Parameterized Interval-Valued Aggregation Functions in Classification of Data with Large Number of Missing Values
Lecture notes in networks and systems(2023)
摘要
In this contribution parameterized interval-valued aggregation functions are applied in an algorithm based on k nearest neighbours algorithm which uses interval modelling to improve the quality of binary classification in the case of large number of missing values in datasets. Missing values are common in real-life problems and as a result performance of classifiers may be lowered significantly. One of the approach to cope with this problem is to use diverse methods of filling missing values. In this contribution we proposed to apply a kind of multiple imputation method involved in interval modelling approach. The considered interval-valued aggregation functions belong to diverse families of aggregations considered with respect to monotonicity conditions based on classical partial order for intervals and also other comparability relations for intervals. Moreover, these interval-valued aggregation functions are defined with diverse parameters which makes possibility to fit a given aggregation to the data and considered algorithm.
更多查看译文
关键词
classification,aggregation,values,data,interval-valued
AI 理解论文
溯源树
样例
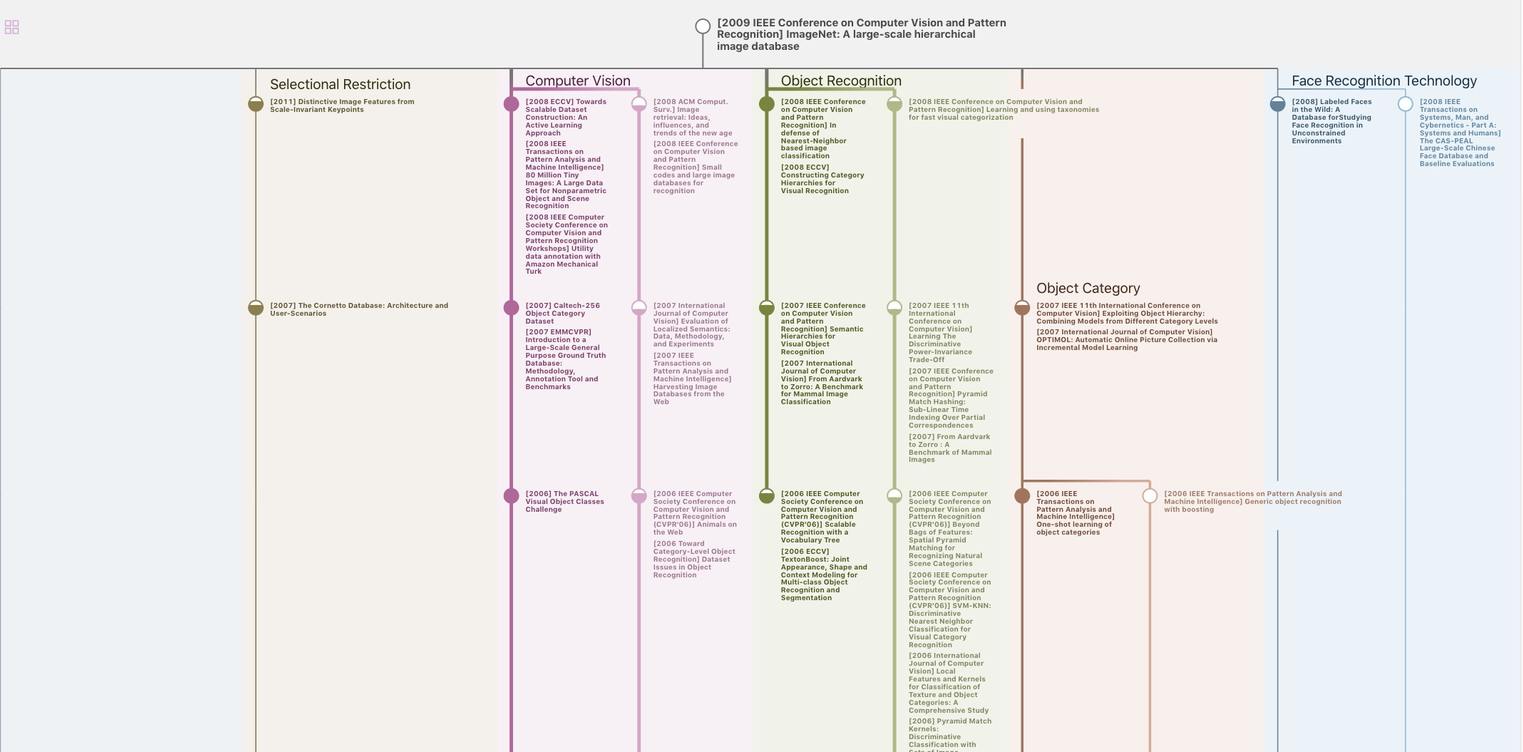
生成溯源树,研究论文发展脉络
Chat Paper
正在生成论文摘要