Area-wise relational knowledge distillation
COMMUNICATIONS FOR STATISTICAL APPLICATIONS AND METHODS(2023)
摘要
Knowledge distillation (KD) refers to extracting knowledge from a large and complex model (teacher) and transferring it to a relatively small model (student). This can be done by training the teacher model to obtain the activation function values of the hidden or the output layers and then retraining the student model using the same training data with the obtained values. Recently, relational KD (RKD) has been proposed to extract knowledge about relative di fferences in training data. This method improved the performance of the student model compared to conventional KDs. In this paper, we propose a new method for RKD by introducing a new loss function for RKD. The proposed loss function is defined using the area di fference between the teacher model and the student model in a specific hidden layer, and it is shown that the model can be successfully compressed, and the generalization performance of the model can be improved. We demonstrate that the accuracy of the model applying the method proposed in the study of model compression of audio data is up to 1.8% higher than that of the existing method. For the study of model generalization, we demonstrate that the model has up to 0.5% better performance in accuracy when introducing the RKD method to self-KD using image data.
更多查看译文
关键词
deep learning,model compression,knowledge distillation,audio signal processing,image processing
AI 理解论文
溯源树
样例
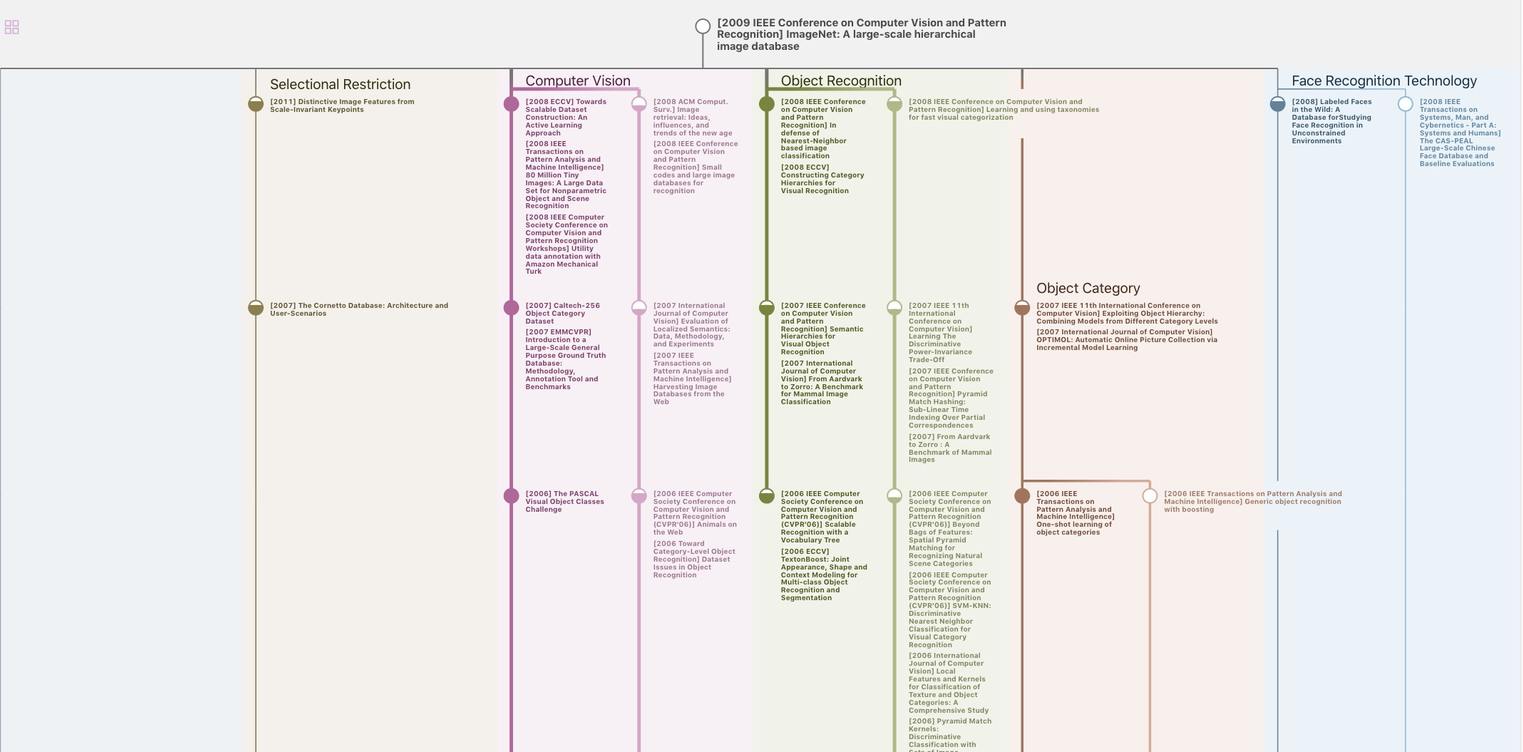
生成溯源树,研究论文发展脉络
Chat Paper
正在生成论文摘要