MC-VEO: A Visual-Event Odometry With Accurate 6-DoF Motion Compensation.
IEEE Trans. Intell. Veh.(2024)
摘要
Nowadays, robust and accurate odometries, as the foundation technology of navigation systems, gains significance in autonomous driving and robotic navigation fields. Although odometries, especially visual odometries (VOs), have made substantial progress, their application scenarios are still limited by the normal cameras' frame rate limitations and their low robustness to motion blur. The event camera, a recently proposed bionic sensor, seeks to tackle these challenges, offering new possibilities for VO solutions to overcome extreme environments. However, integrating event cameras into VO faces challenges like the RGB-event modality gap and the requirement for efficient event processing. To address these research gaps to some extent, we propose a novel visual-event odometry, namely MC-VEO (Motion Compensated Visual-Event Odometry). Specifically, by introducing the temporal Gaussian weight into the standard contrast maximization framework, we propose the first effective 6-DoF motion compensation method that generates deblurred event frames from event data without additional sensors. The generated frames then be aligned with the RGB images through Event Generation Model (EGM) in MC-VEO, so as to overcome the RGB-event modality gap. Additionally, during the optimization of the EGM-based motion estimation algorithm, our decoupling and pre-calculation, matrix representation and parallel solving further accelerate the per-point processing of events, which enables MC-VEO to show satisfactory speed performance even when facing large amounts of events and candidate points. The superior performance of MC-VEO is evaluated by both qualitative and quantitative experimental results. To ensure that our results are fully reproducible, all the relevant data and codes have been released publicly.
更多查看译文
关键词
Visual-Event Odometry,SLAM,Contrast Maximization,Motion Compensation,Data Fusion
AI 理解论文
溯源树
样例
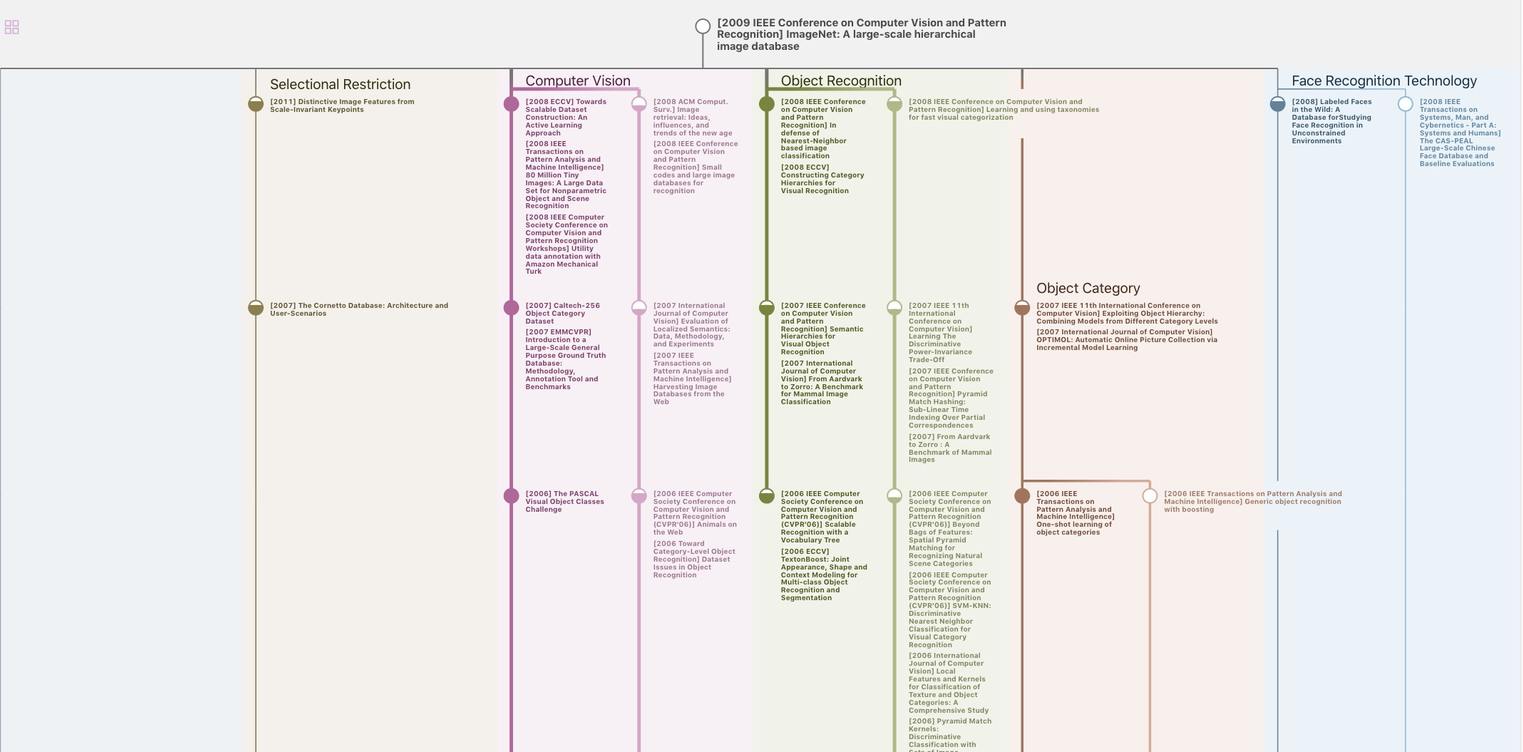
生成溯源树,研究论文发展脉络
Chat Paper
正在生成论文摘要