Moreau-Yoshida Variational Transport: A General Framework For Solving Regularized Distributional Optimization Problems
arXiv (Cornell University)(2023)
摘要
We consider a general optimization problem of minimizing a composite objective functional defined over a class of probability distributions. The objective is composed of two functionals: one is assumed to possess the variational representation and the other is expressed in terms of the expectation operator of a possibly nonsmooth convex regularizer function. Such a regularized distributional optimization problem widely appears in machine learning and statistics, such as proximal Monte-Carlo sampling, Bayesian inference and generative modeling, for regularized estimation and generation. We propose a novel method, dubbed as Moreau-Yoshida Variational Transport (MYVT), for solving the regularized distributional optimization problem. First, as the name suggests, our method employs the Moreau-Yoshida envelope for a smooth approximation of the nonsmooth function in the objective. Second, we reformulate the approximate problem as a concave-convex saddle point problem by leveraging the variational representation, and then develope an efficient primal-dual algorithm to approximate the saddle point. Furthermore, we provide theoretical analyses and report experimental results to demonstrate the effectiveness of the proposed method.
更多查看译文
关键词
regularized distributional optimization problems,transport,moreau-yoshida
AI 理解论文
溯源树
样例
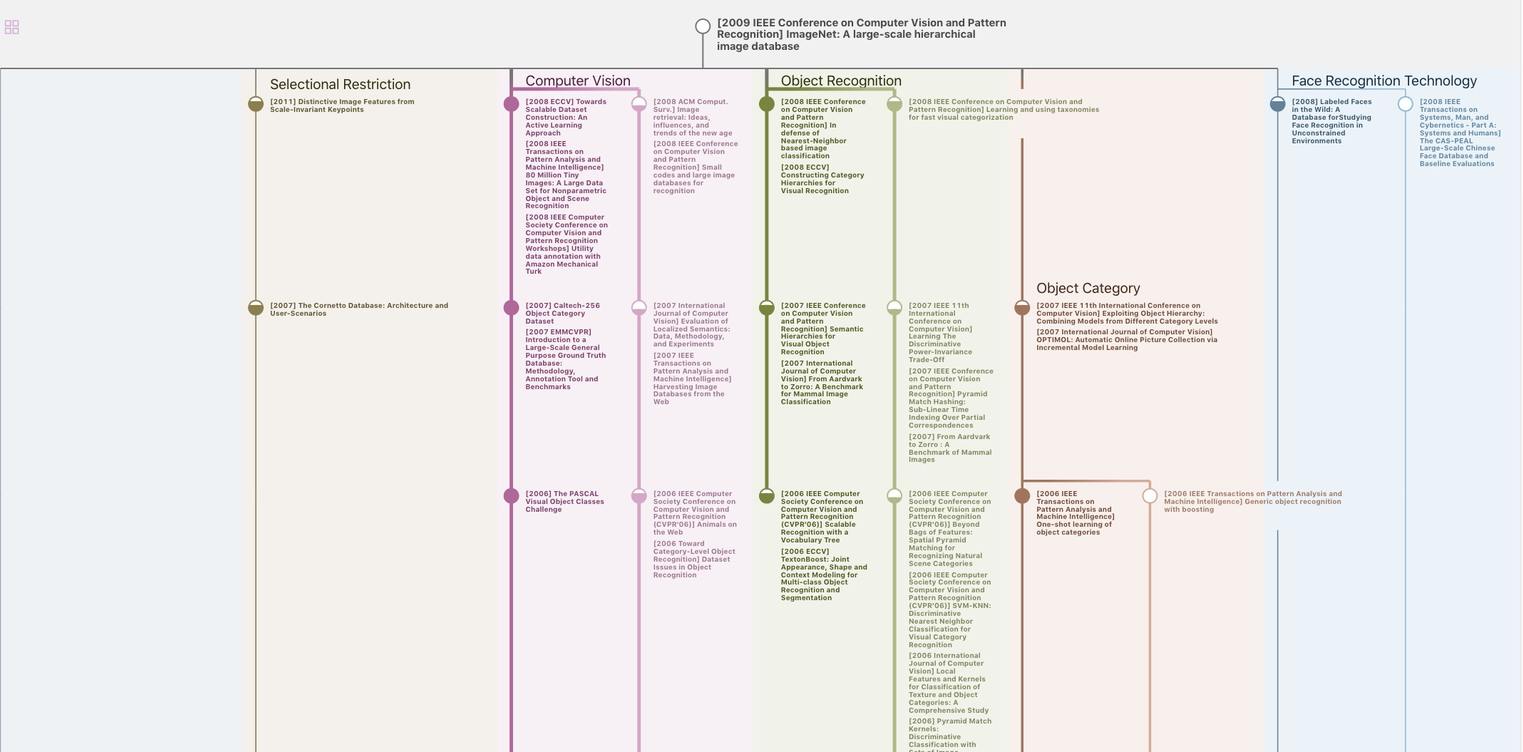
生成溯源树,研究论文发展脉络
Chat Paper
正在生成论文摘要