Adaptive Network Configuration for Efficient and Accurate Neural Video Inference.
IEEE Trans. Cogn. Commun. Netw.(2024)
摘要
Cameras are widely used in many fields, e.g., intelligent transportation, autonomous driving, surveillance, etc. It is thus vital to conduct video analytics in an efficient and accurate manner. However, camera’s built-in capacity is insufficient to support neural network processing, while offloading video streams incurs prohibitive latency and communication cost. In this paper, we find that frame rate, resolution, and neural network inference model, have an intertwined impact on network resource demand. The optimal configuration of these factors also varies with video content feature. To address these challenges, we propose a dynamic configuration update scheme based on predictive video perception using a long short-term memory (LSTM) neural network, to adapt configuration to content changes. This scheme consists of a change detector and a configuration profiler. Through theoretical modeling and analysis, we derive the detection thresholds for both dynamic and stationary video contents, considering the LSTM prediction error. The configuration profiler then updates system by solving an optimization problem, which maximizes the overall utility considering analytics accuracy and resource consumption. Extensive real-world traces-based experiments show that the proposed scheme can save profiling resources by up to 95% while ensuring high accuracy compared with other benchmarks.
更多查看译文
关键词
Video analytics,neural networks,resource allocation,dynamic configuration,distribution estimation,LSTM
AI 理解论文
溯源树
样例
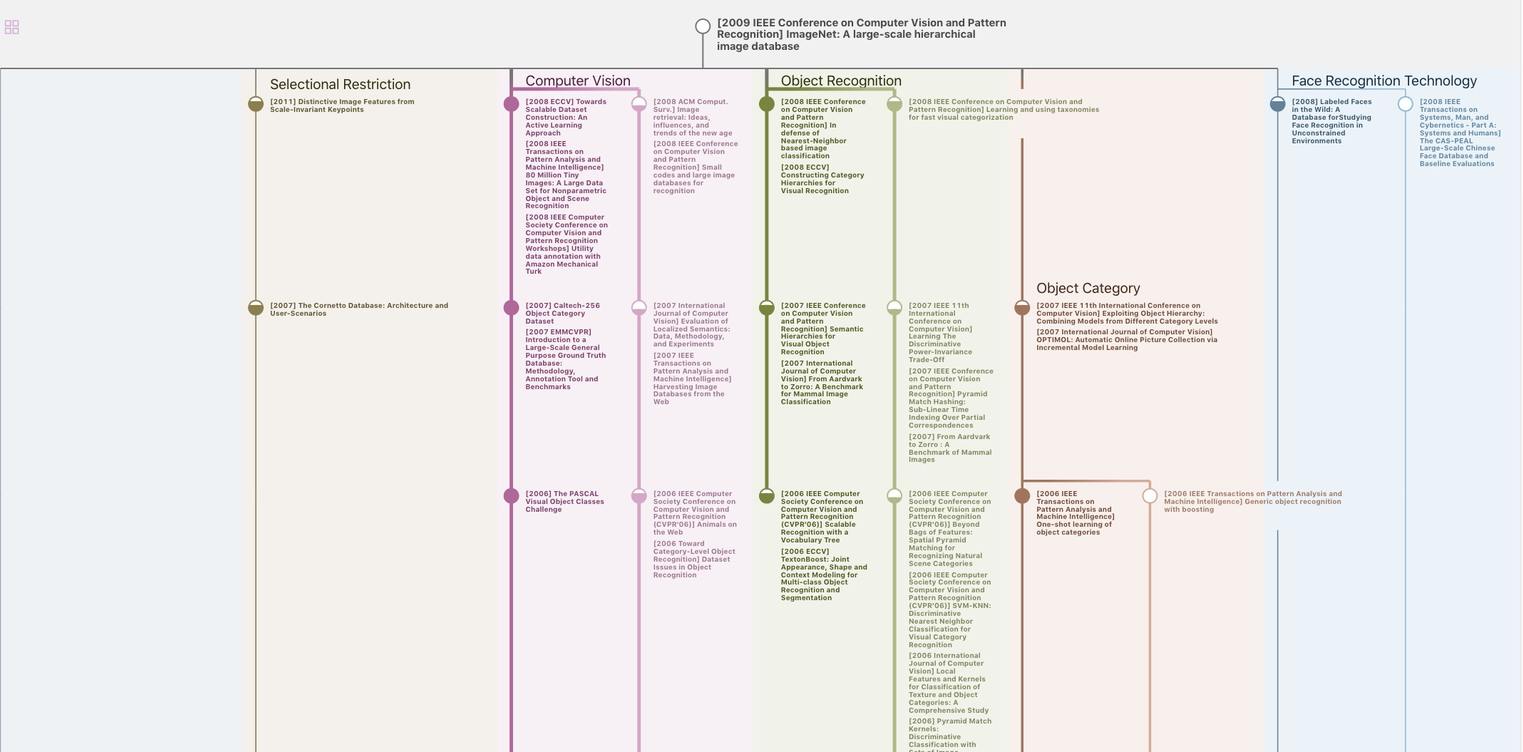
生成溯源树,研究论文发展脉络
Chat Paper
正在生成论文摘要