Salient Object Detection With Dual-Branch Stepwise Feature Fusion and Edge Refinement
IEEE TRANSACTIONS ON CIRCUITS AND SYSTEMS FOR VIDEO TECHNOLOGY(2024)
摘要
In recent years, Transformers have been gradually applied in salient object detection tasks with good results. However, the Transformer's global modeling capabilities can lead to the loss of local details that are important in salient object detection tasks. A feature extraction backbone based on a convolutional neural network (CNN) is good at extracting local detail features due to the gradual expansion of the receptive field but is limited by the size of the receptive field, resulting in an insufficient ability to extract global semantic features. Therefore, this paper combines the Transformer with a CNN and presents a dual-branch encoder to ensure that the features extracted contain rich global semantic information as well as local detail features. In addition, due to the different features extracted by the Transformer and CNN, noise may be introduced in the fusion of the two features, so different features need to be processed correspondingly during fusion. The fusion enhancement module (FEM) we propose fuses the features of the two branches step by step. A hybrid attention mechanism is used to carry out weighted fusion of different features. This progressive approach minimizes the differences between the features of the two branches so that the merged features retain the semantic and detail features extracted by the two branches to the greatest extent. Considering the loss of detailed information caused by repeated downsampling, we propose an edge refinement module (ERM) to address the need for accurate outline prediction. This module leverages salient features to obtain edge features and gradually refines the prediction results by incorporating these edge features. It makes full use of the connection between salient features and edge features and does not introduce additional edges to extract branches. Extensive experimental evaluations conducted on five benchmark tests demonstrate the superior performance of our method compared to other existing approaches. Code can be found at https://github.com/gfq1605694825/DSRNet-main.
更多查看译文
关键词
Feature extraction,Transformers,Task analysis,Semantics,Image edge detection,Convolutional neural networks,Object detection,Salient object detection,dual-branch encoder,attention,gradual feature fusion,edge enhancement
AI 理解论文
溯源树
样例
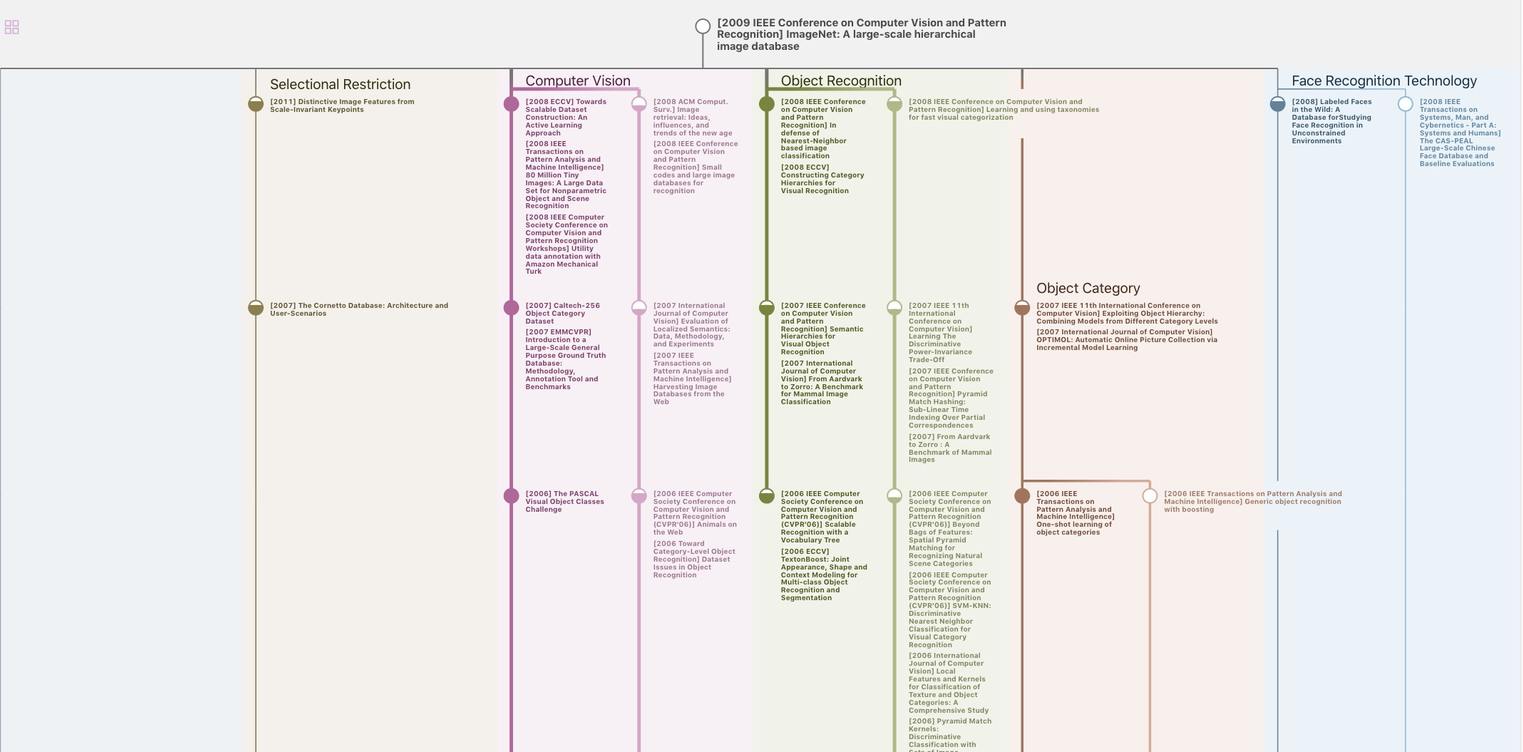
生成溯源树,研究论文发展脉络
Chat Paper
正在生成论文摘要