Improved Continually Evolved Classifiers for Few-Shot Class-Incremental Learning
IEEE TRANSACTIONS ON CIRCUITS AND SYSTEMS FOR VIDEO TECHNOLOGY(2024)
摘要
Few-shot class-incremental learning (FSCIL) aims to continually learn new classes using a few samples while not forgetting the old classes. The scarcity of new training data will seriously destroy the model's stability and plasticity. Continually Evolved Classifiers (CEC) (Zhang et al., 2021), a kind of framework, maintains the stability by freezing the encoder and achieves the plasticity by evolving the classifier along with a pseudo incremental learning scheme. However, the performance of CEC is limited due to 1) inequitable information gains between classifier weights and test features, and 2) inefficient learning task construction strategy. To address the first issue, we propose a Knowledge-guided Relation Refinement Module (KRRM) to update both the classifier weights and test features. The main function of KRRM is achieved through cross-attention to propagate the knowledge represented by old encoded data. To address the second issue, we design a Pseudo Incremental relation Refinement Learning (PIRL) that utilizes a novel hard concepts mining strategy to mine hard concept tasks globally and locally. By successfully addressing the two issues, our proposed method, named Improved Continually Evolved Classifiers (CEC+), extends the potential of CEC without introducing any additional parameters. More precisely, extensive experiments on CIFAR100, miniImageNet, and Caltech-UCSD Birds-200-2011, demonstrate that our proposed method surpasses prior state-of-the-art methods.
更多查看译文
关键词
Lifelong learning,few-shot class-incremental learning,image recognition,cross-attention mechanism
AI 理解论文
溯源树
样例
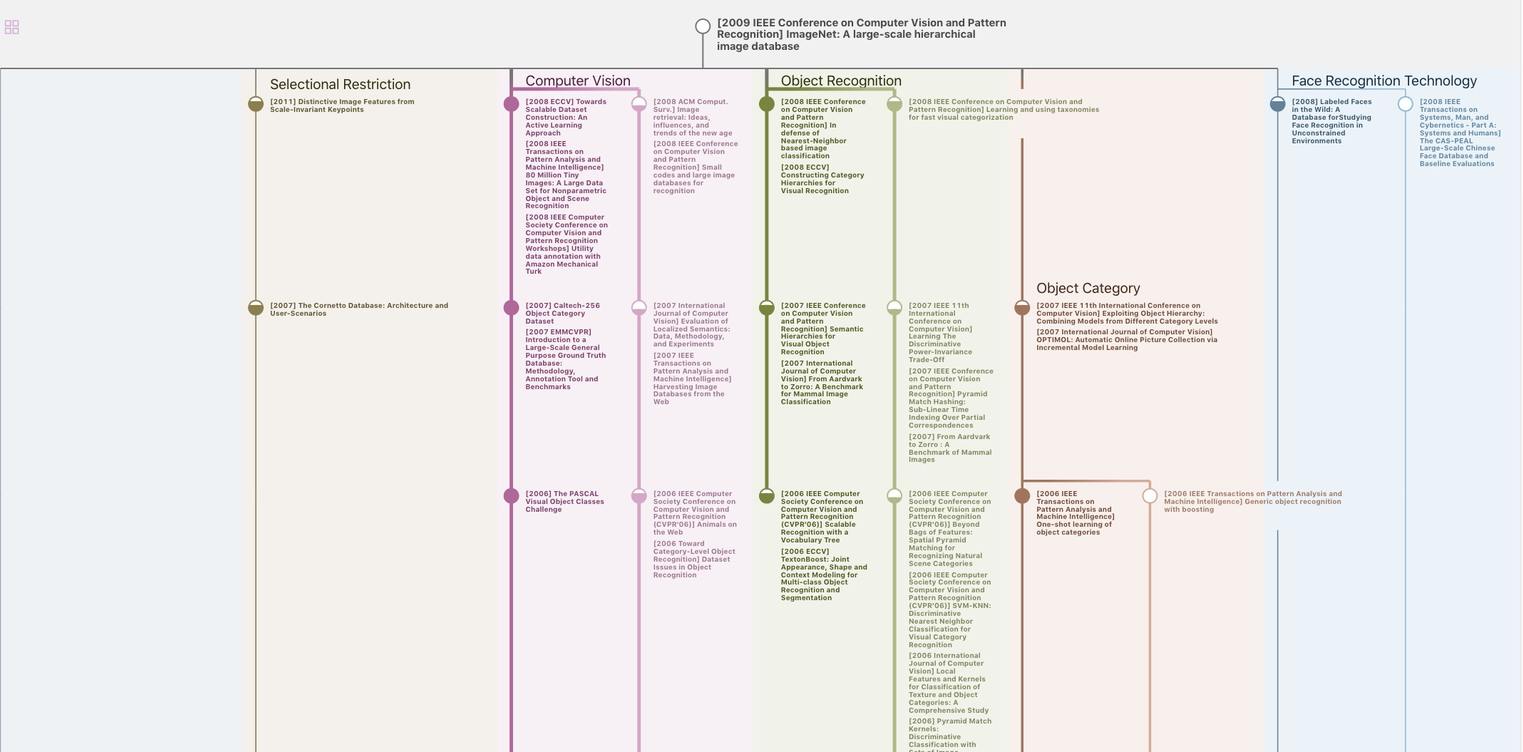
生成溯源树,研究论文发展脉络
Chat Paper
正在生成论文摘要