A Surrogate Model for Studying Solar Energetic Particle Transport and the Seed Population
SPACE WEATHER-THE INTERNATIONAL JOURNAL OF RESEARCH AND APPLICATIONS(2023)
摘要
The high energy particles originating from the Sun, known as solar energetic particles (SEPs), contribute significantly to the space radiation environment, posing serious threats to astronauts and scientific instruments on board spacecraft. The mechanism that accelerates the SEPs to the observed energy ranges, their transport in the inner heliosphere, and the influence of suprathermal seed particle spectrum are open questions in heliophysics. Accurate predictions of the occurrences of SEP events well in advance are necessary to mitigate their adverse effects but prediction based on first principle models still remains a challenge. In this scenario, adopting a machine learning approach to SEP modeling and prediction is desirable. However, the lack of a balanced database of SEP events restrains this approach. We addressed this limitation by generating large data sets of synthetic SEP events sampled from the physics-based model, Energetic Particle Radiation Environment Module (EPREM). Using this data, we developed neural networks-based surrogate models to study the seed population parameter space. Our models, EPREM-S, run thousands to millions of times faster (depending on computer hardware), making simulation-based inference workflows practicable in SEP studies while providing predictive uncertainty estimates using a deep ensemble approach. Space weather refers to the spatio-temporal variations in the near-Earth environment constituted by the solar wind. These variations cause disruptions in the technological infrastructure on Earth and in space. Outside the Earth's protective envelope, spacecraft are exposed to a harsh radiation environment constituted by the galactic cosmic rays and solar energetic particles (SEPs), posing serious threats to the astronauts and the electronics on board. Mitigation of space radiation hazard includes shielding but radiation exceeding 100 MeV produces secondary penetrating particles and nuclear fragments in the shielding material. The alternate solution is to predict the occurrences of SEPs well in advance. Since predicting the arrival time and location of SEPs based on first principle models remains a challenge, adopting a machine learning approach to SEP modeling and prediction is desirable. However, the lack of a balanced database of SEP events restrains this approach. We addressed this limitation by generating synthetic SEP events sampled from the physics-based model Energetic Particle Radiation Environment Module and developed neural networks-based surrogate models to study the seed population parameter space. Our models run thousands to millions of times faster, making simulation-based inference workflows practicable in SEP studies while providing predictive uncertainty estimates using a deep ensemble approach. Developed a surrogate model EPREM-S yielding the same output as EPREM, with low predictive uncertainty while thousands of times fasterA proof-of-concept study of EPREM-S and EPREM revealed that the surrogate models achieved generalization beyond the training setIn an event-analysis, as a Bayesian inference problem, all input parameters' ground truth values were recovered as mode of the posterior
更多查看译文
关键词
surrogate model,neural network,solar energetic particle,uncertainty quantification,seed population,EPREM
AI 理解论文
溯源树
样例
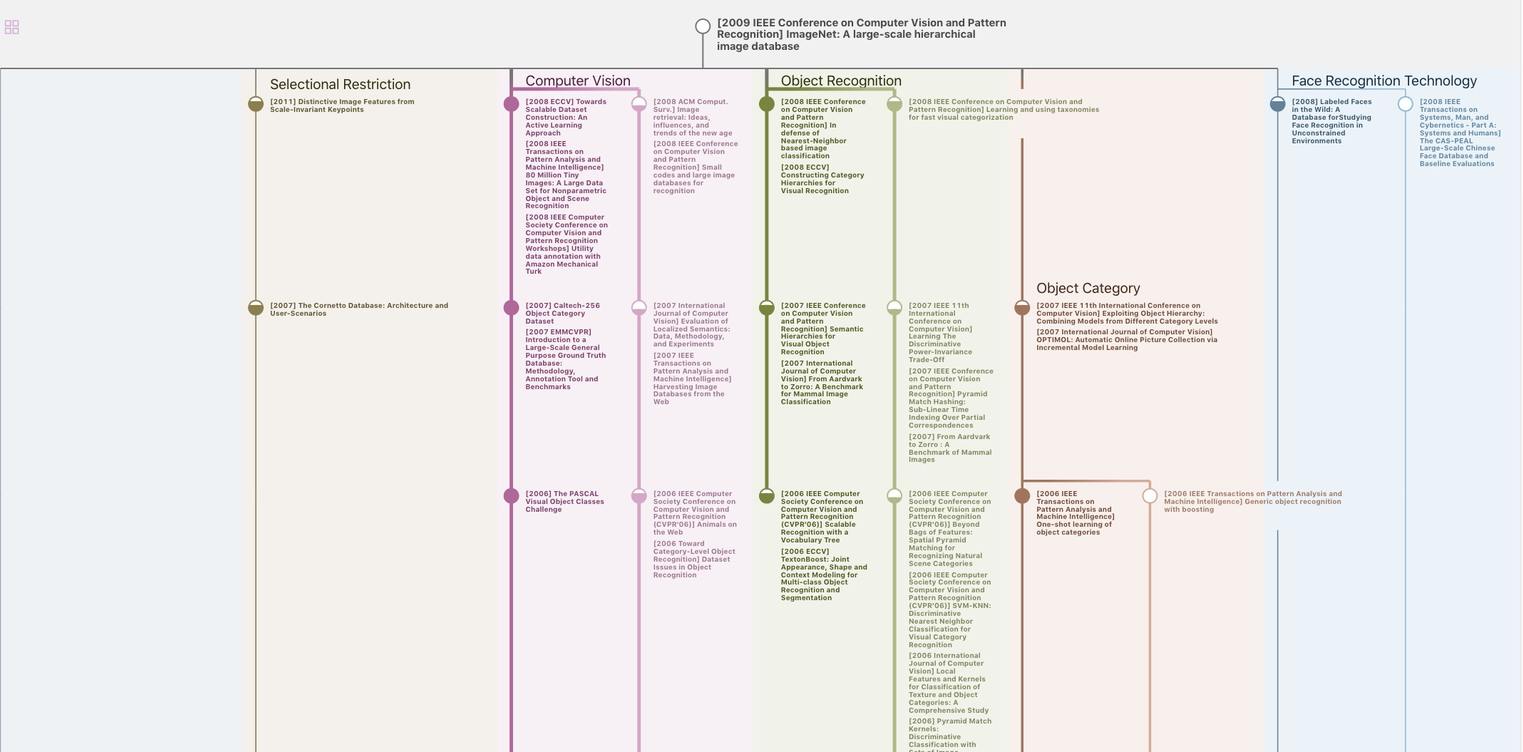
生成溯源树,研究论文发展脉络
Chat Paper
正在生成论文摘要