Machine-learning score to predict in-hospital outcomes in patients Hospitalized in Intensive Cardiac Care Unit
European Heart Journal(2023)
摘要
Abstract Background While few scores are available for risk stratification of patients hospitalized in Intensive Cardiac Care Unit (ICCU) using traditional statistical methods, the potential benefit of machine learning is not established. Purpose To investigate the feasibility and accuracy of machine-learning (ML) to predict in-hospital major adverse events (MAE) in patients hospitalized in ICCU and compare its performance with existing scores. Methods From April 7 to 22, 2021, a French nationwide, multicenter, prospective, study involving 39 centers included all consecutive patients admitted in ICCU (N=1499). The primary outcome was in-hospital MAE defined as a composite outcome including all-cause in-hospital mortality, cardiogenic shock and resuscitated cardiac arrest (severe ventricular arrhythmia requiring defibrillation or anti-arrhythmic agents). Twenty-eight clinical, biological, ECG, and echocardiographic variables were considered for the Boruta variable selection algorithm. Using 31 centers randomly as an index cohort, several ML algorithms (logistic regression, ridge regression, boosted cost-sensitive C5.0 and XGBoost. C5.0 ) were evaluated to build a ML-score to predict in-hospital MAE. The other 8 centers formed the external validation cohort of the ML-score. Results Among 1499 consecutive patients included (age 63.3±14.9 years, 69.6% male), 61 had in-hospital MAE (4.3%). Out of 28 variables included in the feature selection, seven were selected as of particular importance to predict MAE in the training set (N=844) including: illicit drug use, mean arterial pressure, Killip stage, exhaled CO level, left ventricular ejection fraction, TAPSE value and peak E/e’ ratio. Boosted cost-sensitive C5.0 technique showed the best performance compared with other ML-techniques (area under the curve [AUC]=0.90, PR-AUC=0.57, F1 score=0.5), using 5 of the 7 above mentioned variables: mean arterial pressure, exhaled CO level, left ventricular ejection fraction, TAPSE value and peak E/e’ ratio. Our ML-score exhibited a higher AUC compared with existing scores for MAE prediction (ML score: 0.90 vs TIMI-score: 0.56, GRACE-score: 0.52, Acute HF-score: 0.65; all p<0.001). ML-score also exhibited a good AUC in the external cohort (AUC 0.97). Conclusions Including five simple clinical and echocardiographic variables (mean arterial pressure, exhaled CO level, left ventricular ejection fraction, TAPSE value and peak E/e’ ratio) routinely assessed in patients admitted in ICCU, the ML score exhibited a better prognostic value to predict in-hospital outcomes compared with existing scores.ML model and existing scores: ROC curveML model and existing scores: PR curve
更多查看译文
关键词
machine-learning machine-learning,score,outcomes,in-hospital
AI 理解论文
溯源树
样例
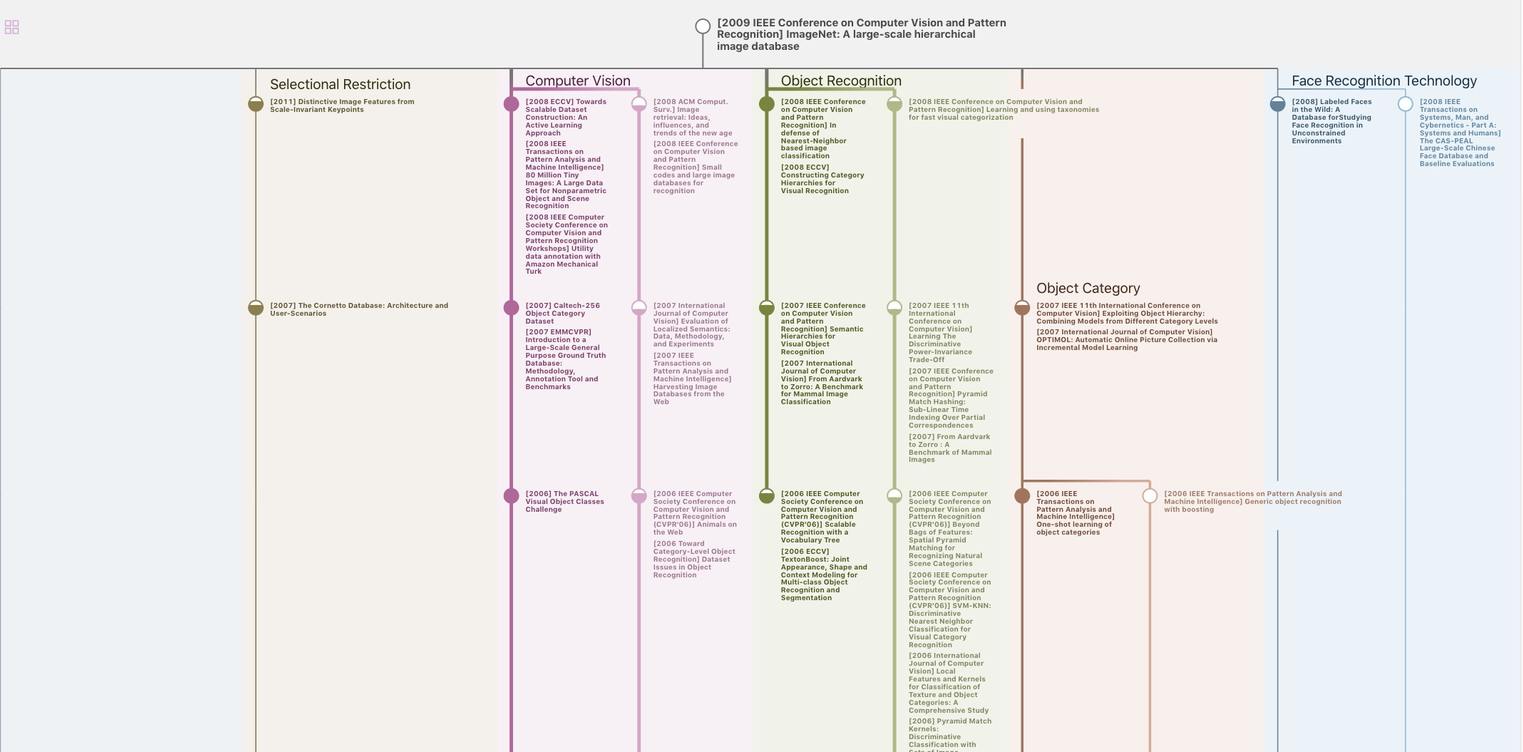
生成溯源树,研究论文发展脉络
Chat Paper
正在生成论文摘要