Machine learning-accelerated discovery of novel 2D ferromagnetic materials with strong magnetization
Research Square (Research Square)(2023)
摘要
Two-dimensional ferromagnetic (2DFM) semiconductors (metals, half-metals, and so on) are important materials for next-generation nano-electronic and nano-spintronic devices. However, these kinds of materials remain scarce, “trial and error” experiments and calculations are both time-consuming and expensive. In the present work, in order to obtain the optimal 2DFM materials with strong magnetization, a machine learning (ML) framework was established to search the 2D material space containing over 2417 samples and identified 615 compounds whose magnetic orders were then determined via high-throughput first-principles calculations. With the adoption of ML algorithms, two classification models and a regression model were trained. The interpretability of the regression model was evaluated through Shapley Additive exPlanations (SHAP) analysis. Unexpectedly, it is found that Cr2NF2 is a potential antiferromagnetic ferroelectric 2D multiferroic material. More importantly, 60 novel 2DFM candidates were predicted, and among them, 13 candidates have magnetic moments of > 7μB. Os2Cl8, Fe3GeSe2, and Mn4N3S2 were predicted to be novel 2DFM semiconductors, metals, and half-metals, respectively. With the adoption of the ML approach in the current work, the prediction of 2DFM materials with strong magnetization can be accelerated, and the computation time can be drastically reduced by more than one order of magnitude.
更多查看译文
关键词
2D ferromagnetic,Machine learning,High through-put screening,DFT,Model interpretability
AI 理解论文
溯源树
样例
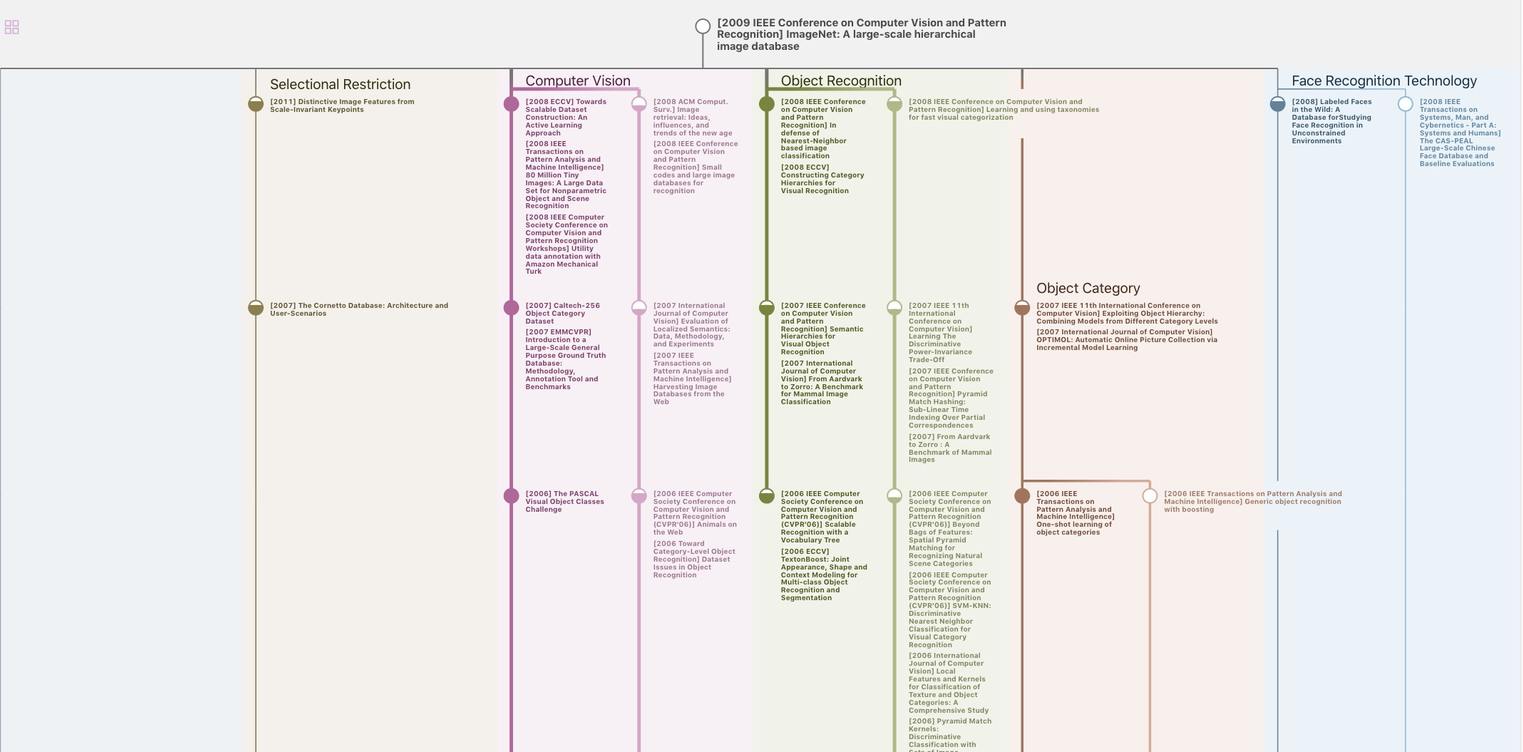
生成溯源树,研究论文发展脉络
Chat Paper
正在生成论文摘要