CLIP-VG: Self-Paced Curriculum Adapting of CLIP for Visual Grounding
IEEE TRANSACTIONS ON MULTIMEDIA(2024)
摘要
Visual Grounding (VG) is a crucial topic in the field of vision and language, which involves locating a specific region described by expressions within an image. To reduce the reliance on manually labeled data, unsupervised methods have been developed to locate regions using pseudo-labels. However, the performance of existing unsupervised methods is highly dependent on the quality of pseudo-labels and these methods always encounter issues with limited diversity. In order to utilize vision and language pre-trained models to address the grounding problem, and reasonably take advantage of pseudo-labels, we propose CLIP-VG, a novel method that can conduct self-paced curriculum adapting of CLIP with pseudo-language labels. We propose a simple yet efficient end-to-end network architecture to realize the transfer of CLIP to the visual grounding. Based on the CLIP-based architecture, we further propose single-source and multi-source curriculum adapting algorithms, which can progressively find more reliable pseudo-labels to learn an optimal model, thereby achieving a balance between reliability and diversity for the pseudo-language labels. Our method outperforms the current state-of-the-art unsupervised method by a significant margin on RefCOCO/+/g datasets in both single-source and multi-source scenarios, with improvements ranging from 6.78% to 10.67% and 11.39% to 14.87%, respectively. Furthermore, our approach even outperforms existing weakly supervised methods.
更多查看译文
关键词
Grounding,Reliability,Adaptation models,Task analysis,Visualization,Data models,Annotations,Visual grounding,curriculum learning,pseudo-language label,and vision-language models
AI 理解论文
溯源树
样例
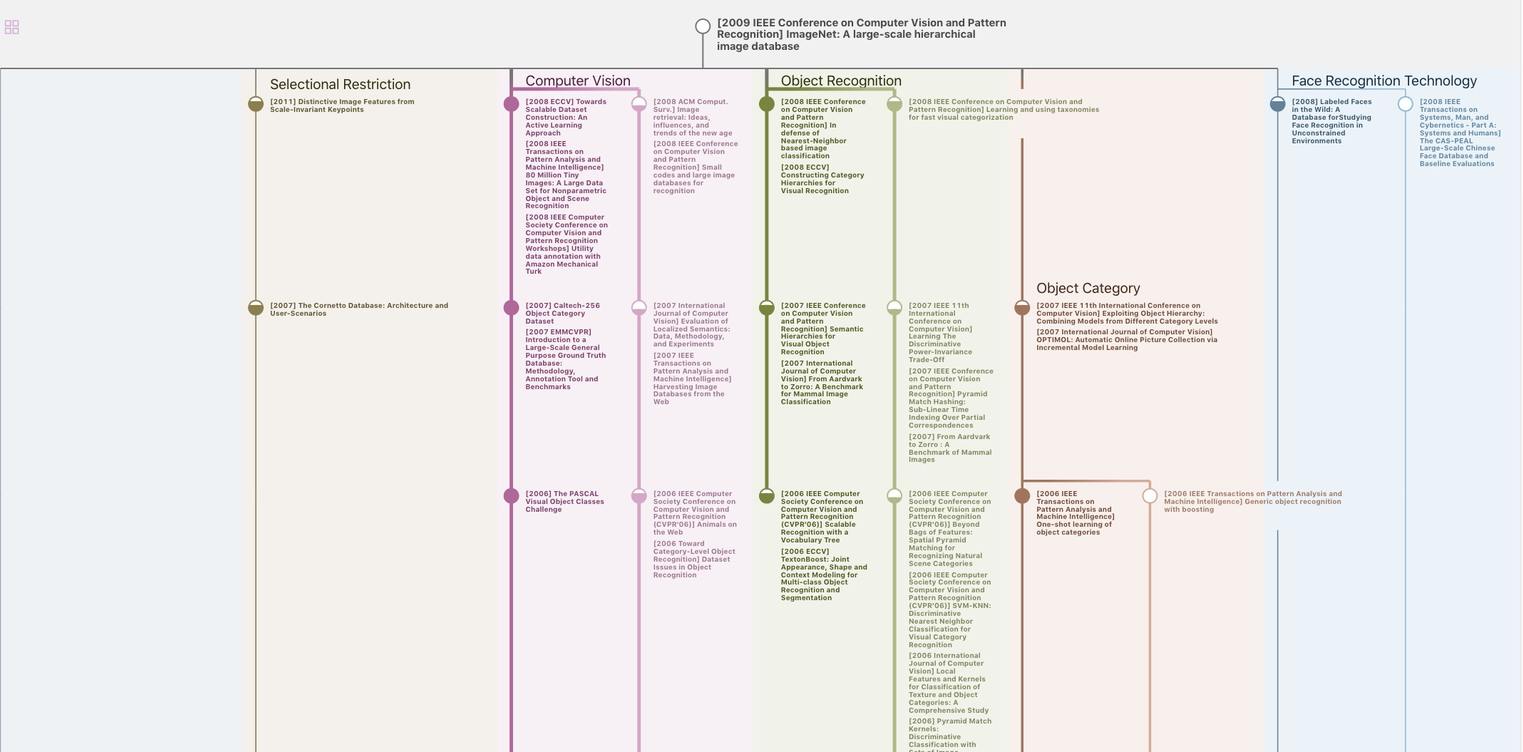
生成溯源树,研究论文发展脉络
Chat Paper
正在生成论文摘要