Finite Volume Graph Network(FVGN): Predicting unsteady incompressible fluid dynamics with finite volume informed neural network
arXiv (Cornell University)(2023)
摘要
In recent years, the development of deep learning is noticeably influencing the progress of computational fluid dynamics. Numerous researchers have undertaken flow field predictions on a variety of grids, such as MAC grids, structured grids, unstructured meshes, and pixel-based grids which have been many works focused on. However, predicting unsteady flow fields on unstructured meshes remains challenging. When employing graph neural networks (GNNs) for these predictions, the message-passing mechanism can become inefficient, especially with denser unstructured meshes. Furthermore, unsteady flow field predictions often rely on autoregressive neural networks, which are susceptible to error accumulation during extended predictions. In this study, we integrate the traditional finite volume method to devise a spatial integration strategy that enables the formulation of a physically constrained loss function. This aims to counter the error accumulation that emerged in autoregressive neural networks during long-term predictions. Concurrently, we merge vertex-center and cell-center grids from the finite volume method, introducing a dual message-passing mechanism within a single GNN layer to enhance the message-passing efficiency. We benchmark our approach against MeshGraphnets for unsteady flow field predictions on unstructured meshes. Our findings indicate that the methodologies combined in this study significantly enhance the precision of flow field predictions while substantially minimizing the training time cost. We offer a comparative analysis of flow field predictions, focusing on cylindrical, airfoil, and square column obstacles in two-dimensional incompressible fluid dynamics scenarios. This analysis encompasses lift coefficient, drag coefficient, and pressure coefficient distribution comparison on the boundary layers.
更多查看译文
关键词
fluid dynamics,neural networkfvgn,finite volume
AI 理解论文
溯源树
样例
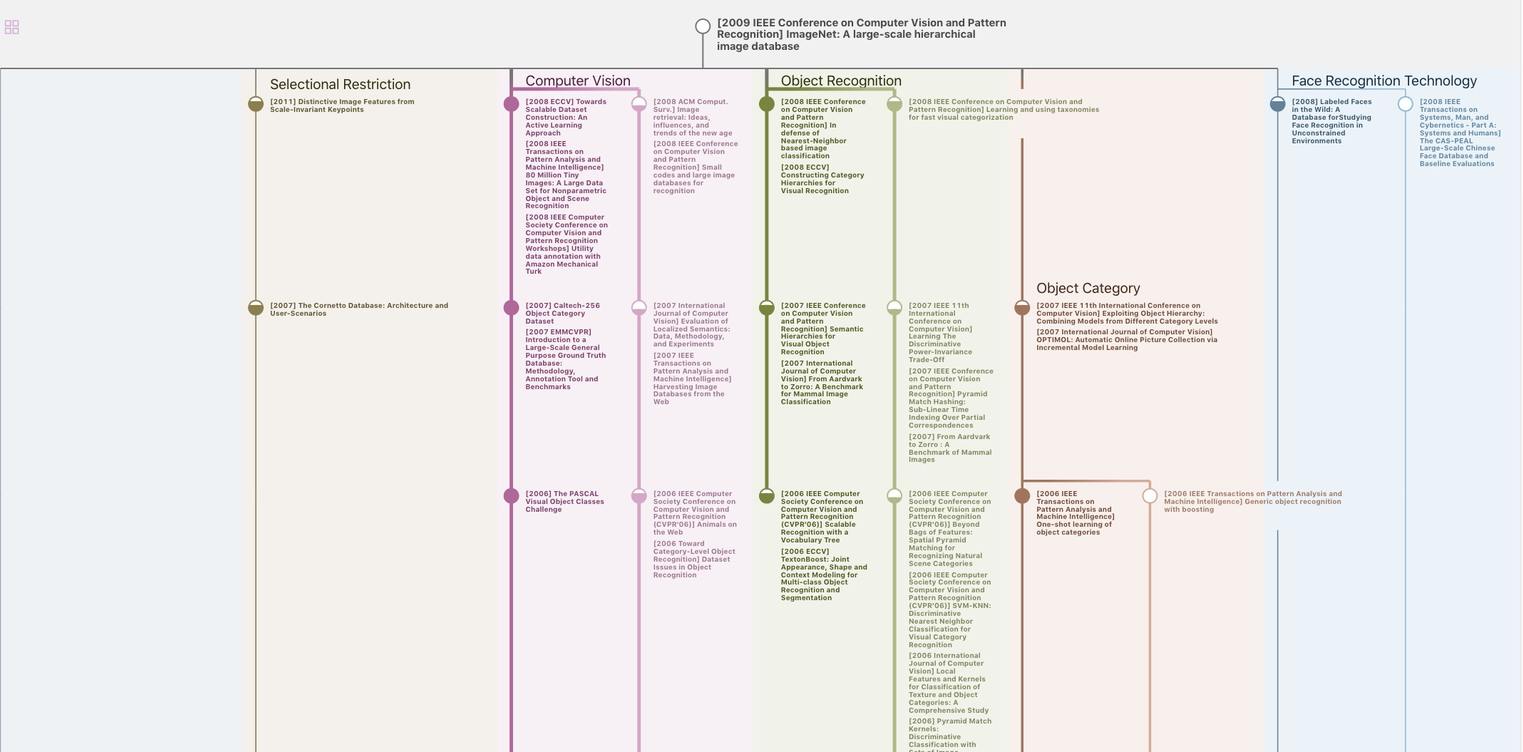
生成溯源树,研究论文发展脉络
Chat Paper
正在生成论文摘要