Three-dimensional reconstruction of granular porous media based on deep generative models
PHYSICAL REVIEW E(2023)
摘要
Reconstruction of microstructure in granular porous media, which can be viewed as granular assemblies, is crucial for studying their characteristics and physical properties in various fields concerned with the behavior of such media, including petroleum geology and computational materials science. In spite of the fact that many existing studies have investigated grain reconstruction, most of them treat grains as simplified individuals for discrete reconstruction, which cannot replicate the complex geometrical shapes and natural interactions between grains. In this work, a hybrid generative model based on a deep-learning algorithm is proposed for high-quality three-dimensional (3D) microstructure reconstruction of granular porous media from a single two-dimensional (2D) slice image. The method extracts 2D prior information from the given image and generates the grain set as a whole. Both a self-attention module and effective pattern loss are introduced in a bid to enhance the reconstruction ability of the model. Samples with grains of varied geometrical shapes are utilized for the validation of our method, and experimental results demonstrate that our proposed approach can accurately reproduce the complex morphology and spatial distribution of grains without any artificiality. Furthermore, once the model training is complete, rapid end-to-end generation of diverse 3D realizations from a single 2D image can be achieved.
更多查看译文
关键词
granular porous media,deep generative models,reconstruction,three-dimensional
AI 理解论文
溯源树
样例
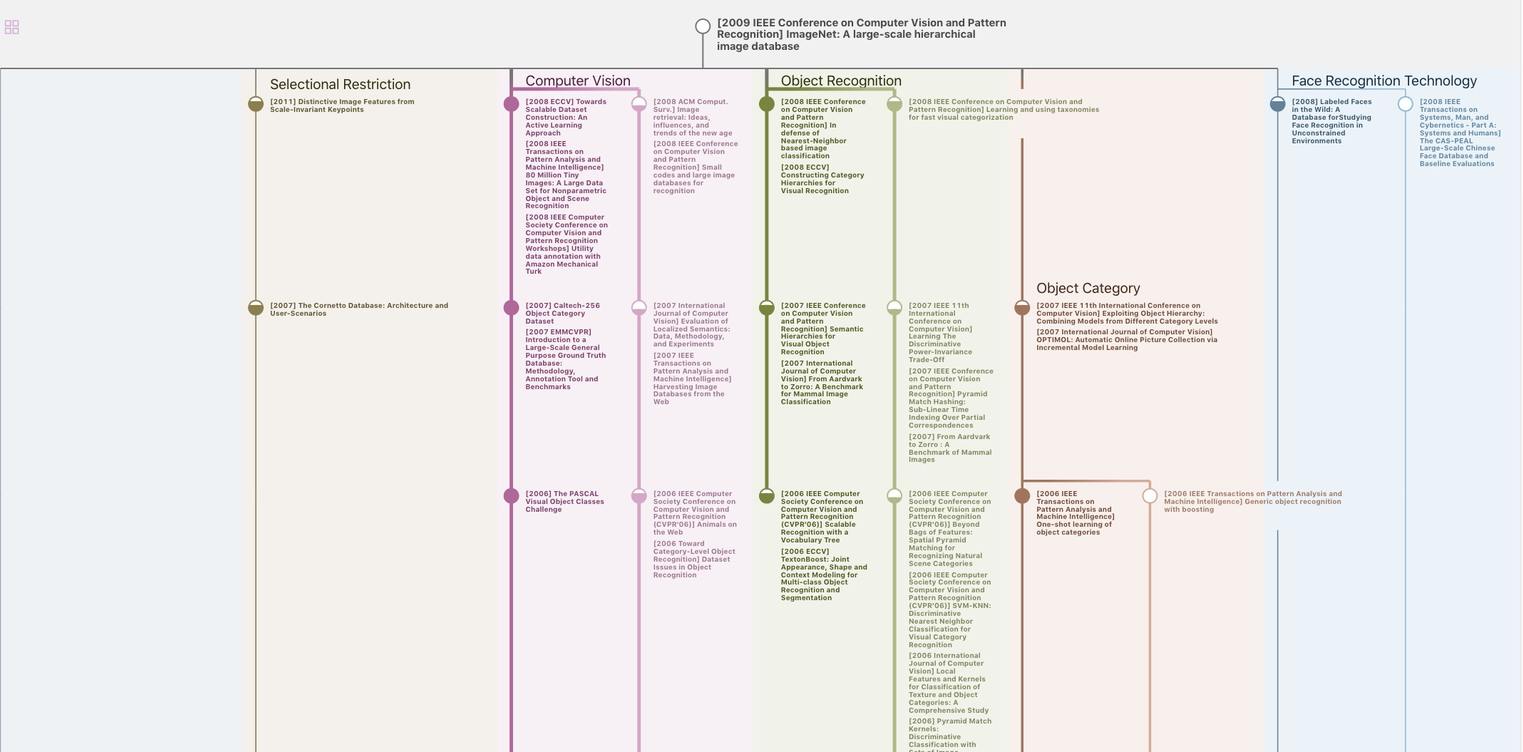
生成溯源树,研究论文发展脉络
Chat Paper
正在生成论文摘要