A Convolutional Neural Network with Meta-feature Learning for Wireless Capsule Endoscopy Image Classification
Journal of Medical and Biological Engineering(2023)
摘要
Purpose Wireless Capsule Endoscopy is a widely used method for gastrointestinal tract inspection. Automatic gastrointestinal abnormality detection systems face a key issue of intra-class variance, especially in binary classification tasks. Due to visual and shape disparity in abnormalities, this problem arises. This difficulty can be overcome with a system that may be able to characterize patterns of abnormality in different aspects. Methods In this study, a convolutional neural network (CNN) is proposed for abnormality detection that extracts a rich pool of meaningful features from gastrointestinal images using diverse convolution operations. It consists of three CNNs running in parallel, each of which is capable of learning a unique characteristic. While the first network operates using depthwise separable convolution that helps in extracting a variety of features, the second network exploits cosine normalized convolution which makes the model robust to inputs with high variations. Relative patterns from the rich pool of variegated features from these couple of networks are extracted through a novel meta-feature extraction technique introduced in the third network that finds statistical co-relation within features. Results The proposed hybrid CNN model is trained and tested on two widely accepted datasets. Outcomes demonstrate that the proposed model outperforms six state-of-the-art methods with the highest AUC of 96% and 98% on KID and Kvasir-Capsule datasets, respectively. Conclusion This trio of CNNs adeptly manages intra-class variability and proficiently categorizes gastrointestinal images as either normal or abnormal. The model's unique`approach for feature learning leads to superior performance compared to existing methodologies.
更多查看译文
关键词
Attention mechanism,CNN,Feature extraction,Statistical features,Wireless capsule endoscopy,Anomaly detection
AI 理解论文
溯源树
样例
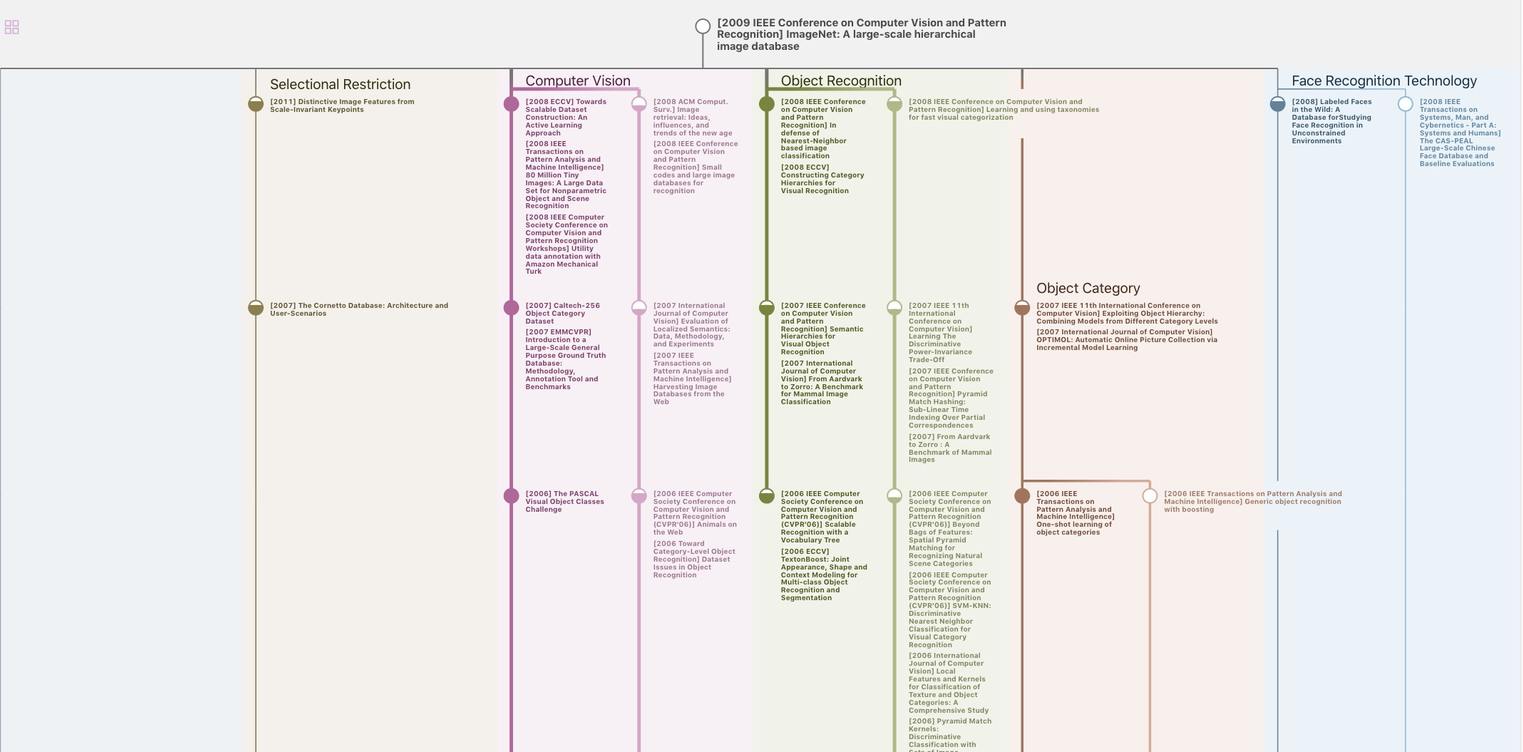
生成溯源树,研究论文发展脉络
Chat Paper
正在生成论文摘要