Dual Stage Stylization Modulation for Domain Generalized Semantic Segmentation
arXiv (Cornell University)(2023)
摘要
Obtaining sufficient labeled data for training deep models is often challenging in real-life applications. To address this issue, we propose a novel solution for single-source domain generalized semantic segmentation. Recent approaches have explored data diversity enhancement using hallucination techniques. However, excessive hallucination can degrade performance, particularly for imbalanced datasets. As shown in our experiments, minority classes are more susceptible to performance reduction due to hallucination compared to majority classes. To tackle this challenge, we introduce a dual-stage Feature Transform (dFT) layer within the Adversarial Semantic Hallucination+ (ASH+) framework. The ASH+ framework performs a dual-stage manipulation of hallucination strength. By leveraging semantic information for each pixel, our approach adaptively adjusts the pixel-wise hallucination strength, thus providing fine-grained control over hallucination. We validate the effectiveness of our proposed method through comprehensive experiments on publicly available semantic segmentation benchmark datasets (Cityscapes and SYNTHIA). Quantitative and qualitative comparisons demonstrate that our approach is competitive with state-of-the-art methods for the Cityscapes dataset and surpasses existing solutions for the SYNTHIA dataset. Code for our framework will be made readily available to the research community.
更多查看译文
关键词
dual stage stylization modulation,domain generalized semantic
AI 理解论文
溯源树
样例
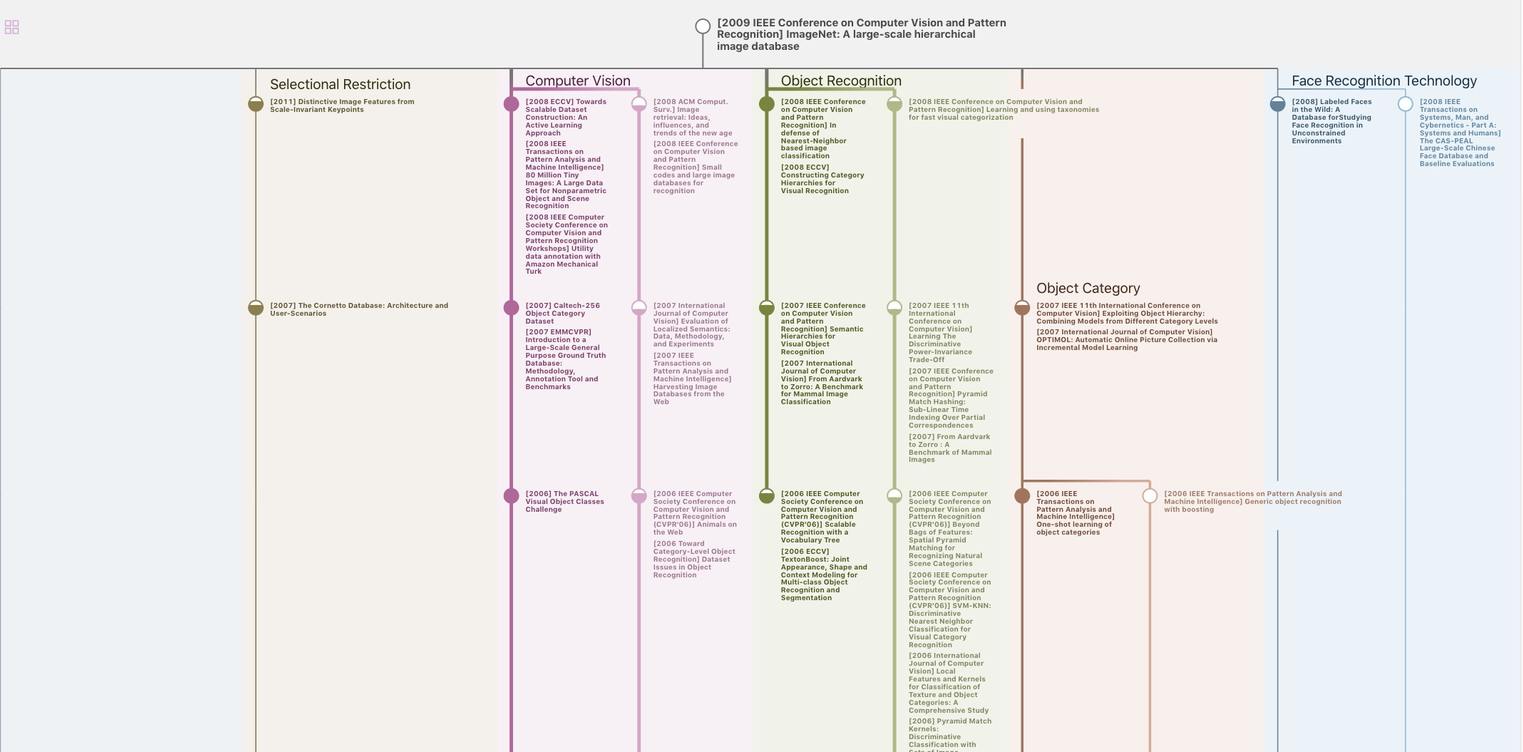
生成溯源树,研究论文发展脉络
Chat Paper
正在生成论文摘要