An early warning method for abnormal behavior of college students based on multimodal fusion and improved decision tree
JOURNAL OF INTELLIGENT & FUZZY SYSTEMS(2023)
摘要
With the development of the Internet and the informatization construction of universities, the massive data accumulated by "campus big data" presents problems such as discreteness and sparseness. Students with abnormal behaviors have become an urgent problem to be solved in student behavior analysis. This paper proposes an early warning method for abnormal behaviour of college students based on multimodal fusion and an improved decision tree (EWMABCS-MFIDT). First, given the insufficient representation of student behavioral portraits and the problems of timeliness and dynamics in behavioral labels, a student behavioral portrait based on the multimodal fusion method is proposed. Second, aiming at the timeliness and backwardness of abnormal behavior prediction, based on student behavior classification prediction, this paper proposes an improved decision tree-based early warning method for abnormal student behavior. Finally, we design a student behavior analysis and early warning framework under the campus big data environment. Taking the abnormal early warning of students' academic performance as an example, compared with other early warning algorithms, the EWMABCS-MFIDT method can improve the accuracy of early warning and make students' educational work more targeted, personalized, and predictive.
更多查看译文
关键词
Education big data,student behavior portrait,multimodal fusion,abnormal behavior early warning,improved decision tree
AI 理解论文
溯源树
样例
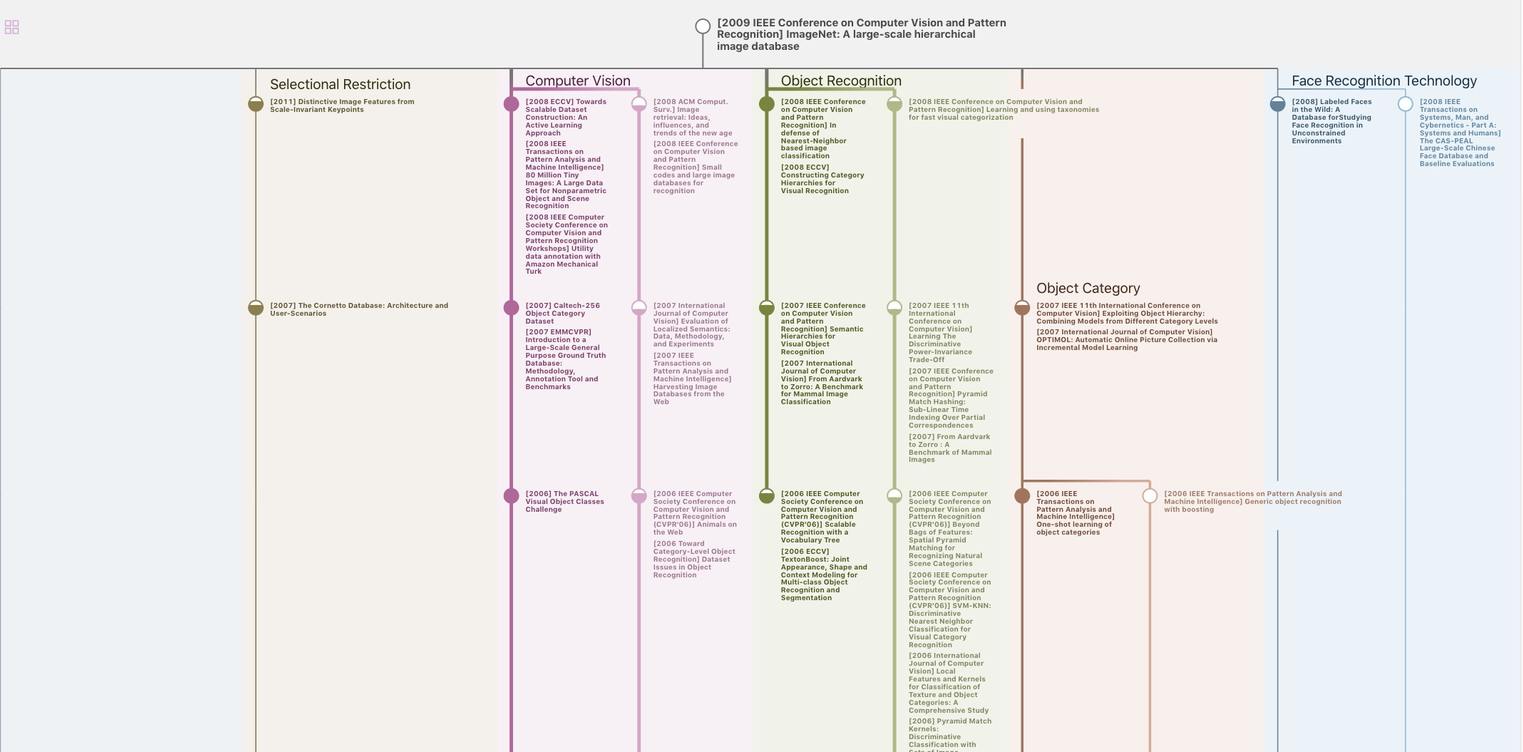
生成溯源树,研究论文发展脉络
Chat Paper
正在生成论文摘要