3D Semantic Subspace Traverser: Empowering 3D Generative Model with Shape Editing Capability
arXiv (Cornell University)(2023)
摘要
Shape generation is the practice of producing 3D shapes as various representations for 3D content creation. Previous studies on 3D shape generation have focused on shape quality and structure, without or less considering the importance of semantic information. Consequently, such generative models often fail to preserve the semantic consistency of shape structure or enable manipulation of the semantic attributes of shapes during generation. In this paper, we proposed a novel semantic generative model named 3D Semantic Subspace Traverser that utilizes semantic attributes for category-specific 3D shape generation and editing. Our method utilizes implicit functions as the 3D shape representation and combines a novel latent-space GAN with a linear subspace model to discover semantic dimensions in the local latent space of 3D shapes. Each dimension of the subspace corresponds to a particular semantic attribute, and we can edit the attributes of generated shapes by traversing the coefficients of those dimensions. Experimental results demonstrate that our method can produce plausible shapes with complex structures and enable the editing of semantic attributes. The code and trained models are available at https://github.com/TrepangCat/3D_Semantic_Subspace_Traverser
更多查看译文
关键词
3d generative model,shape
AI 理解论文
溯源树
样例
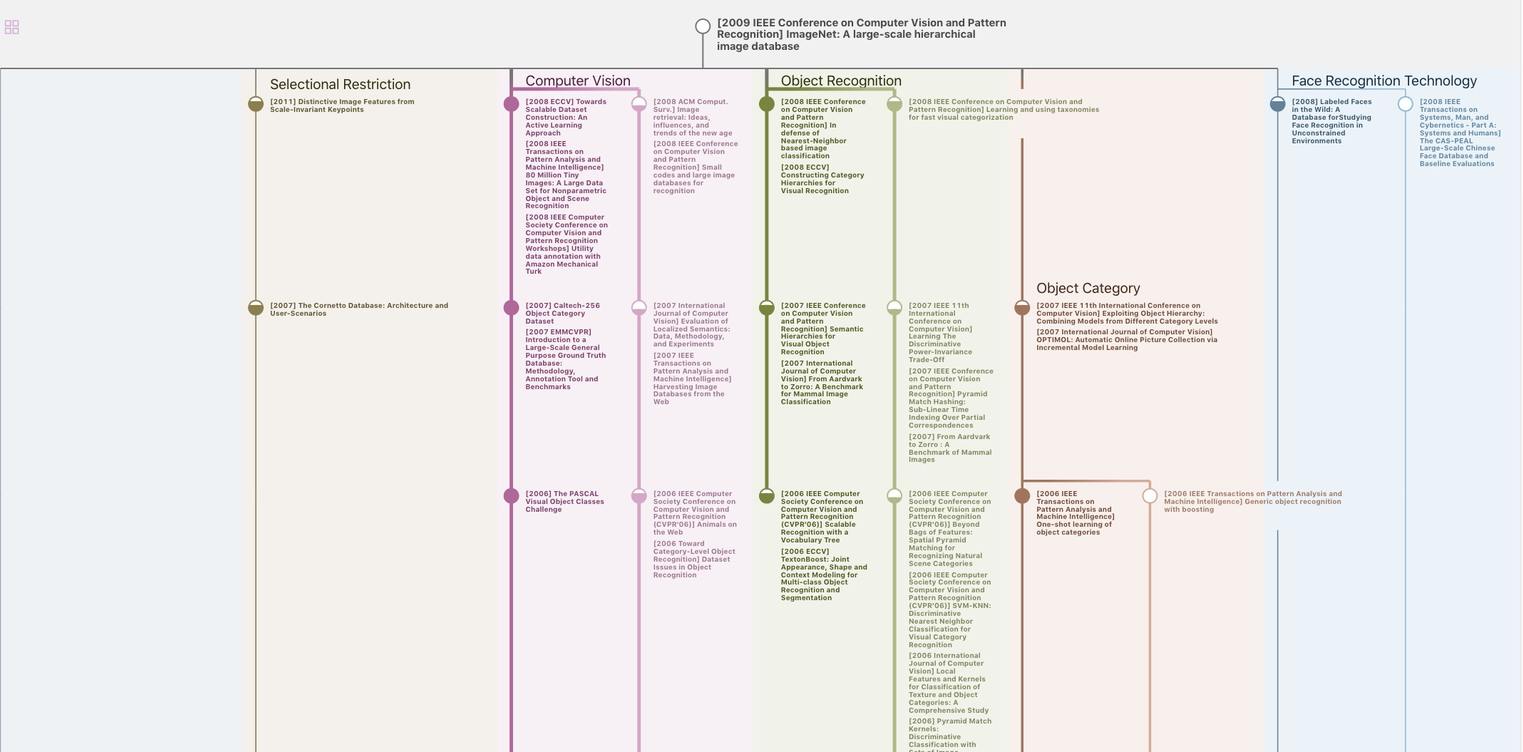
生成溯源树,研究论文发展脉络
Chat Paper
正在生成论文摘要