Efficient Edge-AI Models for Robust ECG Abnormality Detection on Resource-Constrained Hardware
medRxiv (Cold Spring Harbor Laboratory)(2023)
摘要
Abstract This study introduces two models, CLTC and CCfC, designed for abnormality identification using ECG data. Trained on the TNMG subset dataset, both models were evaluated for their performance, generative capacity, and resilience. They demonstrated comparable results in terms of F1 scores and AUROC values. The CCfC model achieved slightly higher accuracy, while the CLTC model showed better handling of empty channels. Remarkably, the models were successfully deployed on a resource-constrained microcontroller, proving their suitability for edge device applications. Generalization capabilities were confirmed through the evaluation of the CPSC dataset. The models’ efficient resource utilization, occupying 70.6% of total storage and 9.4% of flash memory, makes them promising candidates for real-world healthcare applications. Overall, this research advances abnormality identification in ECG data, contributing to the progress of AI in healthcare.
更多查看译文
关键词
robust ecg abnormality detection,resource-constrained
AI 理解论文
溯源树
样例
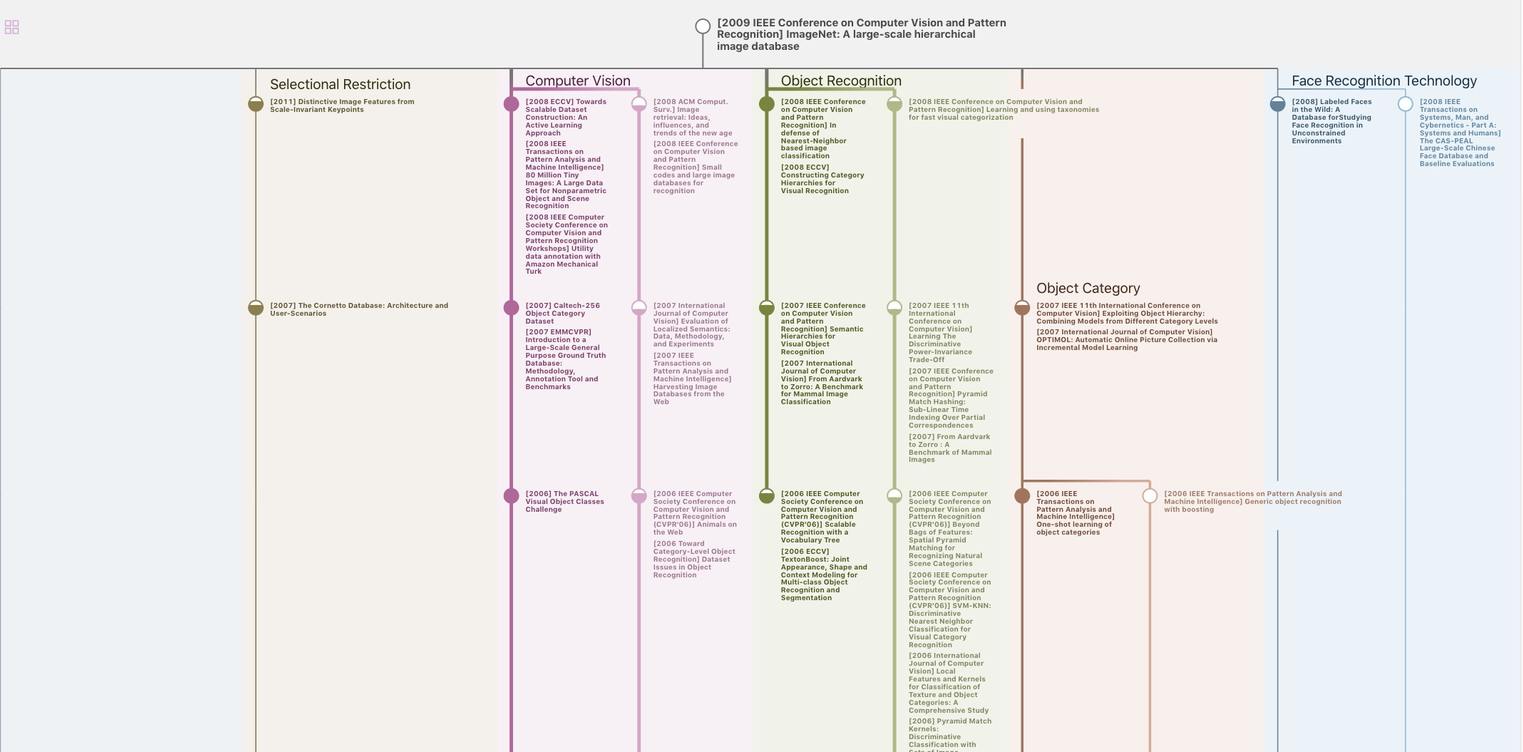
生成溯源树,研究论文发展脉络
Chat Paper
正在生成论文摘要