Innovative series binary ai strategies for improved detection of incorrectly positioned dental panoramic
Journal of Medical Imaging and Radiation Sciences(2023)
摘要
OBJECTIVE Correct positioning is vital in dental panoramic imaging, but errors frequently occur. This study aimed to develop a blended AI approach for categorizing incorrect positioning in dental panoramic images using binary two-class CNN methods. MATERIALS & METHODS A retrospective study (IRB: A202205095) was conducted with samples of normal (510), slumped position (E1, 375), chin tipped high or low (E2, 717), open lip or tongue against the palate (E3, 546), and head turned to one side or tilted (E4, 300) images. The blended AI approach involved a series of binary two-class CNN methods comparing normal and each error category. The hyperparameters included batch size, epoch size, and learning rates (0.001). The dataset was divided into 70%, 20%, and 10% for training, validation, and testing of the CNN models, respectively. Classification performance was assessed using accuracy and Kappa value, following a 2-fold cross-validation schema. RESULTS The accuracy (Kappa value) achieved for normal and E1, normal and E2, normal and E3, and normal and E4 were 0.9986 (0.9956), 0.9976 (0.9937), 0.9895 (0.9475), and 0.9968 (0.9912), respectively. CONCLUSION The study demonstrated that a blended AI approach using binary two-class CNN methods effectively categorizes error positioning factors in dental panoramic images. This innovative strategy has potential value for clinical applications in dental panoramic imaging. Correct positioning is vital in dental panoramic imaging, but errors frequently occur. This study aimed to develop a blended AI approach for categorizing incorrect positioning in dental panoramic images using binary two-class CNN methods. A retrospective study (IRB: A202205095) was conducted with samples of normal (510), slumped position (E1, 375), chin tipped high or low (E2, 717), open lip or tongue against the palate (E3, 546), and head turned to one side or tilted (E4, 300) images. The blended AI approach involved a series of binary two-class CNN methods comparing normal and each error category. The hyperparameters included batch size, epoch size, and learning rates (0.001). The dataset was divided into 70%, 20%, and 10% for training, validation, and testing of the CNN models, respectively. Classification performance was assessed using accuracy and Kappa value, following a 2-fold cross-validation schema. The accuracy (Kappa value) achieved for normal and E1, normal and E2, normal and E3, and normal and E4 were 0.9986 (0.9956), 0.9976 (0.9937), 0.9895 (0.9475), and 0.9968 (0.9912), respectively. The study demonstrated that a blended AI approach using binary two-class CNN methods effectively categorizes error positioning factors in dental panoramic images. This innovative strategy has potential value for clinical applications in dental panoramic imaging.
更多查看译文
关键词
improved detection
AI 理解论文
溯源树
样例
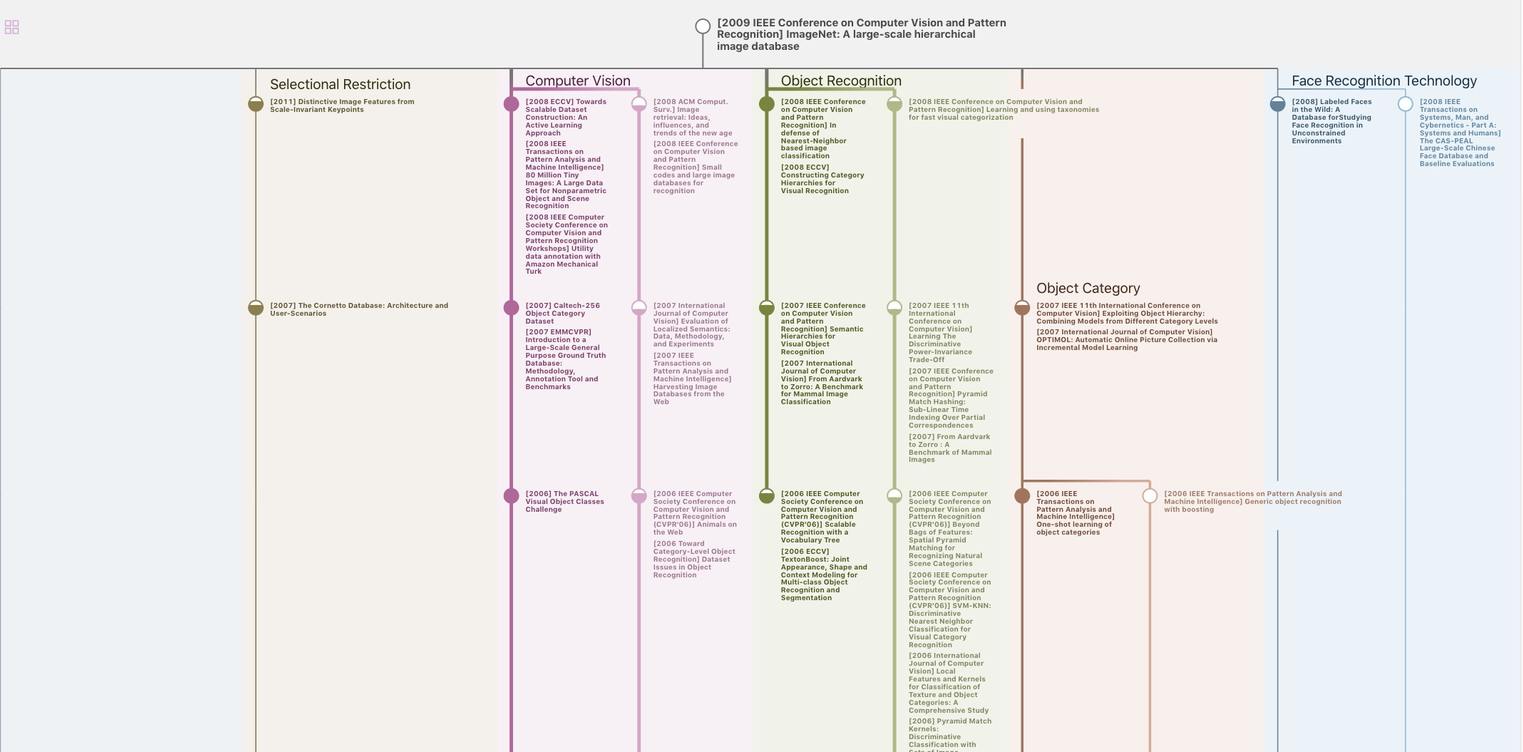
生成溯源树,研究论文发展脉络
Chat Paper
正在生成论文摘要