Cross-Type Representation Learning in GNSS: A Novel Approach for Enhanced SPP Positioning through Pseudorange Residuals
Research Square (Research Square)(2023)
摘要
Abstract In the evolving domain of satellite navigation, accurate Single Point Positioning (SPP) remains a paramount objective. After an in-depth analysis of pseudorange residuals' characteristics, this study unveils a pioneering method by harnessing cross-type representation learning within GNSS, seamlessly integrating the capabilities of natural language processing with conventional navigation methodologies. By innovatively translating diverse conditions into "literal" terms, we re-envision how navigation challenges are addressed. Our introduced C-SPP algorithm significantly surpasses established SPP methods like RTKLIB and NETDIFF, achieving a remarkable accuracy boost of 33.27% and 24.47%, respectively. Moreover, while there's a slight increment in computational time, it's impressively marginal, showcasing our method's balance between precision and efficiency. The research underscores the importance of context adaptability, emphasizing potential efficiency gains from regional applications. With a unique blend of advanced NLP and GNSS positioning methods, our study offers a refreshing lens to view and tackle pseudorange residuals, opening avenues for further research and applications.
更多查看译文
关键词
representation learning,gnss,pseudorange residuals,enhanced spp positioning,cross-type
AI 理解论文
溯源树
样例
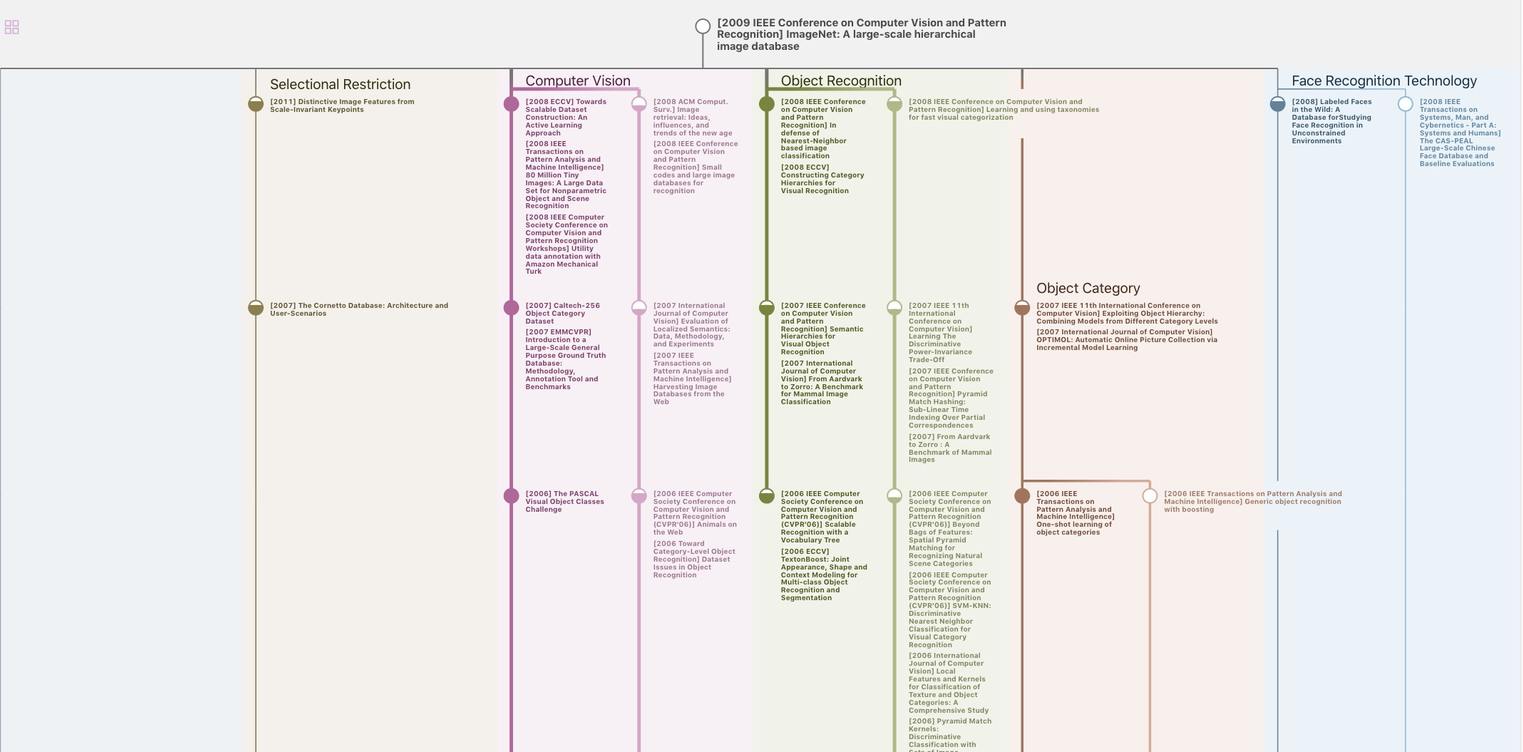
生成溯源树,研究论文发展脉络
Chat Paper
正在生成论文摘要