A novel deep belief network architecture with interval type-2 fuzzy set based uncertain parameters towards enhanced learning
FUZZY SETS AND SYSTEMS(2024)
摘要
This paper proposes a novel Deep Belief Network (DBN) architecture, the 'Interval Type-2 Fuzzy DBN (IT2FDBN)', which models the weights and biases with IT2 FSs. Thus, it introduces a novel algorithm for augmented deep leaning, which has the capability to address all the limitations of the classical DBN (CDBN) and T1 fuzzy DBN (T1FDBN). We comparatively evaluate the performance of the IT2FDBN by conducting experiments using the popular MNIST handwritten digit recognition datasets. Additionally, to demonstrate its robustness and generalization capabilities, we also conduct experiments taking two noisy variants of MNIST dataset, viz. the MNIST with AWGN (additive white Gaussian noise) and the MNIST with motion blur. We conduct extensive simulations by considering different combinations of nodes in the hidden layers of the DBN for better model selection. We thoroughly compare the results using well-known performance measures such as root mean square error (RMSE) and Error rate. We show that, in terms of RMSE values and error rates, the proposed IT2FDBN outperforms both T1FDBN and CDBN across all the three datasets. Further, we also provide the results of convergence, runtime-based comparison, and statistical analysis in support of our proposal.
更多查看译文
关键词
Deep neural networks,Deep belief networks,Restricted Boltzmann machine,Interval type-2 fuzzy sets,Contrastive divergence
AI 理解论文
溯源树
样例
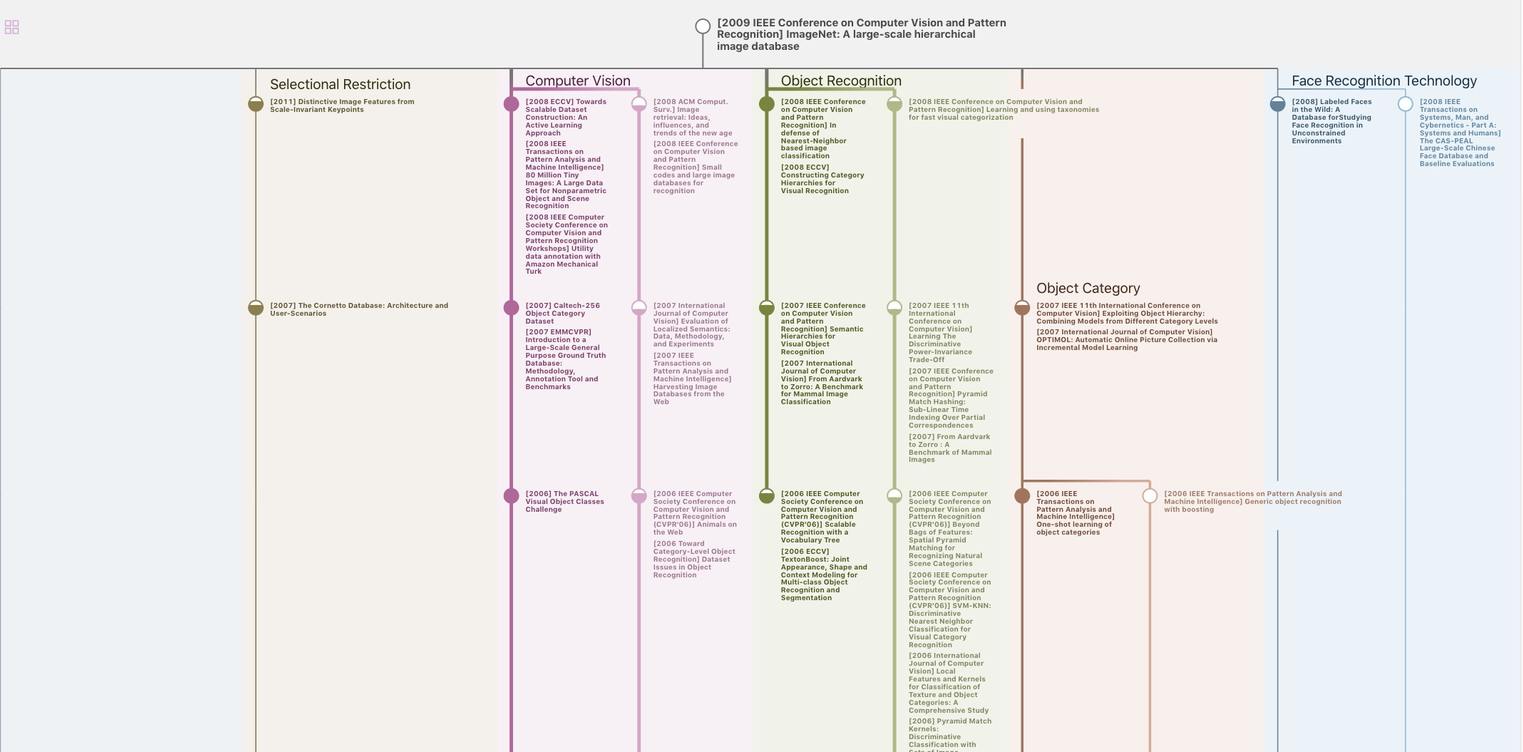
生成溯源树,研究论文发展脉络
Chat Paper
正在生成论文摘要