Overfitting in portfolio optimization
JOURNAL OF RISK MODEL VALIDATION(2023)
摘要
In this paper we measure the out-of-sample performance of sample-based rolling-window neural network (NN) portfolio optimization strategies. We show that if NN strategies are evaluated using the holdout (train-test split) technique, then high out-of-sample performance scores can commonly be achieved. Although this phenomenon is often employed to validate NN portfolio models, we demonstrate that it constitutes a "fata morgana" that arises due to a particular vulnerability of portfolio optimization to overfitting. To assess whether overfitting is present, we set up a dedicated methodology based on combinatorially symmetric cross-validation that involves performance measurement across different holdout periods and varying portfolio compositions (the random-asset-stabilized combinatorially symmetric cross-validation methodology). We compare a variety of NN strategies with classical extensions of the mean-variance model and the 1=N strategy. We find that it is by no means trivial to outperform the classical models. While certain NN strategies outperform the 1=N benchmark, of the almost 30 models that we evaluate explicitly, none is consistently better than the short-sale constrained minimum-variance rule in terms of the Sharpe ratio or the certainty equivalent of returns.
更多查看译文
关键词
portfolio optimization,neural network (NN),deep learning,cross validation,overfitting
AI 理解论文
溯源树
样例
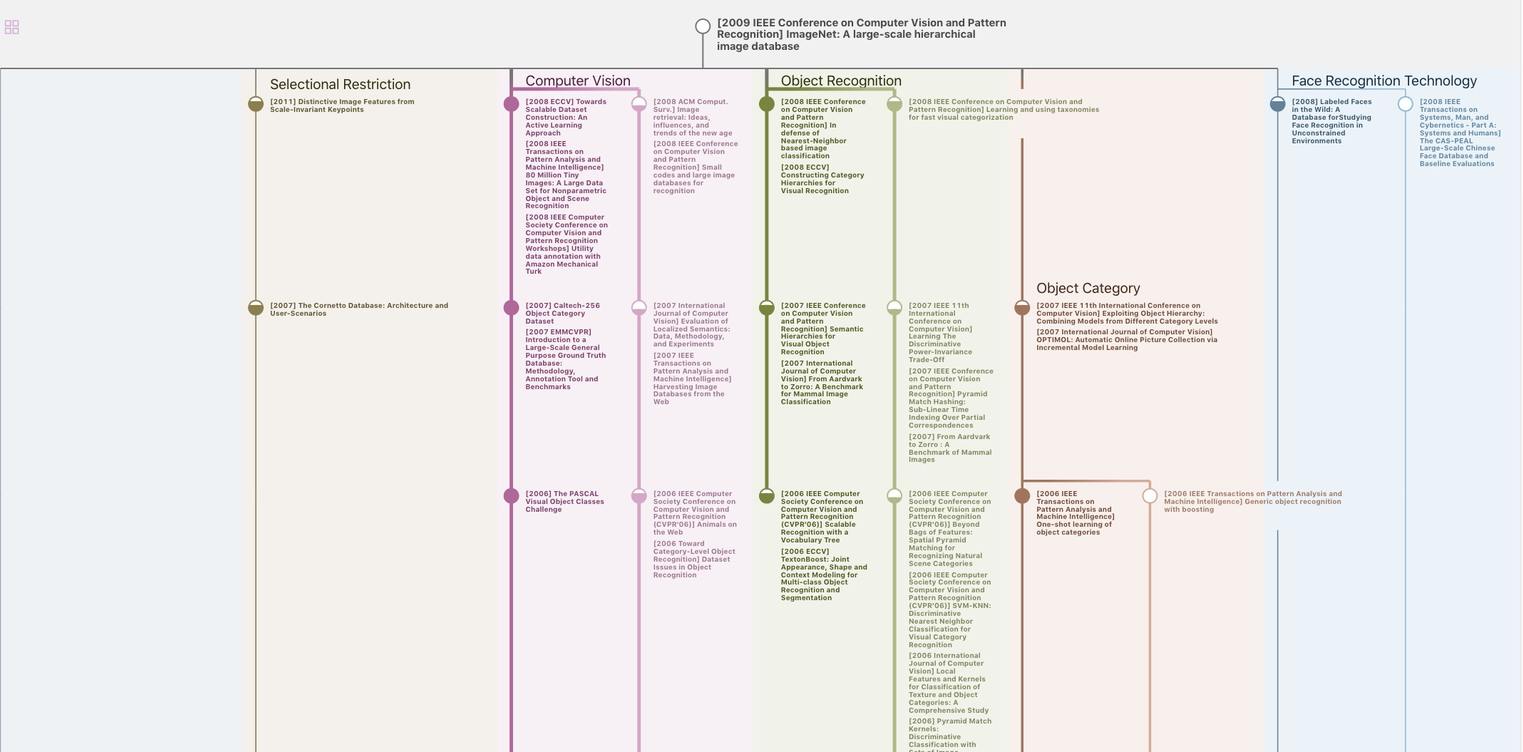
生成溯源树,研究论文发展脉络
Chat Paper
正在生成论文摘要