Spectral Bandwidth Recovery of Optical Coherence Tomography Images using Deep Learning
arXiv (Cornell University)(2023)
摘要
Optical coherence tomography (OCT) captures cross-sectional data and is used for the screening, monitoring, and treatment planning of retinal diseases. Technological developments to increase the speed of acquisition often results in systems with a narrower spectral bandwidth, and hence a lower axial resolution. Traditionally, image-processing-based techniques have been utilized to reconstruct subsampled OCT data and more recently, deep-learning-based methods have been explored. In this study, we simulate reduced axial scan (A-scan) resolution by Gaussian windowing in the spectral domain and investigate the use of a learning-based approach for image feature reconstruction. In anticipation of the reduced resolution that accompanies wide-field OCT systems, we build upon super-resolution techniques to explore methods to better aid clinicians in their decision-making to improve patient outcomes, by reconstructing lost features using a pixel-to-pixel approach with an altered super-resolution generative adversarial network (SRGAN) architecture.
更多查看译文
关键词
optical coherence tomography images,optical coherence tomography,spectral bandwidth recovery,deep learning
AI 理解论文
溯源树
样例
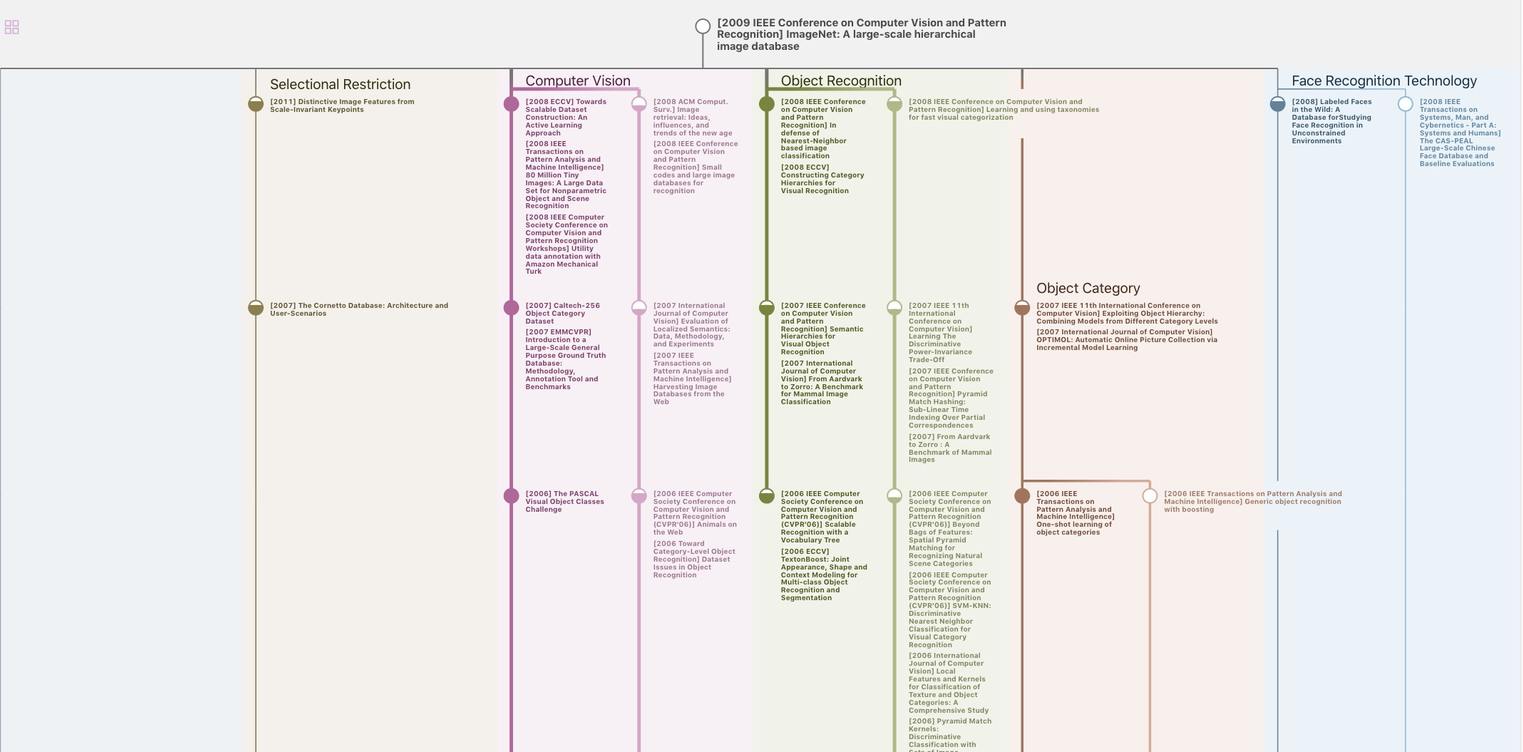
生成溯源树,研究论文发展脉络
Chat Paper
正在生成论文摘要