Advance Prediction of Rockmass Conditions During TBM Tunnelling Based on Cost-Sensitive Learning Under Imbalance Dataset
All Days(2023)
摘要
ABSTRACT The uncertainty of rockmass conditions will be an important factor causing TBM construction risks and inefficient tunnelling. Currently, prediction of rockmass classification based on TBM operation data and machine learning models has been proved feasible by many researchers. However, due to the sample imbalance problem in the previous studies, the prediction accuracy of minority rockmass class is relatively poor. To overcome the above problem, this study proposed a rockmass classification advance prediction method based on cost-sensitive learning (CSL), which using 10 key TBM operation parameters as model inputs. First, through the data preprocessing, the dataset consists of 7538 TBM tunnelling cycles and corresponding rockmass classification information is obtained. And the sample set was divided into training set and test set by stratified sampling. Then, eight classifiers, i.e., support vector machine (SVM), decision tree (DT), random forest (RF), k-nearest neighbors (KNN) and their cost-sensitive version are established to construct the mapping relationship between key TBM operation parameters and rockmass classes. The case study results show that AdaBoost classifier presents the best prediction performance, with the accuracy and F1-score more than 0.9. And CSL can improve the prediction accuracy of minority class samples to some extent to achieve more reasonable prediction results. INTRODUCTION With the continuous improvement of construction mechanization level, tunnel boring machine (TBM) plays an increasingly important role in tunnel construction. Especially for long tunnels, the efficiency and economic advantages of TBM will be more obvious (Liu et al., 2020a; Hou et al., 2022b). TBM is sensitive to the change of rockmass condition, and depends on the empirical adjustment of TBM driver to achieve adaptive tunnelling (Hamidi et al., 2010). However, the geological exploration boreholes before the construction of the long tunnel are relatively sparse, which cannot effectively describe the rockmass conditions of the whole tunnel. Therefore, it is urgent to propose an advanced prediction method of rockmass classification during TBM construction.
更多查看译文
关键词
tbm tunnelling,rockmass conditions,advance prediction,cost-sensitive
AI 理解论文
溯源树
样例
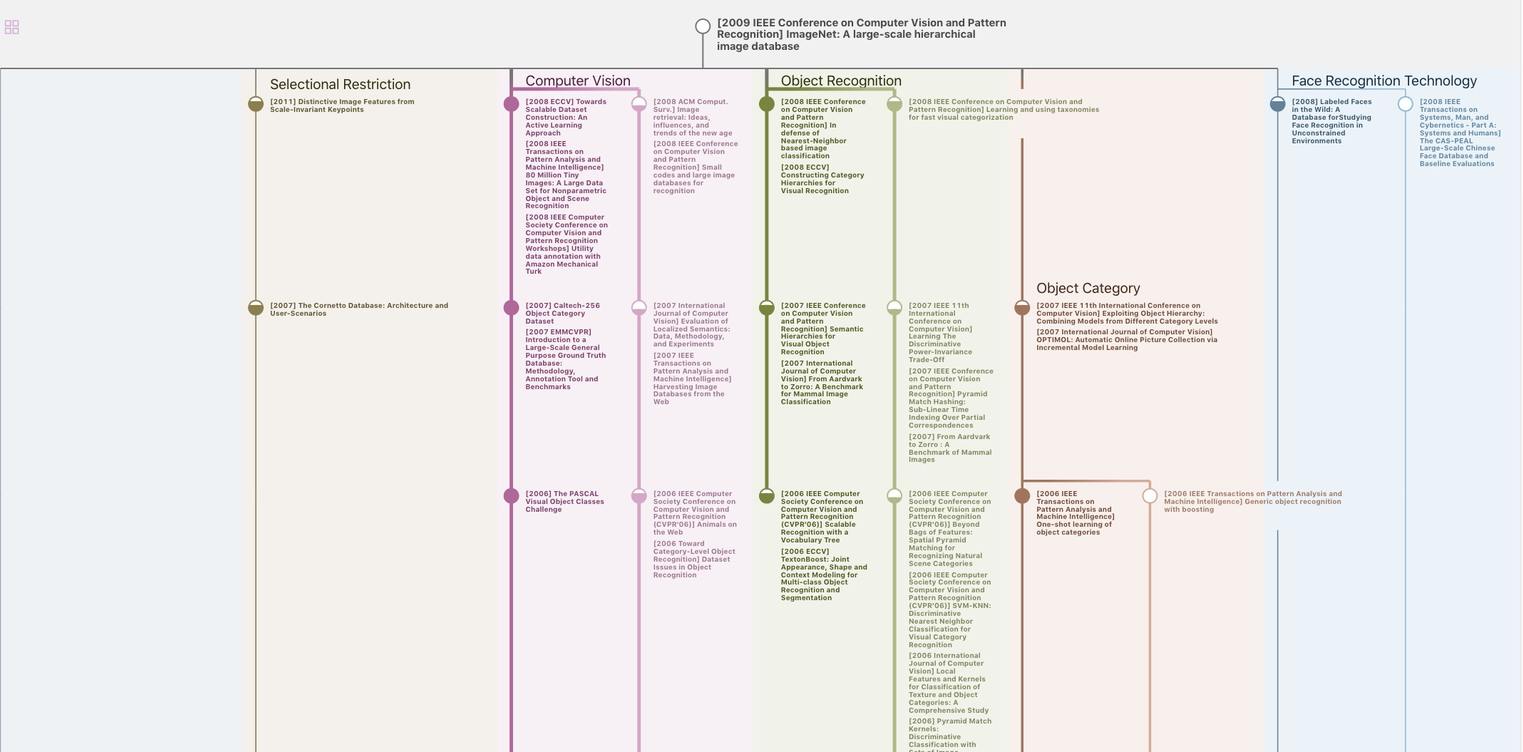
生成溯源树,研究论文发展脉络
Chat Paper
正在生成论文摘要