PhaCIA-TCNs: Short-Term Load Forecasting Using Temporal Convolutional Networks With Parallel Hybrid Activated Convolution and Input Attention
IEEE TRANSACTIONS ON NETWORK SCIENCE AND ENGINEERING(2024)
摘要
Temporal convolution networks (TCNs) are recently proposed to be used in the short-term load forecasting (STLF) tasks in modern smart grids, however, TCNs have two shortcomings, i.e., redundant convolutional operation and equal input importance problems. Therefore, we propose a novel TCN-based backbone model, called PhaCIA-TCNs, to achieve a more accurate short-term load forecasting, where parallel hybrid activated convolution (PhaC) and input attention (IA) are proposed to resolve the above problems, respectively. Specifically, IA is proposed to highlight important input elements while depressing irrelevant ones, which thus rises the model's forecasting accuracies but also brings additional time-cost; then PhaC is further proposed to remedy the efficiency problem and to further enhance the forecasting accuracies by shortening the convolutional learning path to overcome the redundant convolutional operation problem. Extensive experimental results show that i) PhaCIA-TCNs significantly outperform all state-of-the-art RNN-based and TCNs-based baselines in forecasting-error-based evaluation metrics on all datasets; ii) ablation studies show that PhaC and IA are both effective and essential for PhaCIA-TCN to achieve the superior forecasting accuracies in STLF tasks, and by integrating IA and PhaC with TCN, the proposed PhaCIA-TCN not only greatly outperforms TCN in forecasting accuracies but also keeps similar (sometimes even better) learning efficiency.
更多查看译文
关键词
Predictive models,Forecasting,Convolution,Task analysis,Load modeling,Load forecasting,Computational modeling,Short-term load forecasting,temporal convolution networks,input attention,hybrid convolution
AI 理解论文
溯源树
样例
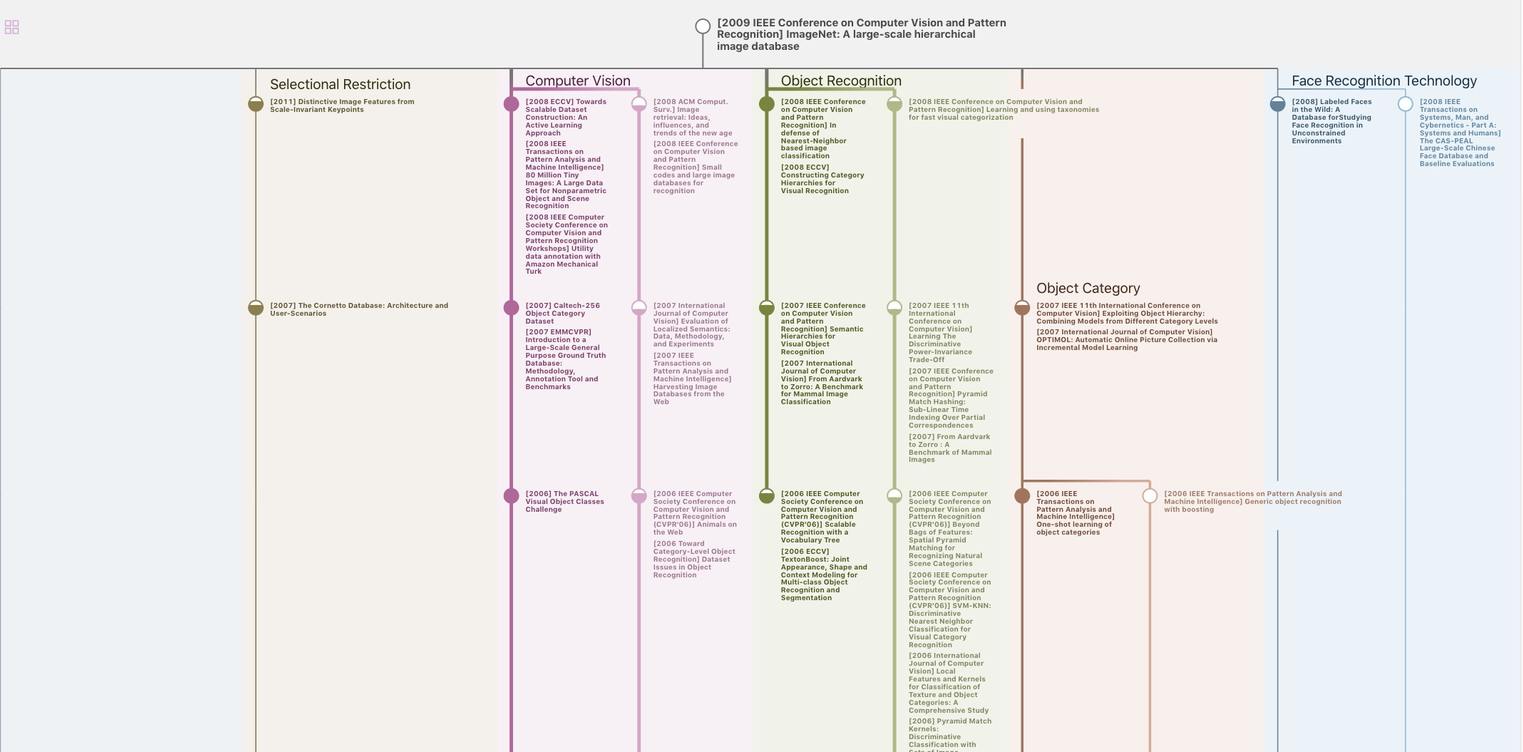
生成溯源树,研究论文发展脉络
Chat Paper
正在生成论文摘要