Non-parametric Hierarchical Bayesian Modeling of the Contrast Sensitivity Function
Journal of Vision(2023)
摘要
The qCSF has been used to assess spatial vision in normal and clinical populations. Because it uses the log parabola CSF model, the estimated contrast sensitivities (CSs) across different spatial frequencies (SFs) are not independent. Most studies have used the area under Log CSF (AULCSF) to simplify statistical inference. Here, we developed a hierarchical Bayesian model (HBM) with population, individual and test levels to compute the joint posterior CS distribution across all levels and SFs to enable statistical inference on CS at individual SFs, using covariance hyperparameters at the population and individual levels to capture the relationship between CSs across SFs. We applied the HBM to a dataset of 112 subjects tested with qCSF trials in each of three (L, M & H) luminance conditions (Hou et al., 2016). The HBM recovered correlations between CSs in pairs of 19 SFs (0.739 to 0.948, -0.065 to 0.451, and -0.044 to 0.340 at the population, individual and test levels, respectively), and precise CS estimates at individual SFs for each subject (average 68.2% HWCI=0.097 log10 units). CS difference distributions between luminance conditions were constructed from the joint posterior distribution. At the group level, luminance had highly significant effects on CS both across 19 SFs jointly and at each SF (all p<0.001). 112, 111, and 71 subjects exhibited significant luminance effects on CS across 19 SFs jointly in L-H, L-M, and H-M, respectively (p< 0.05), whereas 112, 112, and 101 subjects exhibited significant luminance effects in at least two SFs (p<0.05). With covariances, the HBM generated precise CS estimates at individual SFs for each subject based on only 150 optotypes in each condition. The joint posterior distribution from the HBM enabled statistical inference on CS at individual SFs and across SFs at both group and subject levels.
更多查看译文
关键词
contrast sensitivity function,contrast sensitivity,non-parametric
AI 理解论文
溯源树
样例
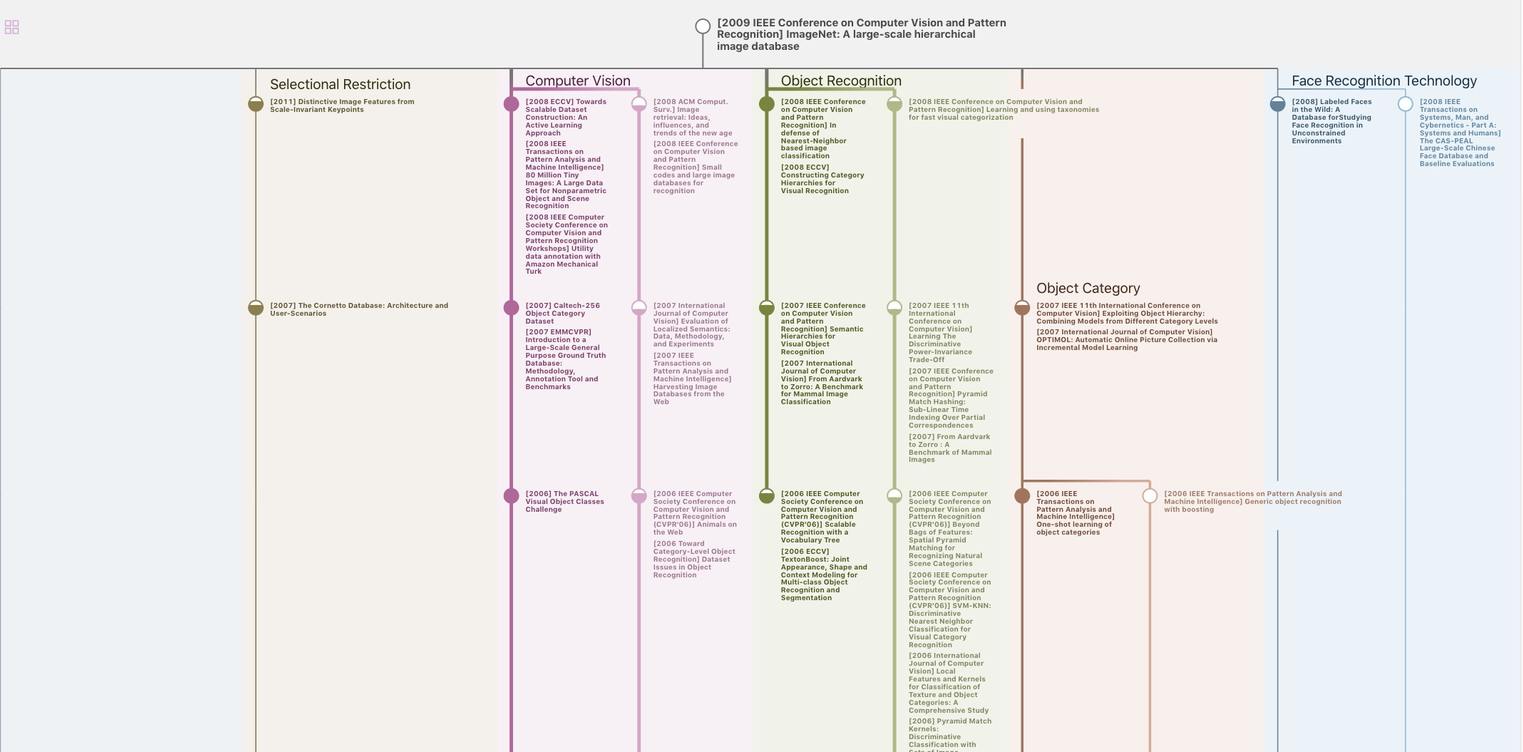
生成溯源树,研究论文发展脉络
Chat Paper
正在生成论文摘要