Emotion-cause pair extraction with bidirectional multi-label sequence tagging
Applied Intelligence(2023)
摘要
Emotion-cause pair extraction (ECPE) is a challenging natural language understanding task that aims to extract all potential emotion-cause clause pairs in a document. Existing works have adopted an end-to-end sequence tagging framework to solve this task. However, these approaches struggle to solve the overlapping pairs problem, which means more than one emotion-cause pairs share the same emotion or cause clause. And they suffer from poor generalization ability when the document contains multiple emotion-cause pairs. To address the mentioned issues, we propose a Bidirectional Multi-Label Sequence Tagging (BMST) framework in this paper. Specifically, the proposed method can leverage two sequence tagging modules to achieve bidirectional emotion-cause pair extraction, each of which integrates relevant distance information into the label scheme and employs a multi-label classification strategy. To improve the generalization ability of the model, we propose a joint reasoning mechanism that can exploit the two sequence tagging probabilities to interactively decode emotion-cause pairs. Besides, we design a distance-aware graph attention network to model the relationship between clauses of different distances. Experimental results show that BMST achieves state-of-the-art performance, demonstrating the effectiveness of our model.
更多查看译文
关键词
Emotion-cause pair extraction,Bidirectional tagging mechanism,Graph attention networks,Multi-label classification
AI 理解论文
溯源树
样例
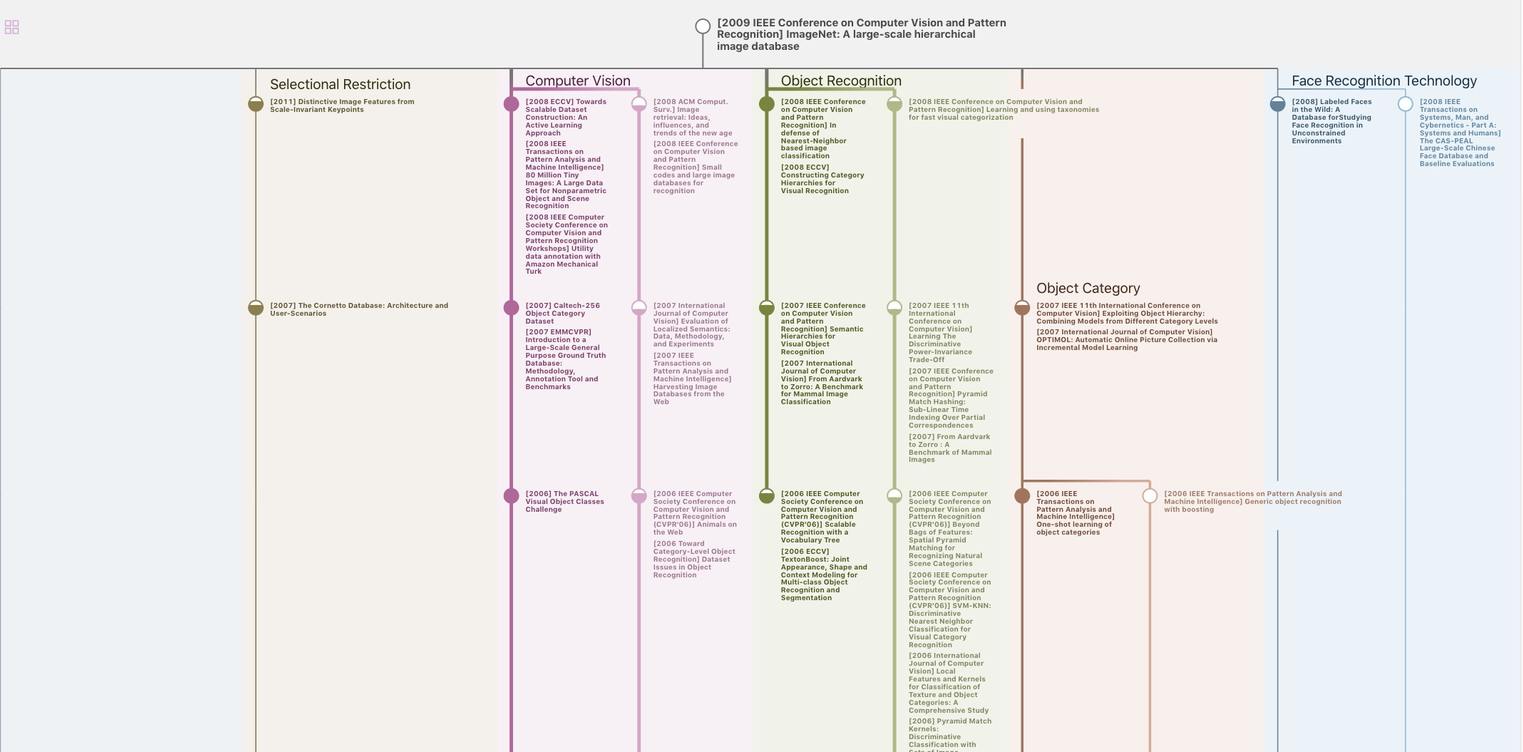
生成溯源树,研究论文发展脉络
Chat Paper
正在生成论文摘要