Enhancing active noise control of road noise using deep neural network to update secondary path estimate in real time
MECHANICAL SYSTEMS AND SIGNAL PROCESSING(2024)
摘要
The performance of active noise control (ANC) is significantly influenced by the accuracy of the secondary path estimate. In the case of a vehicle, dynamic environments, which constantly change the secondary path, can degrade the ANC performance. To address this issue, we proposed a deep learning-based method to continuously update the secondary path estimate in real time. The sensitivity of secondary paths to various conditions was analyzed, with vertical, horizontal, roll, and yaw conditions identified as primary factors; subsequently, a dataset corresponding to these conditions was created. Estimation models were formulated using Delaunay triangulation-based interpolation and three deep neural network (DNN)-based methods which incorporated diverse representations of the secondary path domain. All the methods precisely estimated the secondary path under different conditions. Notably, DNN-based methods required significantly less data storage than the interpolation method, with the principal component analysis (PCA)-based approach performing the best, achieving a 98.5 % reduction. Using this method, updating the secondary path estimate in real time led to reductions of 10.2 and 17.2 dB in road noise and 500-1,000 Hz random noise, respectively. The improvement was notable for both types of noises, indicating that the suggested method can expand the frequency range of ANC.
更多查看译文
关键词
Active noise control,Road noise,Secondary path estimation,Real -time update,Deep neural network,Principal component analysis
AI 理解论文
溯源树
样例
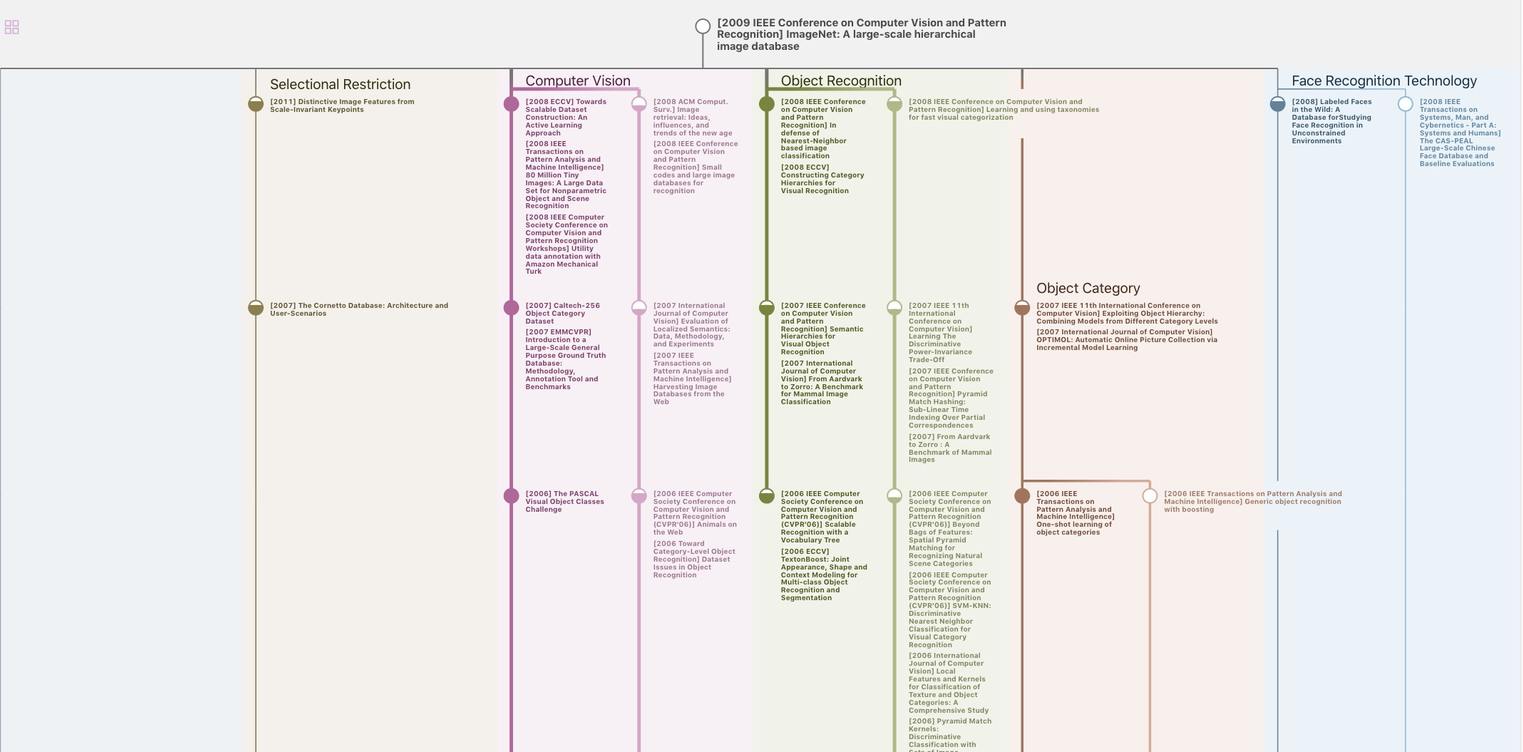
生成溯源树,研究论文发展脉络
Chat Paper
正在生成论文摘要