On the Differential Privacy in Federated Learning based on Over-the-Air Computation
IEEE Transactions on Wireless Communications(2023)
摘要
The federated learning is a promising machine learning technique to bring about advanced services and application for future industries. It has been known that the federated learning secures the privacy of the participants well so far. However, various attacks appear recently which are possible to extract the private information of them in the federated learning systems. Consequently, development of privacy preserving schemes for the federated learning is paramount. In this paper, we consider the over-the-air computation based federated learning system, and adopt the concept of differential privacy to prevent the private information leakage. During the training process, when a sum of local gradients is received via over-the-air computation, they conceal each other and appear to be random to the parameter server. Motivated by this fact, the differential privacy of the over-the-air computation based federated learning is analyzed by considering the inherent randomness of the local gradients. We analytically quantify required amount of the artificial noise to be added to preserve privacy. Furthermore, a parameter estimation based algorithm is proposed which is applicable in real scenarios. The simulation results show the efficacy of the proposed algorithm for preserving privacy.
更多查看译文
关键词
Federated learning,over-the-air computation,differential privacy,central limit theorem
AI 理解论文
溯源树
样例
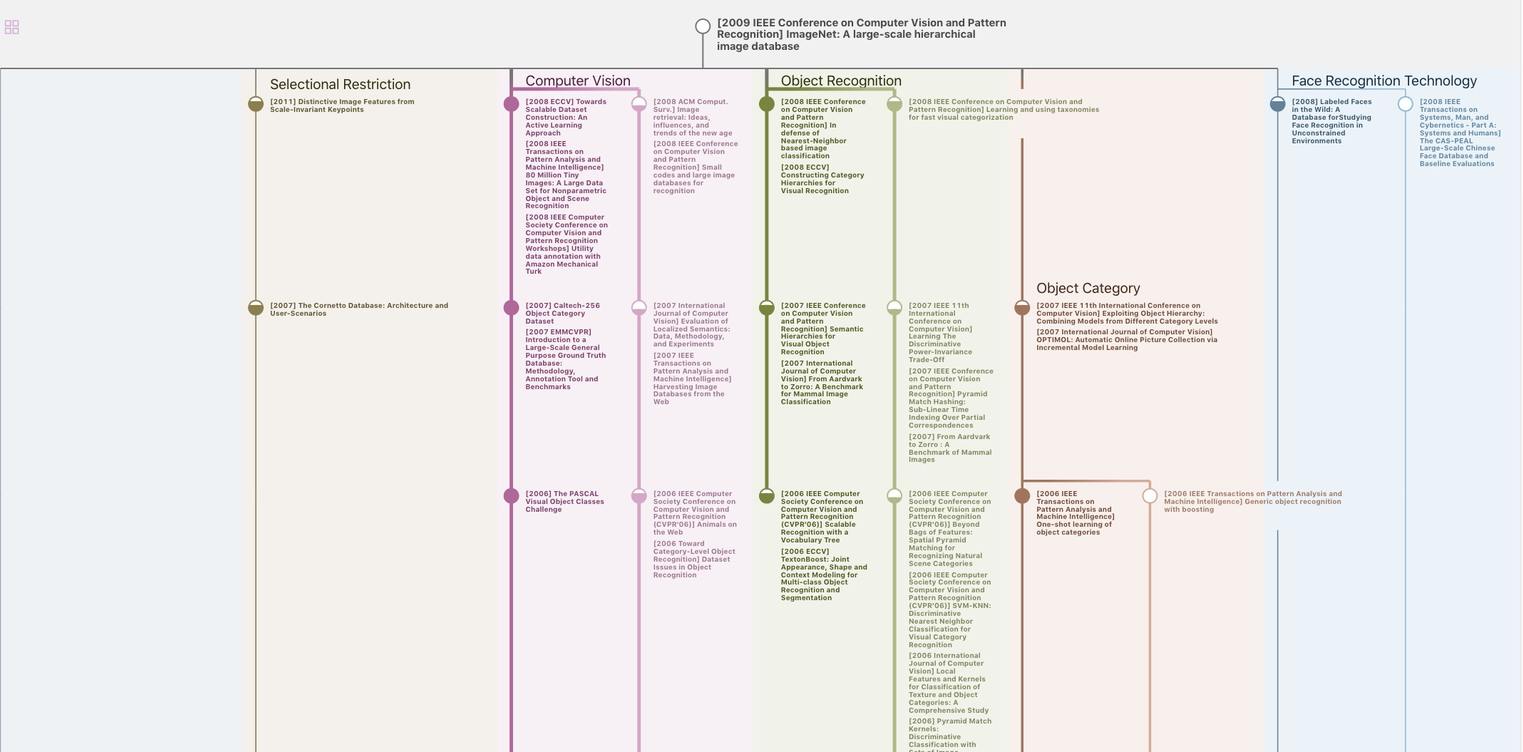
生成溯源树,研究论文发展脉络
Chat Paper
正在生成论文摘要