Pretreatment computed tomography-defined sarcopenia, treatment-associated muscle loss and survival in cervical cancer: a systematic review and meta-analysis
Authorea (Authorea)(2023)
摘要
Background: Sarcopenia has been identified as a predictor of poor prognosis in various types of cancer. However, the impact of pretreatment sarcopenia and the reduction of skeletal muscle mass during treatment on prognosis of patients with cervical cancer is still not well-understood. Objectives: To investigate the association between sarcopenia and prognosis in patients with cervical cancer. Search Strategy: Studies in PubMed, EMBASE, Web of Science, and Cochrane databases were searched. Selection Criteria: Studies exploring the relationship between computed tomography (CT)-defined sarcopenia and the prognosis of cervical cancer were included. Data Collection and Analysis: Our primary outcome was overall survival (OS), secondary outcome was progression-free survival (PFS). The pooled hazard ratio (HR) and 95% confidence interval (CI) of OS and PFS were analyzed by R software. The studies’ quality was assessed using the Quality in Prognostic Studies tool. Main Results: 12 studies involving 1593 patients with cervical cancer were included in the analysis, with a prevalence of sarcopenia ranging from 24.8%-55.0%. Meta-analysis results showed that sarcopenia was an independent predictor of poor OS (HR 1.70, 95% CI = 1.31-2.22; p <0.01) and PFS (HR 1.35, 95% CI = 1.04-1.74; p =0.02) in patients with cervical cancer. Skeletal muscle loss during treatment was significantly associated with a short OS time (HR, 4.00; 95% CI=2.63–6.08, p <0.01). Conclusions: The prevalence of pretreatment sarcopenia was high among patients with cervical cancer. Pretreatment sarcopenia and loss of skeletal muscle during the treatment all had a negative effect on the prognosis in cervical cancer.
更多查看译文
关键词
sarcopenia,cervical cancer,muscle loss,tomography-defined,treatment-associated,meta-analysis
AI 理解论文
溯源树
样例
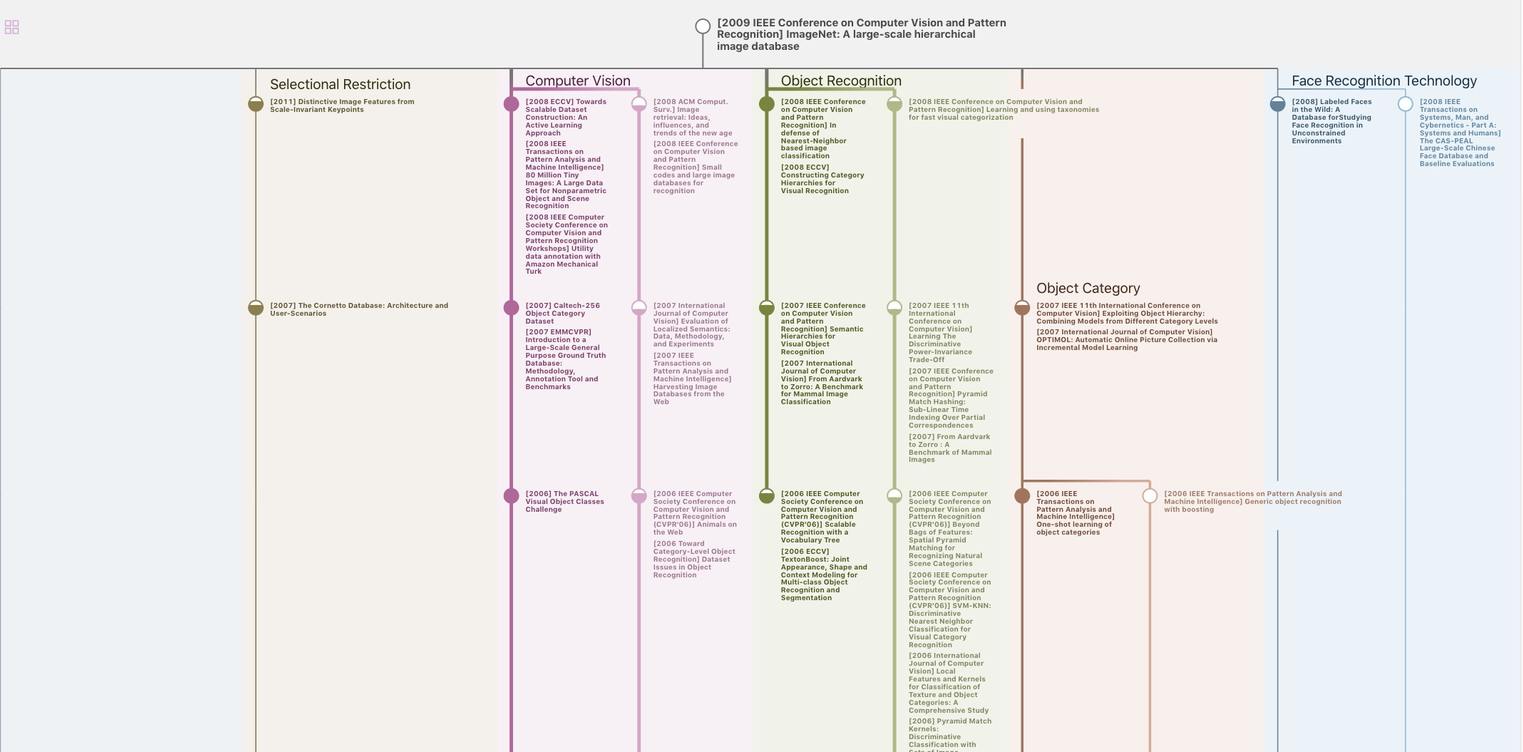
生成溯源树,研究论文发展脉络
Chat Paper
正在生成论文摘要