Multivariate selfsimilarity: Multiscale eigenstructures for selfsimilarity parameter estimation
IEEE Transactions on Signal Processing(2024)
摘要
Scale-free dynamics, formalized by selfsimilarity, provides a versatile paradigm massively and ubiquitously used to model temporal dynamics in real-world data. However, its practical use has mostly remained univariate so far. By contrast, modern applications often demand multivariate data analysis. Accordingly, models for multivariate selfsimilarity were recently proposed. Nevertheless, they have remained rarely used in practice because of a lack of available reliable estimation procedures for the vector of selfsimilarity parameters. Building upon recent mathematical developments, the present work puts forth an efficient estimation procedure based on the theoretical study of the multiscale eigenstructure of the wavelet spectrum of multivariate selfsimilar processes. The estimation performance is studied theoretically in the asymptotic limits of large scale and sample sizes, and computationally for finite-size samples. As a practical outcome, a fully operational and documented multivariate signal processing estimation toolbox is made freely available and is ready for practical use on real-world data. Its potential benefits are illustrated in epileptic seizure prediction from multi-channel EEG data.
更多查看译文
关键词
Multivariate selfsimilarity,selfsimilarity parameter vector,wavelet transform,multiscale eigen-structure
AI 理解论文
溯源树
样例
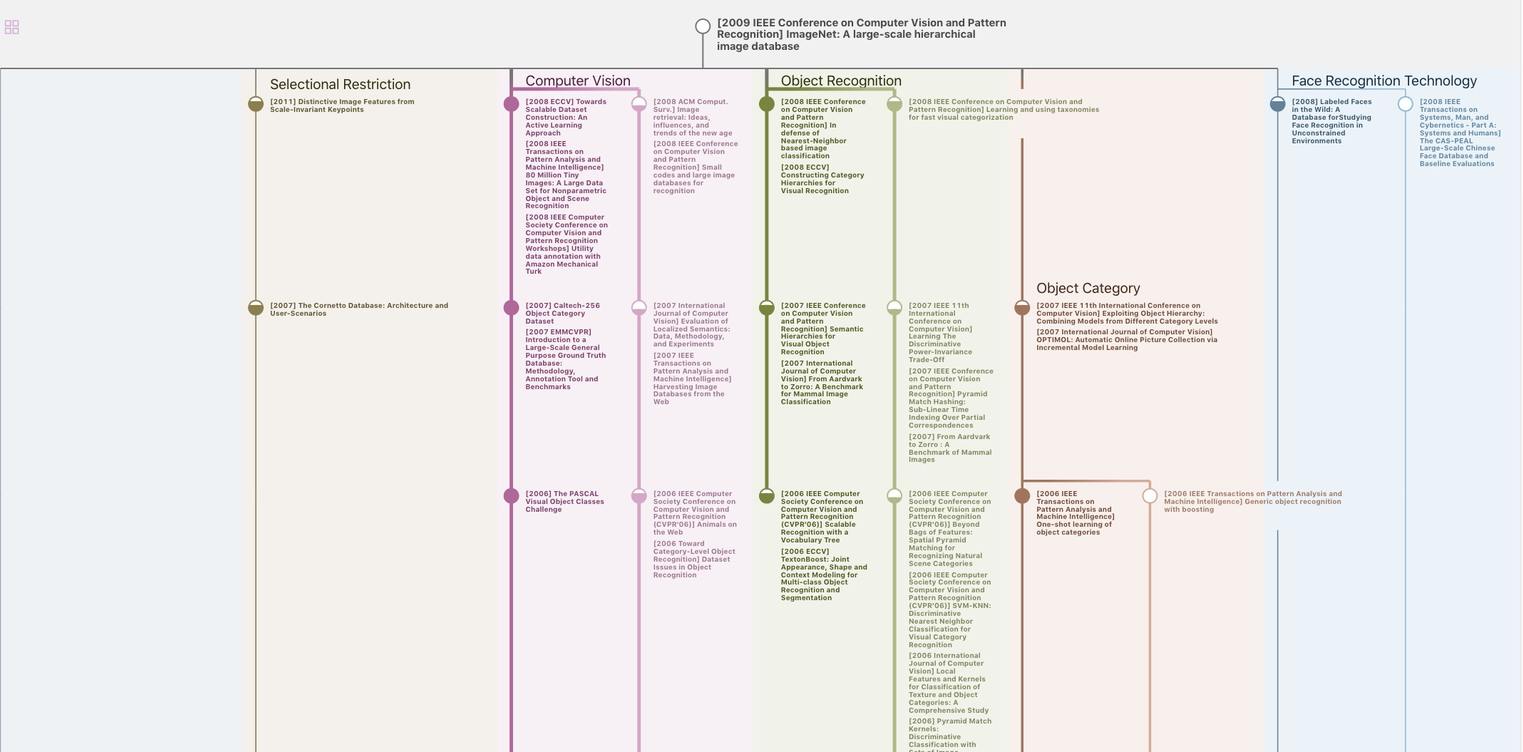
生成溯源树,研究论文发展脉络
Chat Paper
正在生成论文摘要