Attacking-Distance-Aware Attack: Semi-targeted Model Poisoning on Federated Learning.
IEEE Trans. Artif. Intell.(2024)
摘要
Existing model poisoning attacks on federated learning (FL) assume that an adversary has access to the full data distribution. In reality, an adversary usually has limited prior knowledge about clients' data. A poorly chosen target class renders an attack less effective. This work considers a semi-targeted situation where the source class is predetermined but the target class is not. The goal is to cause the misclassification of the global classifier on data from the source class. Approaches such as label flipping have been used to inject malicious parameters into FL. Nevertheless, it has shown that their performances are usually class-sensitive, varying with different target classes. Typically, an attack becomes less effective when shifting to a different target class. To overcome this challenge, we propose the Attacking Distance-aware Attack (ADA) that enhances model poisoning in FL by finding the optimized target class in the feature space. ADA deduces pair-wise class attacking distances using a Fast LAyer gradient MEthod (FLAME). Extensive evaluations were performed on five benchmark image classification tasks and three model architectures using varying attacking frequencies. Furthermore, ADA's robustness to conventional defenses of Byzantine-robust aggregation and differential privacy was validated. The results showed that ADA succeeded in increasing attack performance to 2.8 times in the most challenging case with an attacking frequency of 0.01 and bypassed existing defenses, where differential privacy that was the most effective defense still could not reduce the attack performance to below 50%.
更多查看译文
关键词
backdoor attack,model poisoning,federated learning,semi-targeted
AI 理解论文
溯源树
样例
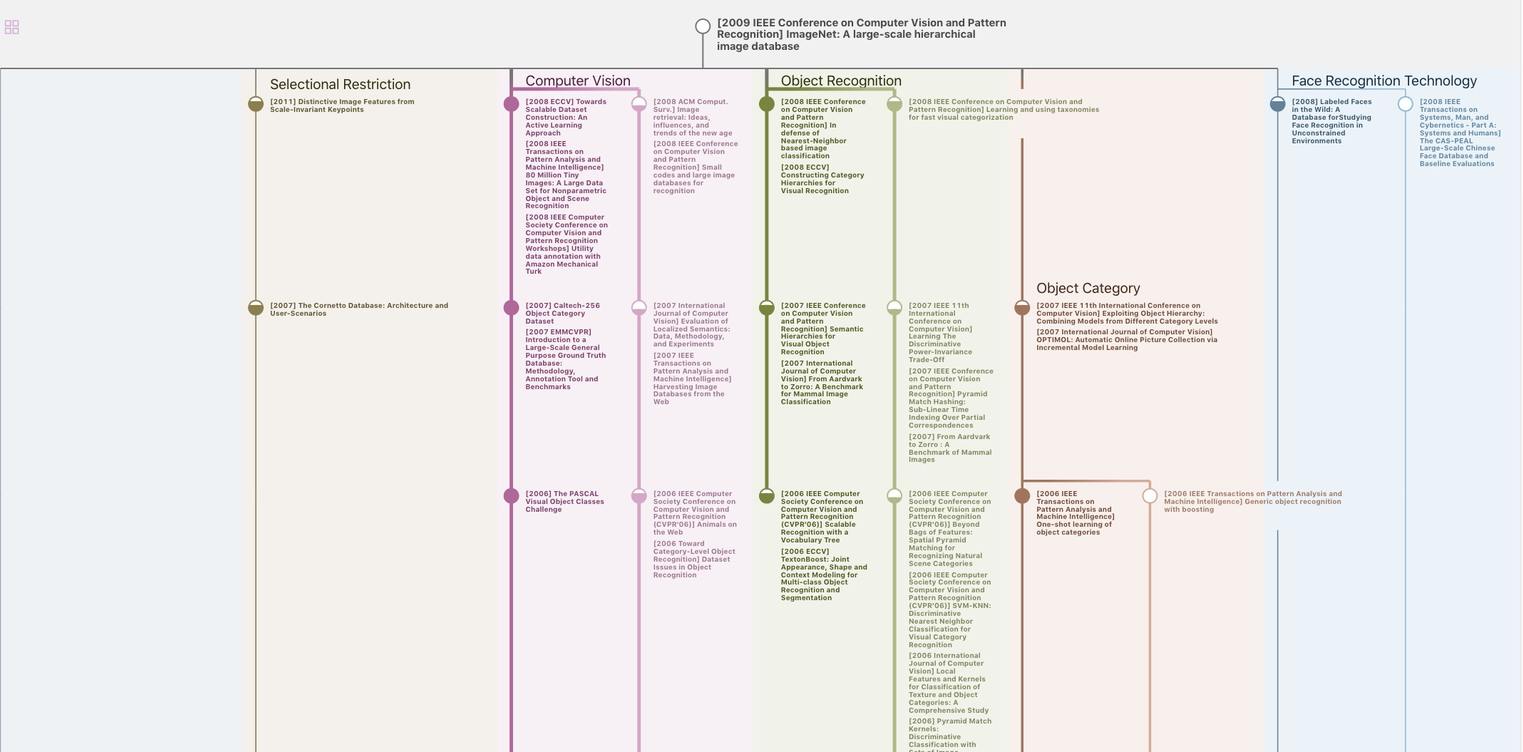
生成溯源树,研究论文发展脉络
Chat Paper
正在生成论文摘要