Biomedical signal to image conversion and classification using flexible deep learning techniques.
Int. J. Bus. Intell. Data Min.(2024)
摘要
Most diseases are diagnosed from image data like CT scans, MRIs, and X-rays. Gene data carries vital information related to the diseases that needs to be analysed for diagnosis. Both the logics are combined and a flexible deep ensemble learning-based model is proposed for the classification of images generated from one-dimensional (1D) data. Earlier works in the detection of brain tumours and epileptic seizures have been developed either directly providing 1D data or images to the classification model, whereas the proposed method utilises the effectiveness of two-dimensional (2D) convolutional neural networks (CNNS) to analyse 1D data like gene expressions and EEG signals after effective conversion to images. The data conversion is performed using three data reduction techniques, i.e., locally linearly embedding (LL-Embedding), multi-dimensional scaling (MDS), and t-distributed stochastic neighbour embedding (t-SNE) with convex hull algorithm to wrap all the data points. Multilayer perceptron is used for second-stage training. The proposed method is verified using brain tumour gene data collected from the genomic data commons (GDC) data portal and the EEG data for epileptic seizures detection provided by the University of Bonn (UoB dataset) and provided 97.38% and 97.33% accuracies respectively.
更多查看译文
关键词
gene to image,EEG to image,convolution neural network,ensemble learning,multilayer perception,brain tumour,epileptic,seizure,convex hull
AI 理解论文
溯源树
样例
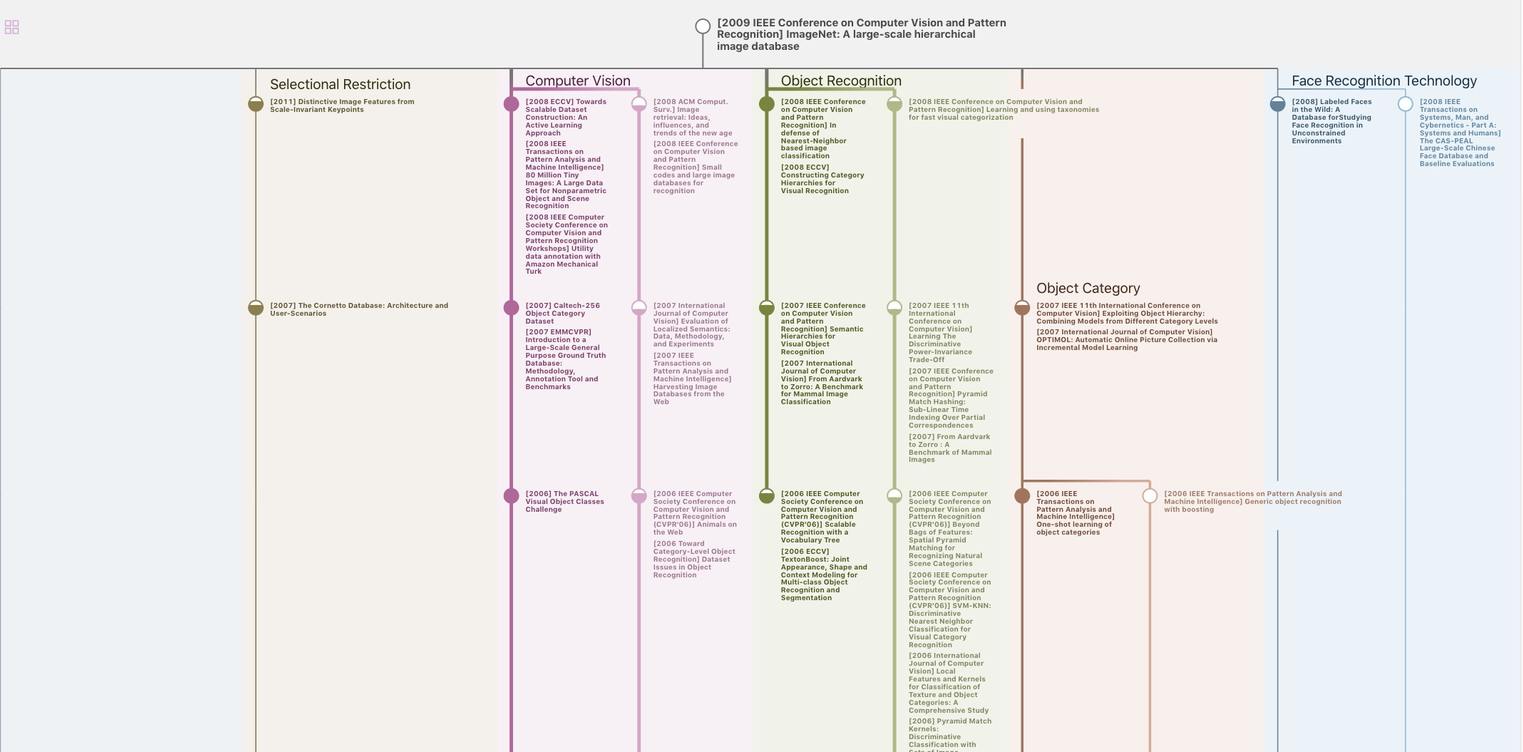
生成溯源树,研究论文发展脉络
Chat Paper
正在生成论文摘要