Efficient Multi-Task CNN for Face and Facial Expression Recognition Using Residual and Dense Architectures for Application in Monitoring Online Learning
INTERNATIONAL JOURNAL OF FUZZY LOGIC AND INTELLIGENT SYSTEMS(2023)
摘要
Multi-task convolutional neural networks (MTCNN) have garnered substantial attention for their impressive feats in image classification. However, MTCNNs tailored for the dual tasks of face recognition and facial expression recognition remain relatively scarce. These networks often entail complexity and substantial computational demands, rendering them unsuitable for practical systems constrained by computational limitations. Addressing this gap, the present paper proposes an efficient MTCNN model that capitalizes on cutting-edge architectures- residual networks and dense-connected networks-to adeptly execute face recognition and facial expression recognition tasks. The model's efficacy is rigorously assessed across well-known datasets (JAFFE, CK+, OuluCASIA, KDEF) as well as collected images from learners (HOUS22). The proposed model consistently attains high accuracy levels across all datasets, with a notable minimum accuracy of 99.55% on testing data. These outcomes stand as a testament to the model's remarkable performance, particularly in relation to its streamlined design. Moreover, the model is seamlessly integrated with an online learning management system, furnishing a versatile means of monitoring learning activities to enhance the overall training quality.
更多查看译文
关键词
Multi-task convolutional neural network,Residual networks,Dense-connected networks,Face recognition,Facial expressions recognition,Online learning management systems
AI 理解论文
溯源树
样例
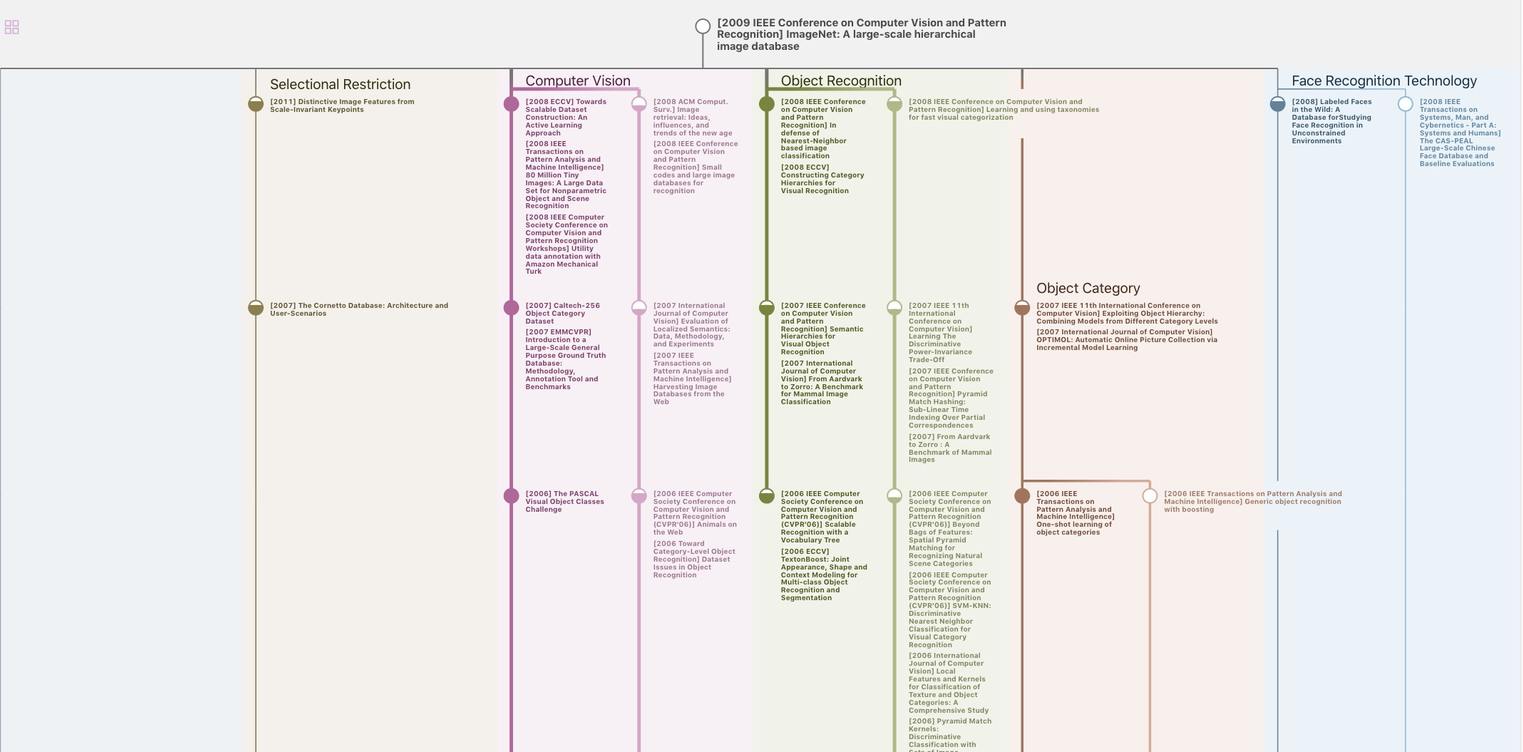
生成溯源树,研究论文发展脉络
Chat Paper
正在生成论文摘要