A Lightweight and Efficient IoT Intrusion Detection Method Based on Feature Grouping
IEEE INTERNET OF THINGS JOURNAL(2024)
摘要
Internet of Things (IoT) devices have been widely used in many fields, bringing many conveniences to people's life. With the massive deployment and application of IoT devices, how to maintain the IoT from cyber-attacks has become one of the major concerns of researchers. Due to IoT devices' limited computational capabilities and storage resources, IoT usually does not have sufficient security defense mechanisms, making it vulnerable to malware or device attacks. However, existing IoT-oriented intrusion detection systems usually only support the detection of specific malicious attacks or require complex models and massive computational resources to obtain high detection accuracy. We propose a lightweight and efficient intrusion detection method based on feature grouping to address the above challenges. We first design a fast protocol parsing method on the raw packet capture files to generate semantic-level parsing features. Then, we propose session merging and feature grouping methods. Finally, we verify the proposed features' effectiveness and analyze the malicious attacks' working process. The proposed method achieves more than 99.5% classification accuracy on three public IoT data sets. The proposed method requires significantly fewer computational resources than baseline methods in the protocol parsing and model training process. Experimental results show that the proposed method is lightweight, efficient, and extensible. Therefore, the proposed method is suitable for IoT intrusion detection.
更多查看译文
关键词
Attack process analysis,Internet of Things (IoT) malware,machine learning (ML) algorithms,network traffic classification,semantic-level features
AI 理解论文
溯源树
样例
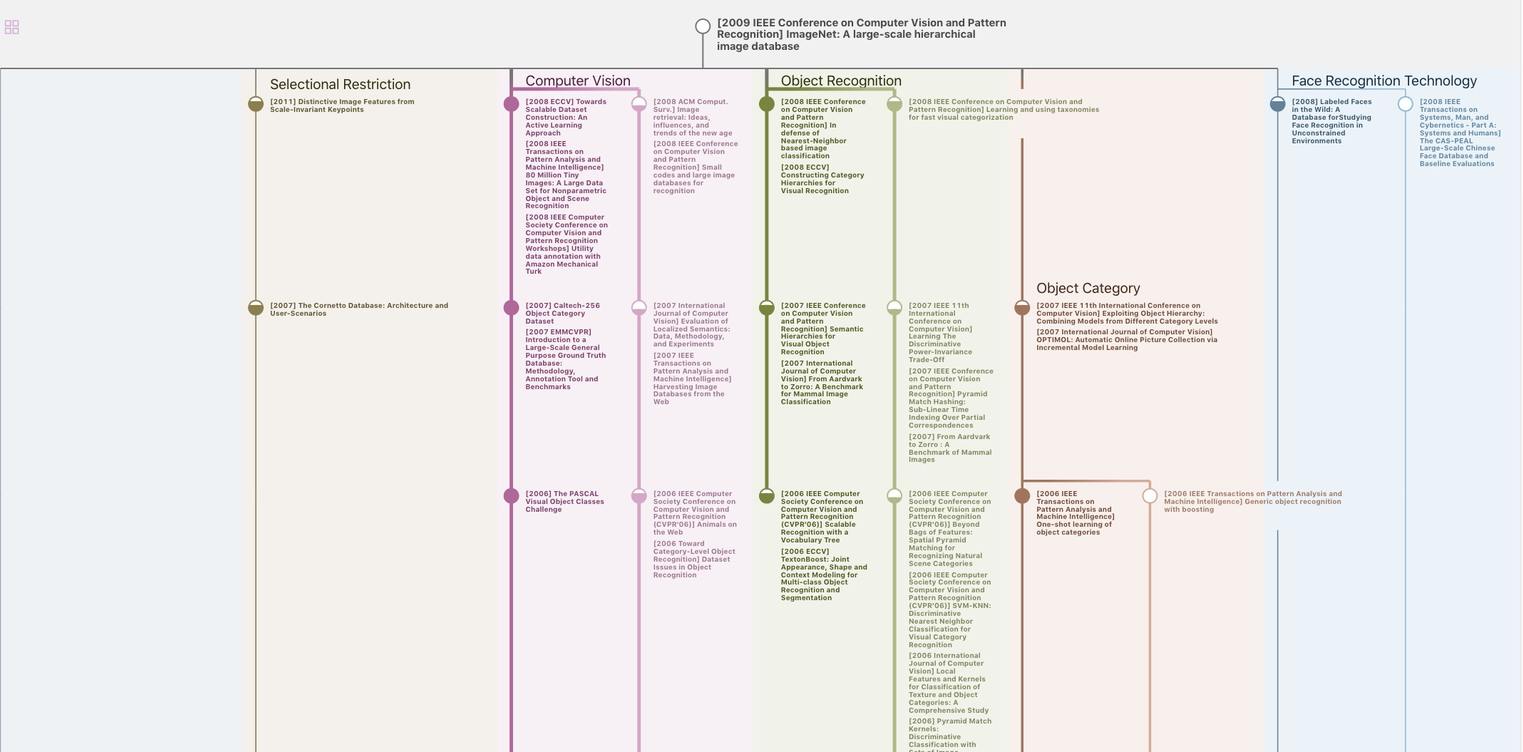
生成溯源树,研究论文发展脉络
Chat Paper
正在生成论文摘要