Enhancing Educational Dialogue Act Classification With Discourse Context and Sample Informativeness.
IEEE Transactions on Learning Technologies(2024)
摘要
Automating the classification of instructional strategies from a large-scale online tutorial dialogue corpus is indispensable to the design of dialogue-based intelligent tutoring systems. Despite many existing studies employing supervised machine learning (ML) models to automate the classification process, they concluded that building a well-performed ML model is nontrivial since the sample size is commonly limited for model training. Based on this fact, we posited that the model performance can be further optimized by the design of input features and the selection of informative instances for model training. By reviewing the existing dialogue-related research, we found that contextual information (i.e., the content of preceding utterances) was a potential feature in many text classification tasks but underexplored in the classification of an instructional strategy. In addition, training a well-performed ML model (e.g., deep neural network) to recognize instructional strategies usually requires a large amount of manually annotated data, which are labor intensive. To alleviate the demand of manual annotation, researchers proposed the use of statistical active learning methods to select informative instances, but this method was rarely used for recognizing the instructional strategy in online tutoring dialogue. Therefore, our study aimed to investigate the improvement of automating the classification of dialogue acts—a popular approach to the detection of instructional strategies—from two perspectives. First, we explored whether and to what extent the incorporation of contextual information can boost a model's prediction performance. Then, we investigated the extent to which the recent active learning methods can alleviate the labor-intensive issues in training the ML model for recognizing the instructional strategies. Our study showed that: 1) the ML models trained on the features that included the contextual information achieved better performance than that of the models excluding it; 2) the effectiveness of the contextual information decayed after the ML model achieved an optimal performance; and 3) compared with the random baseline, active learning methods can select informative samples from the training dataset to train ML models, which can alleviate the labor-intensive issues.
更多查看译文
关键词
Active learning (AL),dialogue acts (DAs),discourse context,educational dialogue analysis,intelligent tutoring systems (ITSs)
AI 理解论文
溯源树
样例
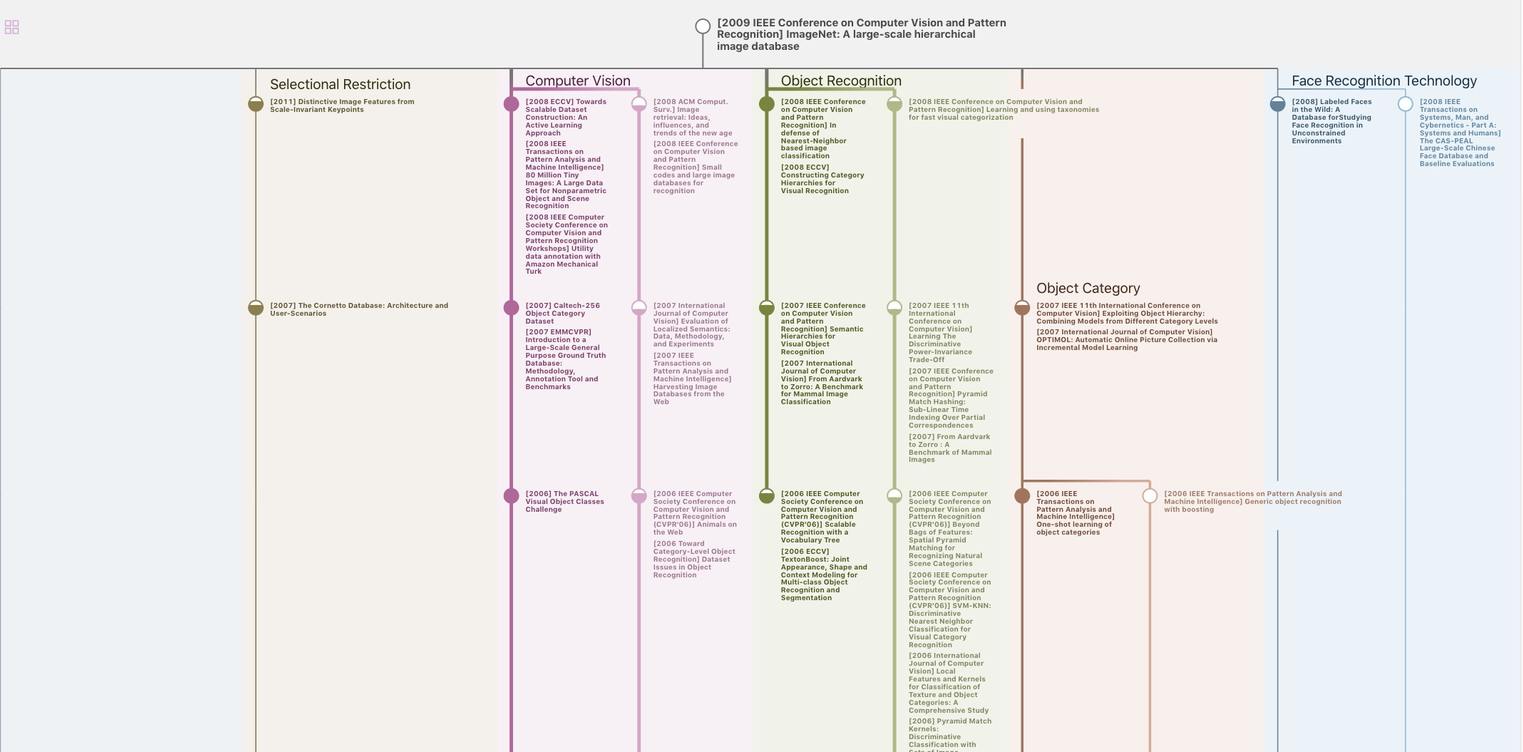
生成溯源树,研究论文发展脉络
Chat Paper
正在生成论文摘要