A semi-agnostic ansatz with variable structure for variational quantum algorithms
Quantum Machine Intelligence(2023)
摘要
Quantum machine learning—and specifically Variational Quantum Algorithms (VQAs)—offers a powerful, flexible paradigm for programming near-term quantum computers, with applications in chemistry, metrology, materials science, data science, and mathematics. Here, one trains an ansatz, in the form of a parameterized quantum circuit, to accomplish a task of interest. However, challenges have recently emerged suggesting that deep ansatzes are difficult to train, due to flat training landscapes caused by randomness or by hardware noise. This motivates our work, where we present a variable structure approach to build ansatzes for VQAs. Our approach, called VAns (Variable Ansatz), applies a set of rules to both grow and (crucially) remove quantum gates in an informed manner during the optimization. Consequently, VAns is ideally suited to mitigate trainability and noise-related issues by keeping the ansatz shallow. We employ VAns in the variational quantum eigensolver for condensed matter and quantum chemistry applications, in the quantum autoencoder for data compression and in unitary compilation problems showing successful results in all cases.
更多查看译文
关键词
Quantum machine learning,Variational quantum algorithms,Quantum circuit discovery
AI 理解论文
溯源树
样例
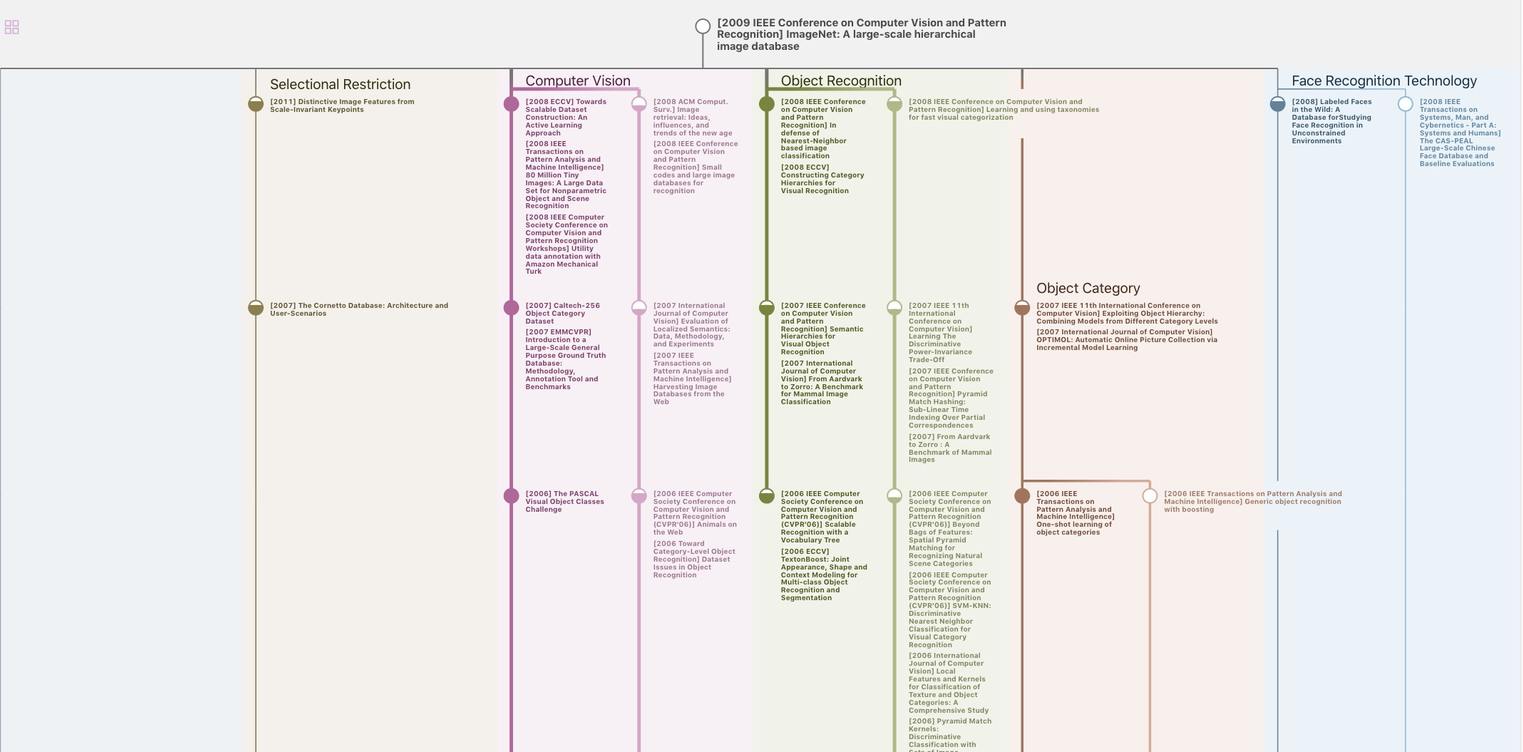
生成溯源树,研究论文发展脉络
Chat Paper
正在生成论文摘要