Exploring natural language processing in mechanical engineering education: Implications for academic integrity
INTERNATIONAL JOURNAL OF MECHANICAL ENGINEERING EDUCATION(2024)
摘要
In this paper, the authors review extant natural language processing models in the context of undergraduate mechanical engineering education. These models have advanced to a stage where it has become increasingly more difficult to discern computer vs. human-produced material, and as a result, have understandably raised questions about their impact on academic integrity. As part of our review, we perform two sets of tests with OpenAI's natural language processing model (1) using GPT-3 to generate text for a mechanical engineering laboratory report and (2) using Codex to generate code for an automation and control systems laboratory. Our results show that natural language processing is a potentially powerful assistive technology for engineering students. However, it is a technology that must be used with care, given its potential to enable cheating and plagiarism behaviours given how the technology challenges traditional assessment practices and traditional notions of authorship.
更多查看译文
关键词
Academic integrity,artificial intelligence,engineering,engineering education,academic misconduct,GPT-3,teaching,learning
AI 理解论文
溯源树
样例
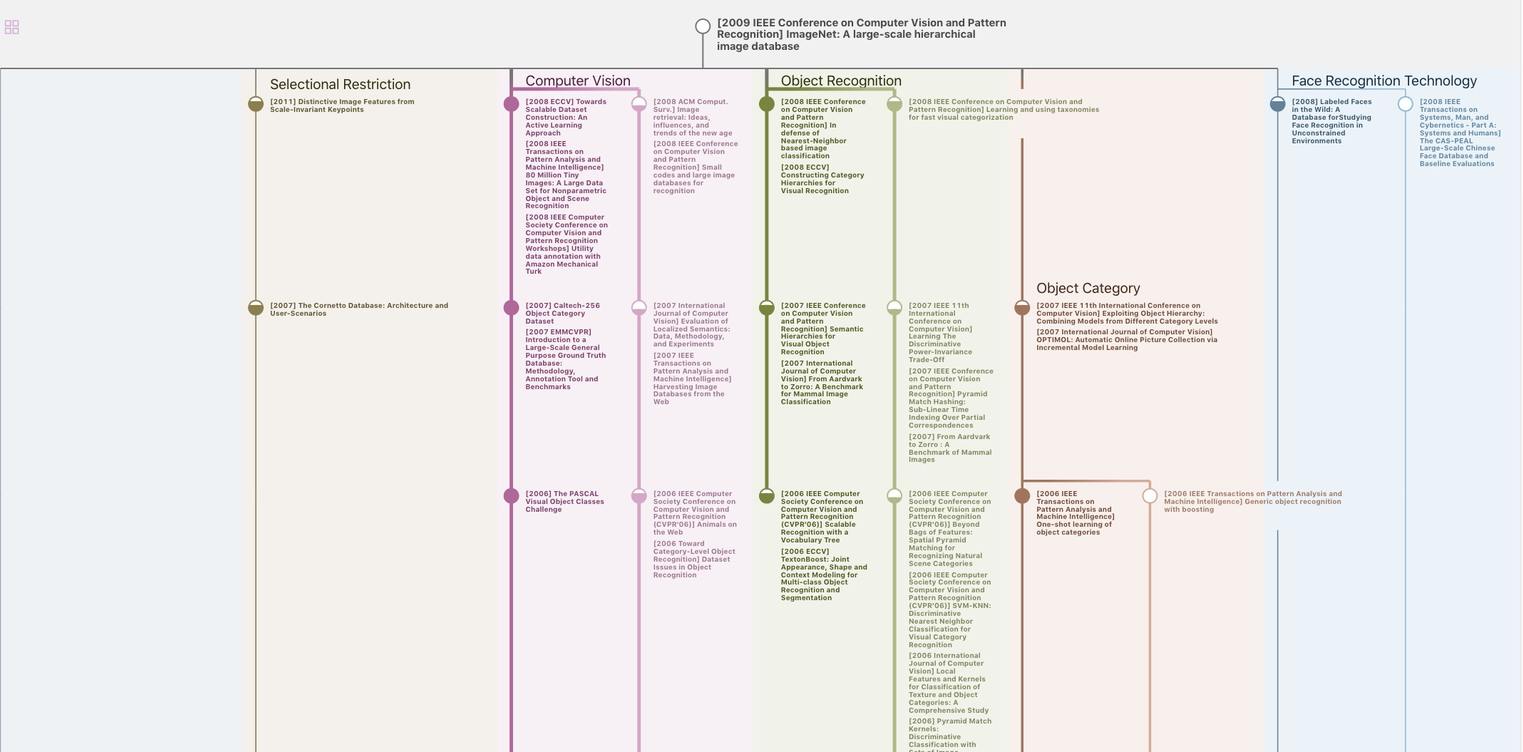
生成溯源树,研究论文发展脉络
Chat Paper
正在生成论文摘要