Features Effectiveness Verification Using Machine-Learning-Based GNSS NLOS Signal Detection in Urban Canyon Environment
Proceedings of the Satellite Division's International Technical Meeting(2023)
摘要
The GNSS positioning performance can be significantly degraded in urban canyon environments due to the multipath effect. However, the multipath error cannot be modeled or corrected accurately because it has no spatiotemporal correlation. Recently, machine learning models have shown the ability to learn potential relationships between data and are especially proficient in fitting nonlinear functions. Based on this statement, many researches regard the machine learning models as the most effective and promising tools to mitigate multipath errors, among which the most popular strategy is the classification of LOS (lineof-sight) and NLOS (non-line-of-sight) signals. Traditional machine learning models such as support vector machine (SVM), decision tree, deep learning models such as Multivariate long Short Term Memory-Fully Convolutional Network (MLSTMFCN) and Convolutional Neural Network (CNN) have been used to conduct relevant classification experiments. Pseudorange residuals, carrier-to-noise ratio and elevation are three most widely selected features as the input of models. However, the effectiveness of these features are rarely verified. In this paper, the most basic machine learning model SVM is used to classify LOS and NLOS signals and an average accuracy of 82.15% is achieved. The labels are given by a fisheye camera and six features are visualized and analyzed, which include carrier-to-noise ratio, elevation, azimuth in body frame, pseudorange residuals, pseudorange consistency and double differenced pseudorange. Finally, the kinetic single point positioning (SPP) with detected NLOS exclusion is conducted. The results reveal that, pseudorange residuals, as well as other features with the same distribution, may be unnecessary in LOS and NLOS classifications tasks. Also, the SPP positioning results reveal that NLOS exclusion is a useful and promising multipath mitigation strategy, although it is strongly dependent on considerable number of satellites. Compared with the original SPP method, the SVM-based NLOS exclusion achieves an accuracy improvement of 76.8%, 2.6% and 63.1% in east, north and up directions, respectively.
更多查看译文
关键词
gnss nlos signal detection,features effectiveness verification,urban canyon environment,machine-learning-based machine-learning-based
AI 理解论文
溯源树
样例
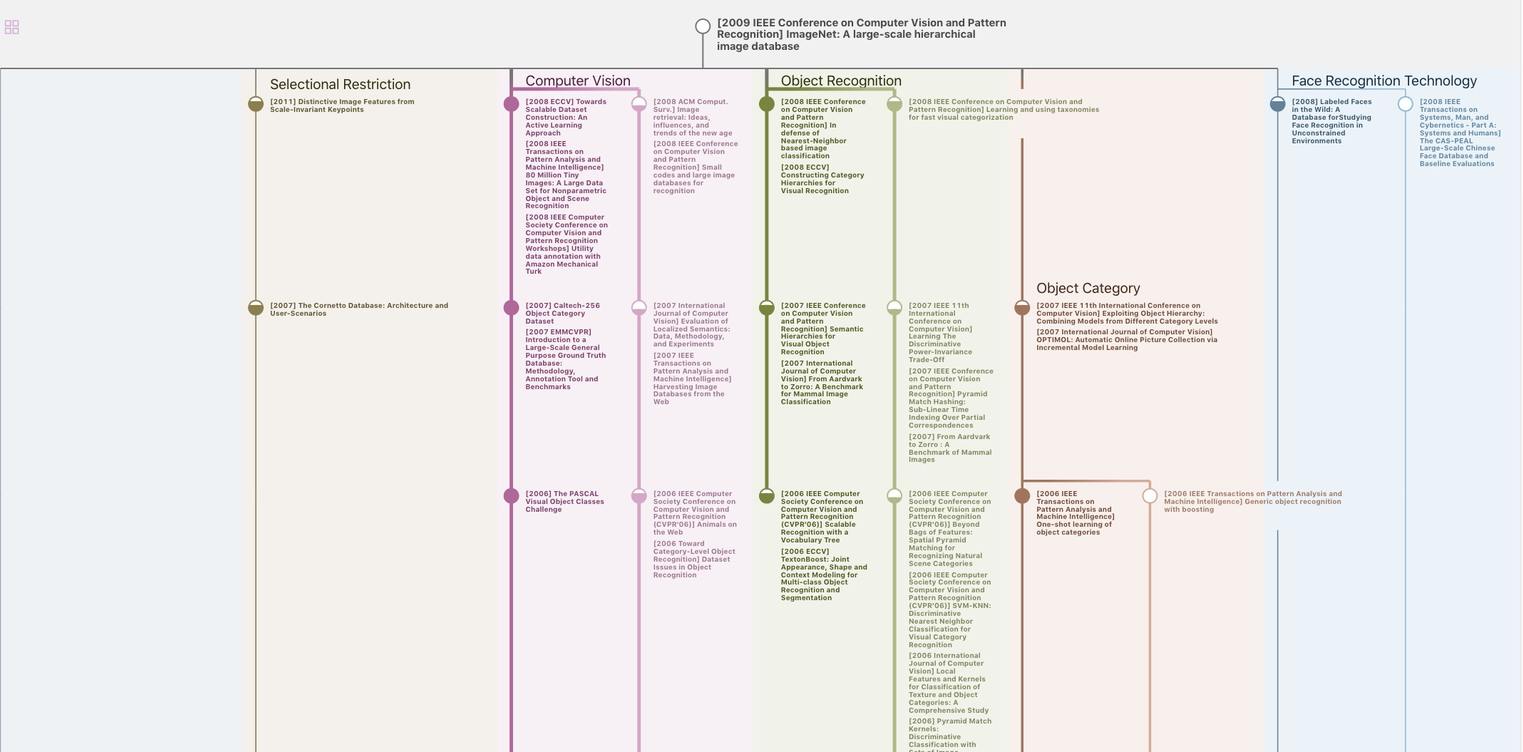
生成溯源树,研究论文发展脉络
Chat Paper
正在生成论文摘要