Learnable Spatial-Spectral Transform-Based Tensor Nuclear Norm for Multi-Dimensional Visual Data Recovery
IEEE Transactions on Circuits and Systems for Video Technology(2023)
摘要
Recently, transform-based tensor nuclear norm (TNN) methods have received increasing attention as a powerful tool for multi-dimensional visual data (color images, videos, and multispectral images, etc.) recovery. Especially, the redundant transform-based TNN achieves satisfactory recovery results, where the redundant transform along spectral mode can remarkably enhance the low-rankness of tensors. However, it suffers from expensive computational cost induced by the redundant transform. In this paper, we propose a learnable spatial-spectral transform-based TNN model for multi-dimensional visual data recovery, which not only enjoys better low-rankness capability but also allows us to design fast algorithms accompanying it. More specifically, we first project the large-scale original tensor to the small-scale intrinsic tensor via the learnable semi-orthogonal transforms along the spatial modes. Here, the semi-orthogonal transforms, serving as the key building block, can boost the spatial low-rankness and lead to a small-scale problem, which paves the way for designing fast algorithms. Secondly, to further boost the low-rankness, we apply the learnable redundant transform along the spectral mode to the small-scale intrinsic tensor. To tackle the proposed model, we apply an efficient proximal alternating minimization-based algorithm, which enjoys a theoretical convergence guarantee. Extensive experimental results on real-world data (color images, videos, and multispectral images) demonstrate that the proposed method outperforms state-of-the-art competitors in terms of evaluation metrics and running time.
更多查看译文
关键词
Tensor completion,semi-orthogonal transform,redundant transform,tensor nuclear norm,proximal alternating minimization algorithm
AI 理解论文
溯源树
样例
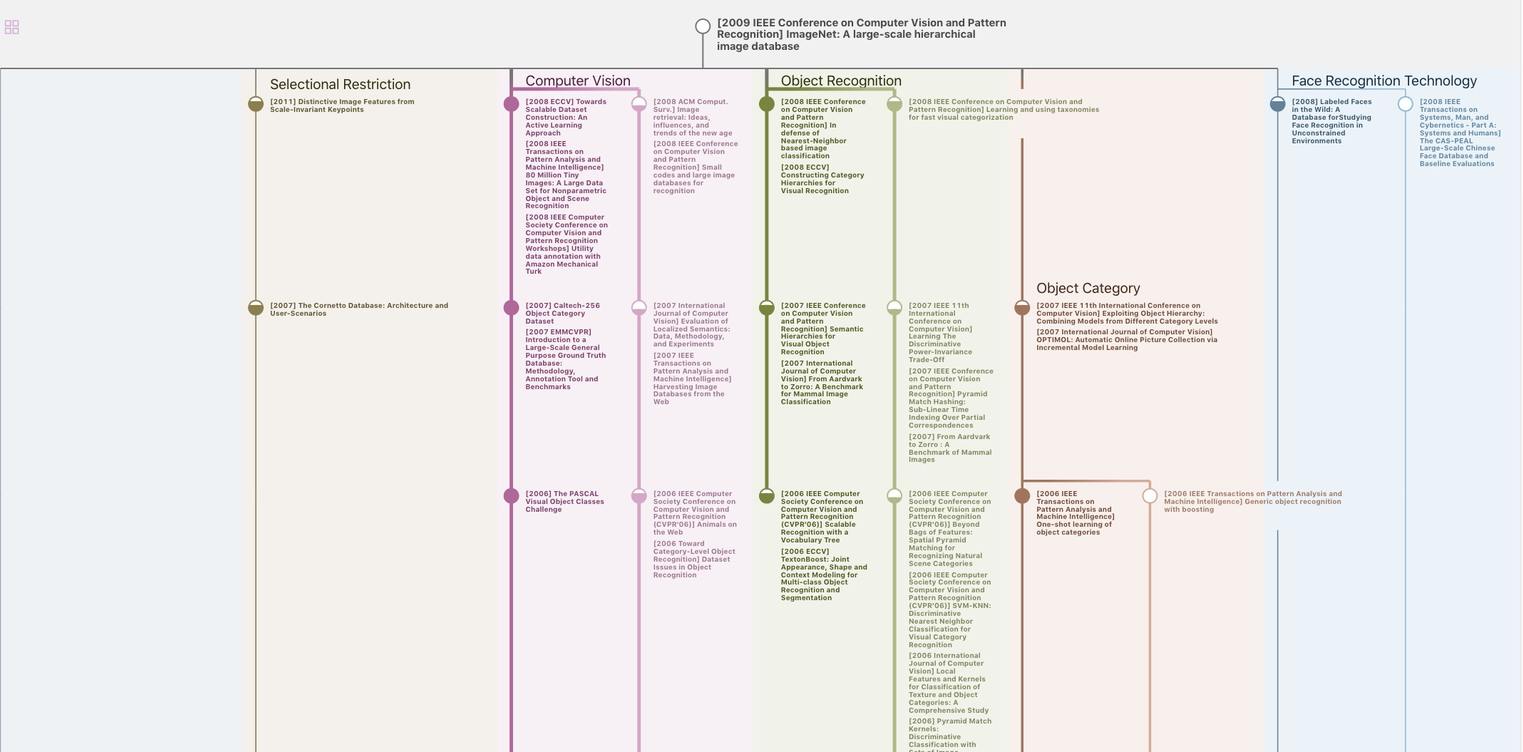
生成溯源树,研究论文发展脉络
Chat Paper
正在生成论文摘要