A Generalizable ECG-based Artificial Intelligence Model for 10-Year Heart Failure Risk Prediction
Cardiovascular Digital Health Journal(2023)
摘要
BackgroundHeart failure (HF) is a progressive condition with high global incidence. HF has two main subtypes: HF with preserved ejection fraction (HFpEF) and HF with reduced ejection fraction (HFrEF). There is an inherent need for simple yet effective ECG-based artificial intelligence (AI; ECG-AI) models which can predict HF risk early to allow for risk modification.ObjectiveThe main objectives were to validate HF risk prediction models using Multi-Ethnic Study of Atherosclerosis (MESA) data and assess performance of on HFpEF and HFrEF classification.MethodsECG-AI model was developed using raw 12-lead ECGs within a convolutional neural network (CNN). The clinical models from ARIC (ARIC-HF) and Framingham Heart Study (FHS-HF) used nine and eight variables, respectively. ECG-AI plus the clinical data within a Cox Proportional Hazard model (ECG-AI-Cox) and, for ECG-based comparison, a new Light Gradient Boosting Machine model using 288 ECG Characteristics (ECG-Chars) were developed. All the models were validated on MESA. The performances of these models were evaluated using the area under the receiver operating characteristic curve (ROC AUC) and compared using De-Long Test.ResultsECG-AI, ECG-Chars, and ECG-AI-Cox resulted in validation AUCs of 0.77, 0.73, and 0.84, respectively. ARIC-HF and FHS-HF yielded AUCs=0.76 and 0.74, respectively and CPH resulted in AUC=0.78. ECG-AI-Cox outperformed all other models. ECG-AI-Cox provided an AUC of 0.85 for HFrEF and 0.83 for HFpEF.ConclusionECG-AI utilizing ECGs provides better validated predictions when compared to HF risk calculators and the ECG feature model and also works well with HFpEF and HFrEF classification.
更多查看译文
关键词
heart failure,artificial intelligence model,artificial intelligence,ecg-based
AI 理解论文
溯源树
样例
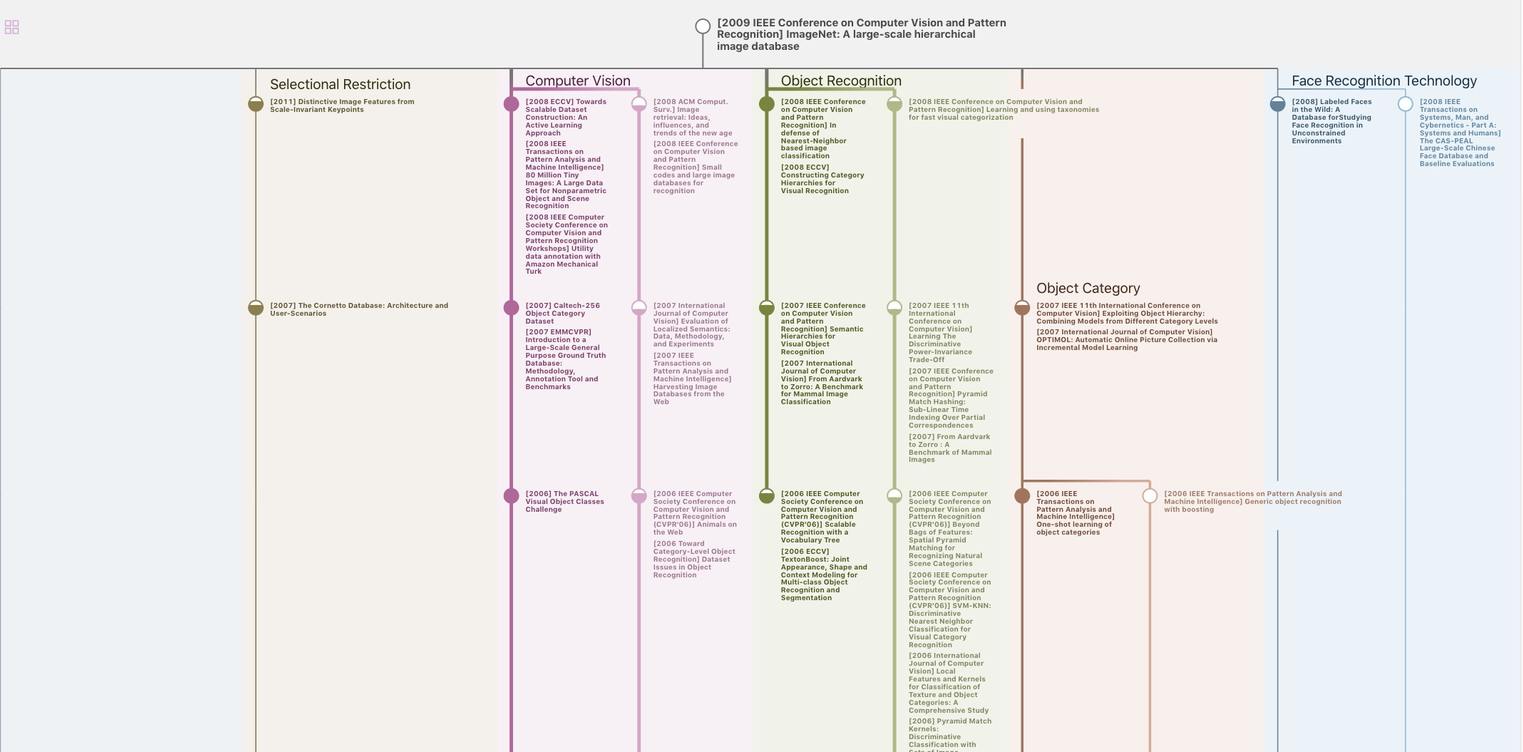
生成溯源树,研究论文发展脉络
Chat Paper
正在生成论文摘要