Lesion segmentation on 18F-fluciclovine PET/CT images using deep learning
Frontiers in Oncology(2023)
摘要
Background and purpose A novel radiotracer, F-18-fluciclovine (anti-3-18F-FACBC), has been demonstrated to be associated with significantly improved survival when it is used in PET/CT imaging to guide postprostatectomy salvage radiotherapy for prostate cancer. We aimed to investigate the feasibility of using a deep learning method to automatically detect and segment lesions on F-18-fluciclovine PET/CT images.Materials and methods We retrospectively identified 84 patients who are enrolled in Arm B of the Emory Molecular Prostate Imaging for Radiotherapy Enhancement (EMPIRE-1) trial. All 84 patients had prostate adenocarcinoma and underwent prostatectomy and( 18)F-fluciclovine PET/CT imaging with lesions identified and delineated by physicians. Three different neural networks with increasing levels of complexity (U-net, Cascaded U-net, and a cascaded detection segmentation network) were trained and tested on the 84 patients with a fivefold cross-validation strategy and a hold-out test, using manual contours as the ground truth. We also investigated using both PET and CT or using PET only as input to the neural network. Dice similarity coefficient (DSC), 95th percentile Hausdorff distance (HD95), center-of-mass distance (CMD), and volume difference (VD) were used to quantify the quality of segmentation results against ground truth contours provided by physicians.Results All three deep learning methods were able to detect 144/155 lesions and 153/155 lesions successfully when PET+CT and PET only, respectively, served as input. Quantitative results demonstrated that the neural network with the best performance was able to segment lesions with an average DSC of 0.68 +/- 0.15 and HD95 of 4 +/- 2 mm. The center of mass of the segmented contours deviated from physician contours by approximately 2 mm on average, and the volume difference was less than 1 cc. The novel network proposed by us achieves the best performance compared to current networks. The addition of CT as input to the neural network contributed to more cases of failure (DSC = 0), and among those cases of DSC > 0, it was shown to produce no statistically significant difference with the use of only PET as input for our proposed method.Conclusion Quantitative results demonstrated the feasibility of the deep learning methods in automatically segmenting lesions on F-18-fluciclovine PET/CT images. This indicates the great potential of F-18-fluciclovine PET/CT combined with deep learning for providing a second check in identifying lesions as well as saving time and effort for physicians in contouring.
更多查看译文
关键词
segmentation,PET/CT,neural network,prostate radiotherapy,deep learning
AI 理解论文
溯源树
样例
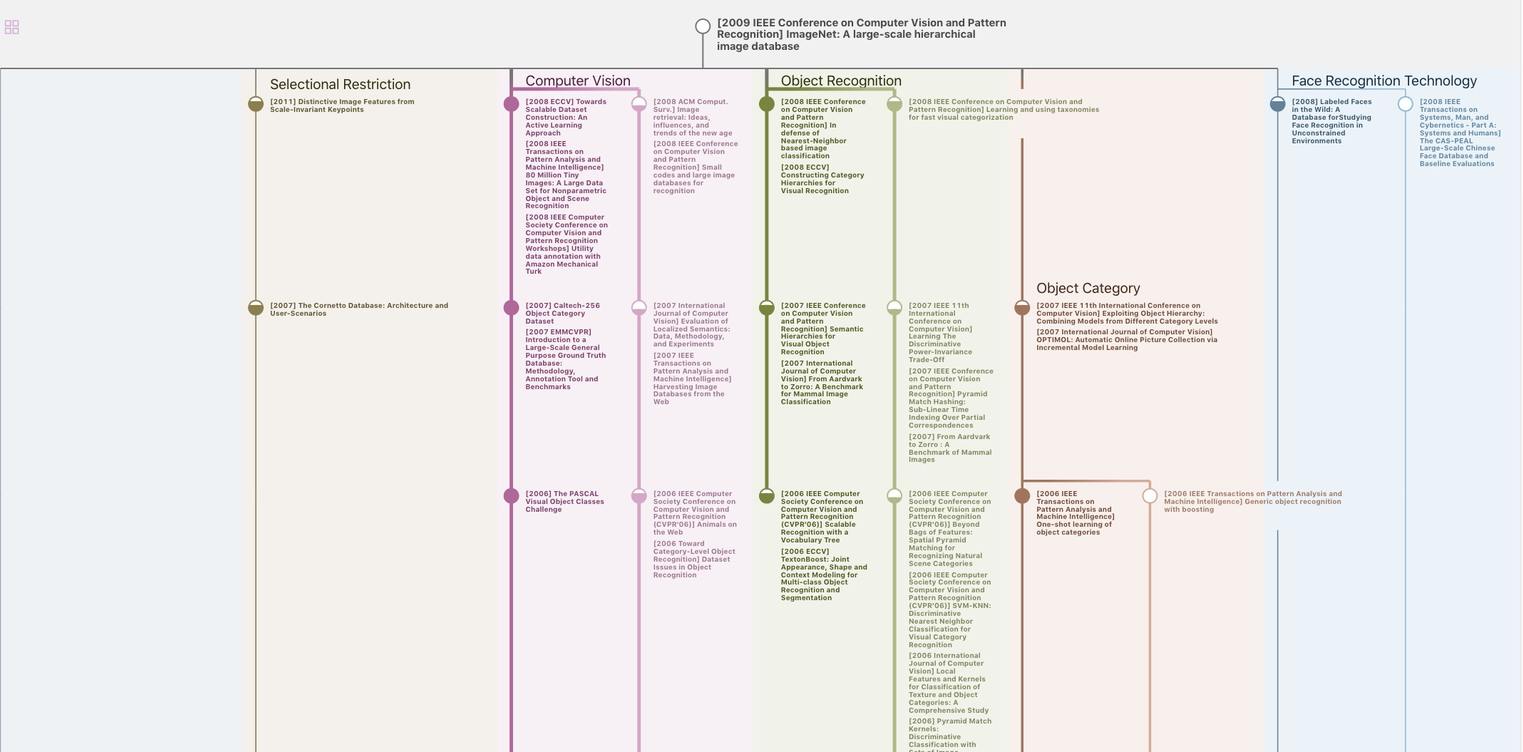
生成溯源树,研究论文发展脉络
Chat Paper
正在生成论文摘要