Effective Transfer of Pretrained Large Visual Model for Fabric Defect Segmentation via Specifc Knowledge Injection
arXiv (Cornell University)(2023)
摘要
Fabric defect segmentation is integral to textile quality control. Despite this, the scarcity of high-quality annotated data and the diversity of fabric defects present significant challenges to the application of deep learning in this field. These factors limit the generalization and segmentation performance of existing models, impeding their ability to handle the complexity of diverse fabric types and defects. To overcome these obstacles, this study introduces an innovative method to infuse specialized knowledge of fabric defects into the Segment Anything Model (SAM), a large-scale visual model. By introducing and training a unique set of fabric defect-related parameters, this approach seamlessly integrates domain-specific knowledge into SAM without the need for extensive modifications to the pre-existing model parameters. The revamped SAM model leverages generalized image understanding learned from large-scale natural image datasets while incorporating fabric defect-specific knowledge, ensuring its proficiency in fabric defect segmentation tasks. The experimental results reveal a significant improvement in the model's segmentation performance, attributable to this novel amalgamation of generic and fabric-specific knowledge. When benchmarking against popular existing segmentation models across three datasets, our proposed model demonstrates a substantial leap in performance. Its impressive results in cross-dataset comparisons and few-shot learning experiments further demonstrate its potential for practical applications in textile quality control.
更多查看译文
关键词
large visual model,segmentation,fabric defect
AI 理解论文
溯源树
样例
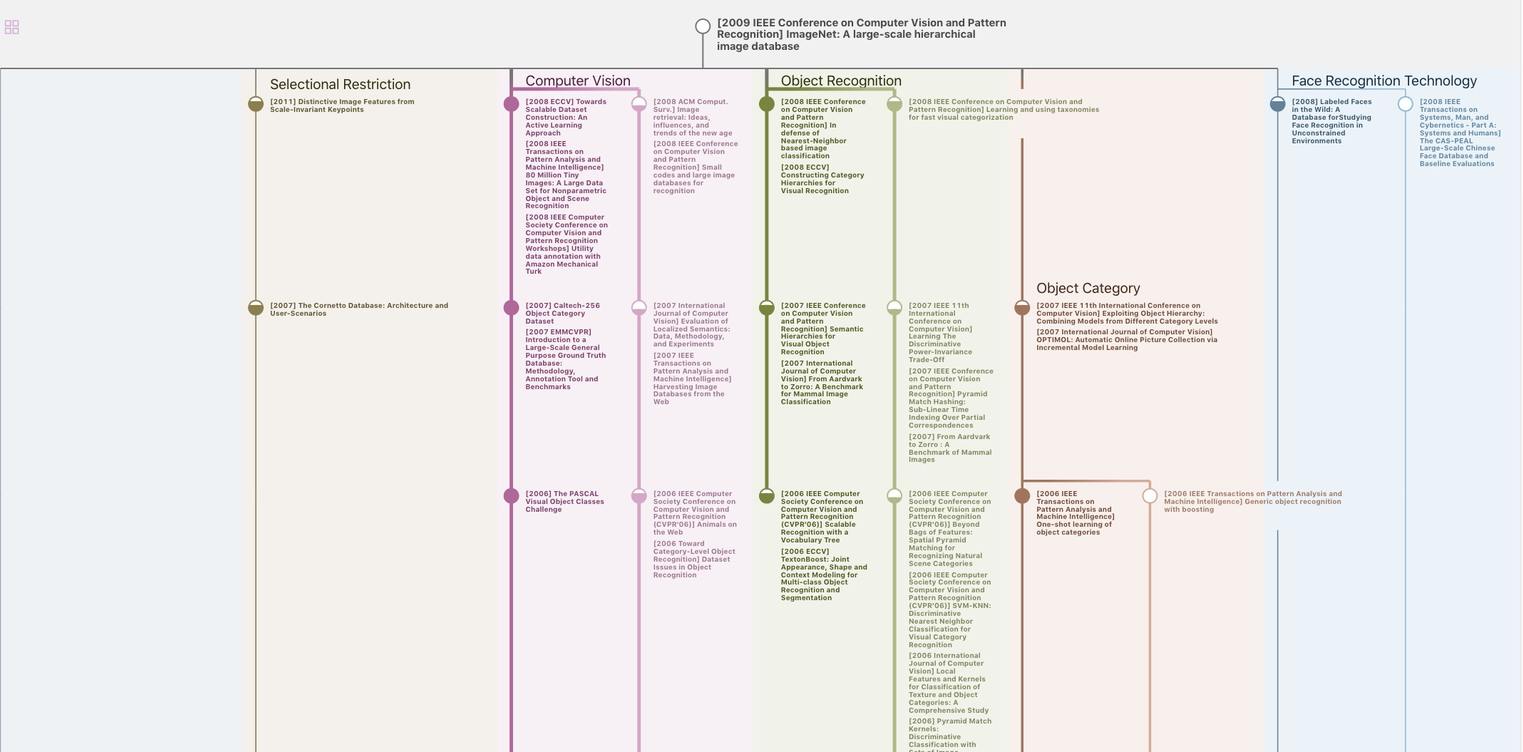
生成溯源树,研究论文发展脉络
Chat Paper
正在生成论文摘要