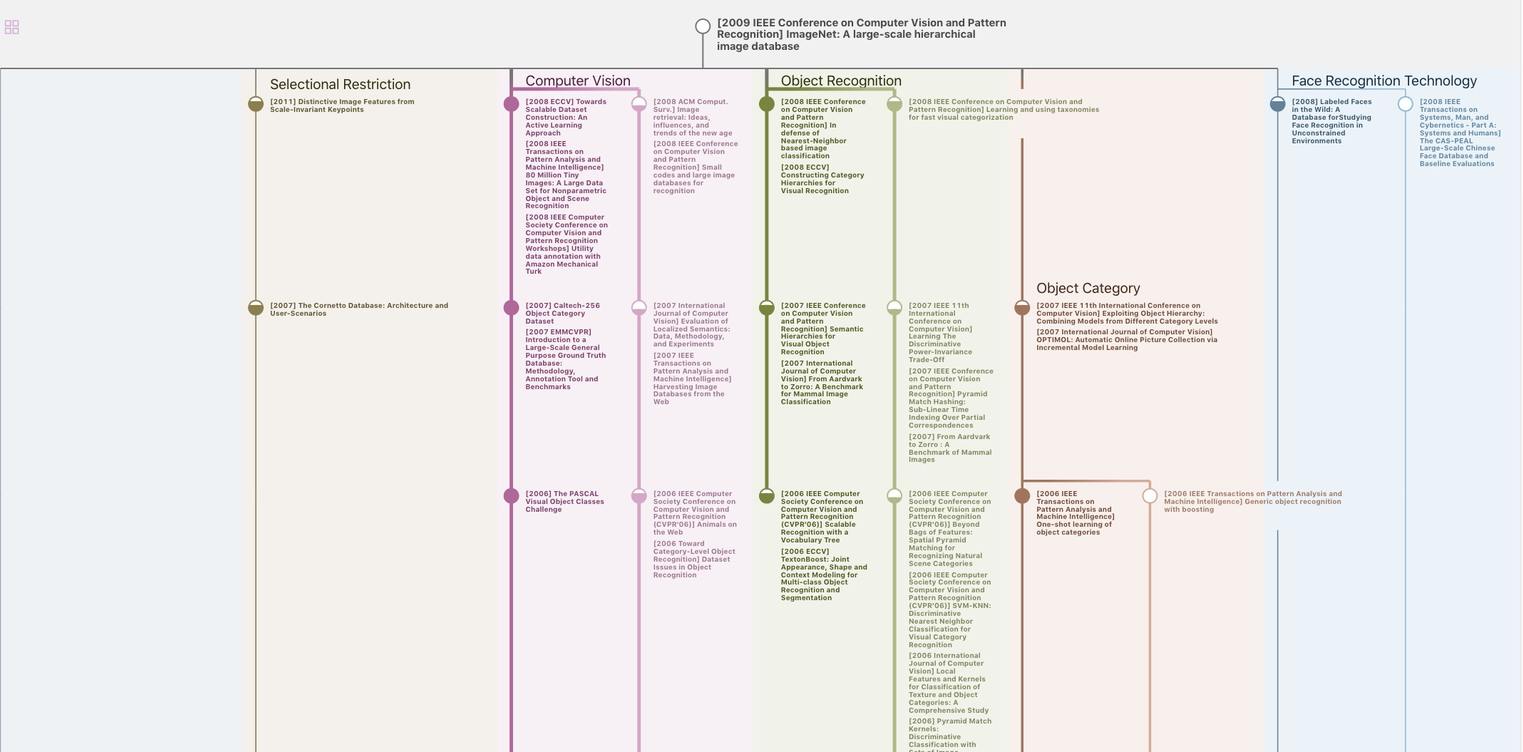
生成溯源树,研究论文发展脉络
Chat Paper
正在生成论文摘要